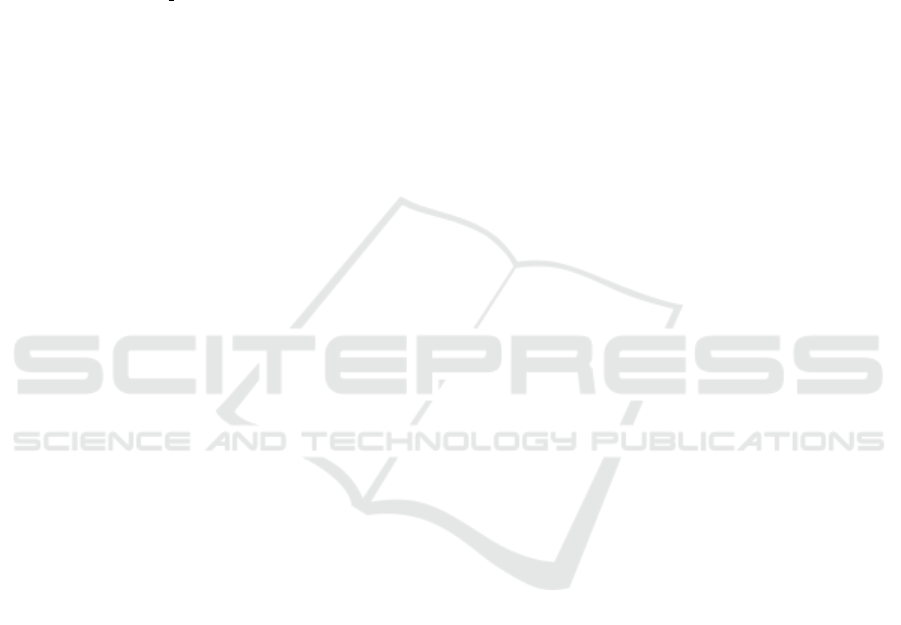
Finally, we plan to improve the classification per-
formances by refining some clue parameters in our
proposed method. We specifically refer to the down-
sampling resolution, the Neural Network structure,
and the length of the rhythm pattern.
ACKNOWLEDGMENT
The authors have been supported by the project PON
2014-2020—ARS01 00860 “ATTICUS: Ambient-
intelligent Tele-monitoring and Telemetry for
Incepting and Catering over hUman Sustainability”
funded by the Ministry of Education, University and
Research—RNA/COR 576347.
REFERENCES
Balestrieri, E., Boldi, F., Colavita, A. R., De Vito, L.,
Laudato, G., Oliveto, R., Picariello, F., Rivaldi, S.,
Scalabrino, S., Torchitti, P., et al. (2019). The ar-
chitecture of an innovative smart t-shirt based on the
internet of medical things paradigm. In 2019 IEEE
International Symposium on Medical Measurements
and Applications (MeMeA), pages 1–6. IEEE.
D. Limmer, M. O’Keefe, H. G. B. M. D. B. (2005). Emer-
gency Care Workbook. Pearson.
De Vito, L., Picariello, E., Picariello, F., Tudosa, I., Lo-
previte, L., Avicolli, D., Laudato, G., and Oliveto, R.
(2021). An undershirt for monitoring of multi-lead
ecg and respiration wave signals. In 2021 IEEE Inter-
national Workshop on Metrology for Industry 4.0 &
IoT (MetroInd4. 0&IoT), pages 550–555. IEEE.
Goldberger, A. L., Amaral, L. A., Glass, L., Hausdorff,
J. M., Ivanov, P. C., Mark, R. G., Mietus, J. E., Moody,
G. B., Peng, C.-K., and Stanley, H. E. (2000). Phys-
iobank, physiotoolkit, and physionet: components of
a new research resource for complex physiologic sig-
nals. circulation, 101(23):e215–e220.
Hochreiter, S. and Schmidhuber, J. (1997). Long short-term
memory. Neural computation, 9(8):1735–1780.
