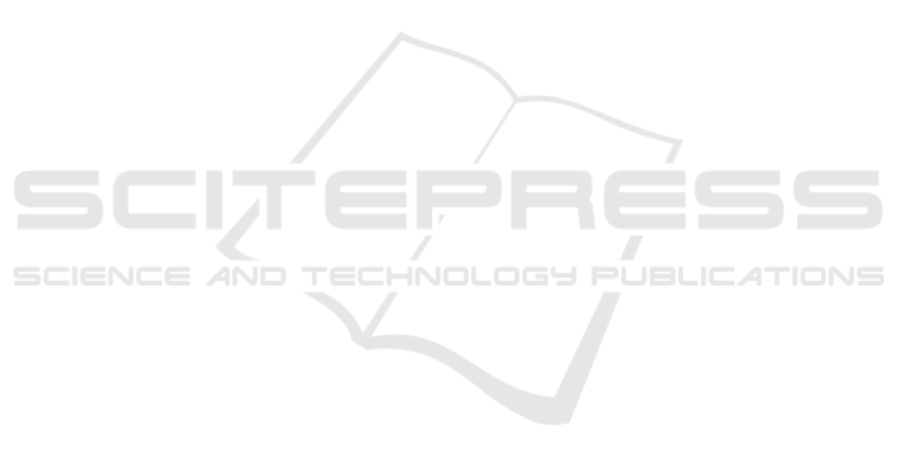
Future work aims to collect and analyse data from
a larger cohort of subjects to further test and validate
our psychometric features. In addition, we aim to use
our validated psychometric features to create a holis-
tic predictive model concordant with the established
allostatic load model(McEwen and Rasgon, 2018),
giving greater focus on chronic stress and its effect
on a person’s overall well-being.
5 CONCLUSIONS
We demonstrated the importance of including psy-
chological data in an acute stress study. Through a
joint analysis of physiological and psychological fea-
tures, we showed that stress classification could be
enhanced. Furthermore, accounting for psychomet-
ric data reduces the number of physiological signal
features needed stress classification. We also found
that our psychometric features could aid in identifying
the type of stress (eustress or distress) an individual
perceives, as indicated by a self-assessment question-
naire’s independent contributions of each mood de-
scriptor (affect). Our work provides an incremental
step towards translating affect linked to stress to suit-
able quantitative measurements similar to those of-
fered by physiological sensors. Joint analysis of psy-
chological and physiological data could be beneficial
towards the detection and management of stress. Fur-
thermore, our work could support the future devel-
opment of holistic stress models consistent with the
well-established allostatic load model. Such models
could be beneficial for workers in harsh environments
like healthcare and personal support workers.
REFERENCES
Asuncion, A. and Newman, D. (2007). Uci machine learn-
ing repository.
Boucsein, W. (2012). Electrodermal activity. Springer Sci-
ence & Business Media.
Campbell, A. A. and Wisco, B. E. (2021). Respiratory si-
nus arrhythmia reactivity in anxiety and posttraumatic
stress disorder: A review of literature. Clinical Psy-
chology Review, page 102034.
Choi, B., Jebelli, H., and Lee, S. (2019). Feasibility analy-
sis of electrodermal activity (eda) acquired from wear-
able sensors to assess construction workers’ perceived
risk. Safety science, 115:110–120.
Choi, J., Ahmed, B., and Gutierrez-Osuna, R. (2011). De-
velopment and evaluation of an ambulatory stress
monitor based on wearable sensors. IEEE trans-
actions on information technology in biomedicine,
16(2):279–286.
Crawford, J. R. and Henry, J. D. (2004). The positive and
negative affect schedule (panas): Construct validity,
measurement properties and normative data in a large
non-clinical sample. British journal of clinical psy-
chology, 43(3):245–265.
de Fazio, R., Cafagna, D., Marcuccio, G., Minerba, A., and
Visconti, P. (2020). A multi-source harvesting system
applied to sensor-based smart garments for monitor-
ing workers’ bio-physical parameters in harsh envi-
ronments. Energies, 13(9):2161.
Edmondson, D., Green, P., Ye, S., Halazun, H. J., and
Davidson, K. W. (2014). Psychological stress and 30-
day all-cause hospital readmission in acute coronary
syndrome patients: an observational cohort study.
PloS one, 9(3):e91477.
Fava, G. A., McEwen, B. S., Guidi, J., Gostoli, S., Offi-
dani, E., and Sonino, N. (2019). Clinical characteri-
zation of allostatic overload. Psychoneuroendocrinol-
ogy, 108:94–101.
George, D. and Mallery, P. (2019). IBM SPSS statistics 26
step by step: A simple guide and reference. Routledge.
Grossman, P. and Taylor, E. W. (2007). Toward understand-
ing respiratory sinus arrhythmia: Relations to cardiac
vagal tone, evolution and biobehavioral functions. Bi-
ological psychology, 74(2):263–285.
Guidi, J., Lucente, M., Sonino, N., and Fava, G. A.
(2021). Allostatic load and its impact on health: a
systematic review. Psychotherapy and psychosomat-
ics, 90(1):11–27.
Healey, J. A. and Picard, R. W. (2005). Detecting stress dur-
ing real-world driving tasks using physiological sen-
sors. IEEE Transactions on intelligent transportation
systems, 6(2):156–166.
Hovsepian, K., Al’Absi, M., Ertin, E., Kamarck, T., Naka-
jima, M., and Kumar, S. (2015). cstress: towards a
gold standard for continuous stress assessment in the
mobile environment. In Proceedings of the 2015 ACM
international joint conference on pervasive and ubiq-
uitous computing, pages 493–504.
Karantonis, D. M., Narayanan, M. R., Mathie, M., Lovell,
N. H., and Celler, B. G. (2006). Implementa-
tion of a real-time human movement classifier us-
ing a triaxial accelerometer for ambulatory monitor-
ing. IEEE transactions on information technology in
biomedicine, 10(1):156–167.
Kreibig, S. D. (2010). Autonomic nervous system activ-
ity in emotion: A review. Biological psychology,
84(3):394–421.
Lai, K., Yanushkevich, S. N., and Shmerko, V. P. (2021).
Intelligent stress monitoring assistant for first respon-
ders. IEEE Access, 9:25314–25329.
Laurent, J., Catanzaro, S. J., Joiner Jr, T. E., Rudolph, K. D.,
Potter, K. I., Lambert, S., Osborne, L., and Gathright,
T. (1999). A measure of positive and negative affect
for children: Scale development and preliminary vali-
dation. Psychological assessment, 11(3):326.
Le Fevre, M., Matheny, J., and Kolt, G. S. (2003). Eu-
stress, distress, and interpretation in occupational
stress. Journal of managerial psychology.
HEALTHINF 2022 - 15th International Conference on Health Informatics
436