Islam, S., Ammour, N., and Alajlan, N. (2017). Atrial fib-
rillation detection with multiparametric rr interval fea-
ture and machine learning technique. In 2017 Interna-
tional Conference on Informatics, Health & Technol-
ogy (ICIHT), pages 1–5. IEEE.
Karim, F., Majumdar, S., Darabi, H., and Chen, S. (2017).
Lstm fully convolutional networks for time series clas-
sification. IEEE access, 6:1662–1669.
Kurzweil, R. C., Gibson, L., Albrecht, P., and Grimshaw,
P. (2009). Atrial fibrillation detection. US Patent
7,596,405.
Laudato, G., Boldi, F., Colavita, A. R., Rosa, G., Scal-
abrino, S., Lazich, A., and Oliveto, R. (2020a). Com-
bining rhythmic and morphological ecg features for
automatic detection of atrial fibrillation: Local and
global prediction models. In International Joint Con-
ference on Biomedical Engineering Systems and Tech-
nologies, pages 425–441. Springer.
Laudato, G., Boldi, F., Colavita, A. R., Rosa, G., Scal-
abrino, S., Torchitti, P., Lazich, A., and Oliveto, R.
(2020b). Combining rhythmic and morphological ecg
features for automatic detection of atrial fibrillation.
In HEALTHINF, pages 156–165.
Laudato, G., Scalabrino, S., Colavita, A., Chiacchiari, Q.,
D’Orazio, R., Donadelli, R., De Vito, L., Picariello, F.,
Tudosa, I., Malatesta, R., Gallo, L., and R, O. (2021).
ATTICUS: Ambient-intelligent Tele-monitoring and
Telemetry for Incepting and Catering over hUman
Sustainability. Frontiers in Human Dynamics - Dig-
ital Impacts (Frontiers).
Padmavathi, K. and Ramakrishna, K. S. (2015). Classifi-
cation of ecg signal during atrial fibrillation using au-
toregressive modeling. Procedia Computer Science,
46:53–59.
Pan, J. and Tompkins, W. J. (1985). A real-time qrs de-
tection algorithm. IEEE transactions on biomedical
engineering, (3):230–236.
Sepulveda-Suescun, J., Murillo-Escobar, J., Urda-Benitez,
R., Orrego-Metaute, D., and Orozco-Duque, A.
(2017). Atrial fibrillation detection through heart
rate variability using a machine learning approach
and poincare plot features. In VII Latin American
Congress on Biomedical Engineering CLAIB 2016,
Bucaramanga, Santander, Colombia, October 26th-
28th, 2016, pages 565–568. Springer.
Xiong, Z., Stiles, M. K., and Zhao, J. (2017). Robust ecg
signal classification for detection of atrial fibrillation
using a novel neural network. In 2017 Computing in
Cardiology (CinC), pages 1–4. IEEE.
Xu, S. S., Mak, M.-W., and Cheung, C.-C. (2018). To-
wards end-to-end ecg classification with raw signal
extraction and deep neural networks. IEEE journal of
biomedical and health informatics, 23(4):1574–1584.
Yuan, C., Yan, Y., Zhou, L., Bai, J., and Wang, L. (2016).
Automated atrial fibrillation detection based on deep
learning network. In 2016 IEEE International Con-
ference on Information and Automation (ICIA), pages
1159–1164. IEEE.
Zhou, X., Ding, H., Wu, W., and Zhang, Y. (2015). A real-
time atrial fibrillation detection algorithm based on the
instantaneous state of heart rate. PloS one, 10(9).
Zurro, V., Stelle, A., and Nadal, J. (1995). Detection of
atrial persistent rhythm based on p-wave recognition
and rr interval variability. In Computers in Cardiology
1995, pages 185–188. IEEE.
Simulating the Doctor’s Behaviour: A Preliminary Study on the Identification of Atrial Fibrillation through Combined Analysis of Heart
Rate and Beat Morphology
453