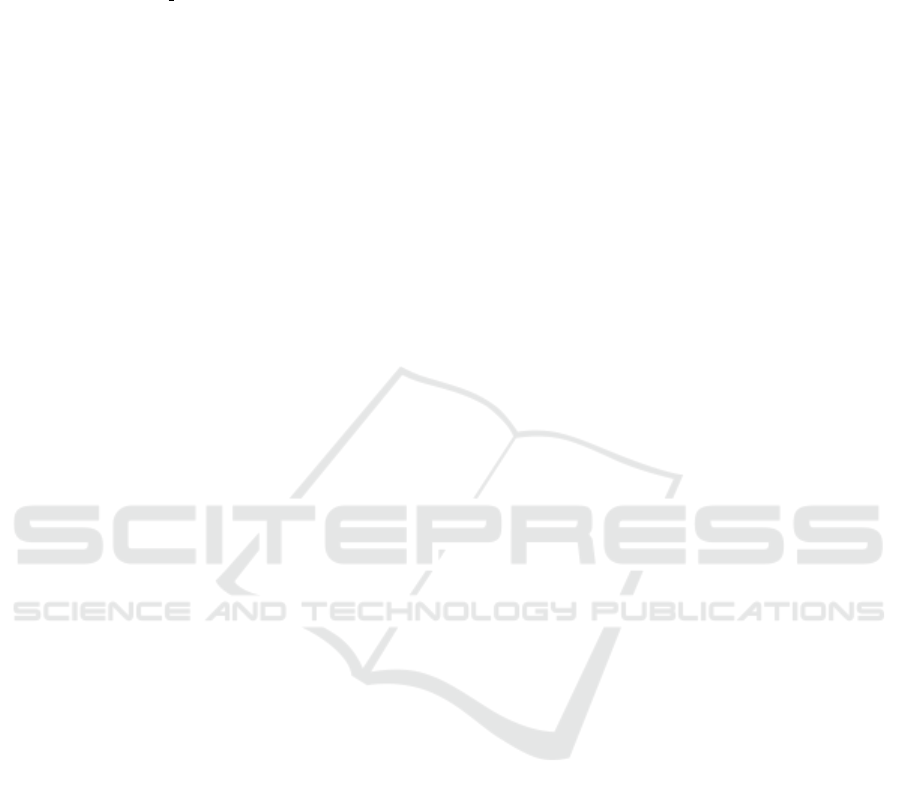
ACKNOWLEDGMENT
The authors have been supported by the project PON
2014-2020—ARS01 00860 “ATTICUS: Ambient-
intelligent Tele-monitoring and Telemetry for
Incepting and Catering over hUman Sustainability”
funded by the Ministry of Education, University and
Research—RNA/COR 576347.
REFERENCES
Balas, E. A., Austin, S. M., Mitchell, J. A., Ewigman, B. G.,
Bopp, K. D., and Brown, G. D. (1996). The clinical
value of computerized information services. a review
of 98 randomized clinical trials. Archives of Family
Medicine, 5(5):271–278.
Balestrieri, E., Boldi, F., Colavita, A. R., De Vito, L.,
Laudato, G., Oliveto, R., Picariello, F., Rivaldi, S.,
Scalabrino, S., Torchitti, P., et al. (2019). The ar-
chitecture of an innovative smart t-shirt based on the
internet of medical things paradigm. In 2019 IEEE
International Symposium on Medical Measurements
and Applications (MeMeA), pages 1–6. IEEE.
Berner, E. S. (2007). Clinical decision support systems,
volume 233. Springer.
Chawla, N. V., Bowyer, K. W., Hall, L. O., and Kegelmeyer,
W. P. (2002). Smote: synthetic minority over-
sampling technique. Journal of artificial intelligence
research, 16:321–357.
De Vito, L., Picariello, E., Picariello, F., Tudosa, I., Lo-
previte, L., Avicolli, D., Laudato, G., and Oliveto, R.
(2021). An undershirt for monitoring of multi-lead
ecg and respiration wave signals. In 2021 IEEE Inter-
national Workshop on Metrology for Industry 4.0 &
IoT (MetroInd4. 0&IoT), pages 550–555. IEEE.
Ghaemi, A., Rezaie-Balf, M., Adamowski, J., Kisi, O.,
and Quilty, J. (2019). On the applicability of max-
imum overlap discrete wavelet transform integrated
with mars and m5 model tree for monthly pan evap-
oration prediction. Agricultural and Forest Meteorol-
ogy, 278:107647.
Goldberger, A. L., Amaral, L. A., Glass, L., Hausdorff,
J. M., Ivanov, P. C., Mark, R. G., Mietus, J. E., Moody,
G. B., Peng, C.-K., and Stanley, H. E. (2000). Phys-
iobank, physiotoolkit, and physionet: components of
a new research resource for complex physiologic sig-
nals. circulation, 101(23):e215–e220.
Hadjem, M., Na
¨
ıt-Abdesselam, F., and Khokhar, A. (2016).
St-segment and t-wave anomalies prediction in an ecg
data using rusboost. In 2016 IEEE 18th Interna-
tional Conference on e-Health Networking, Applica-
tions and Services (Healthcom), pages 1–6. IEEE.
Harun-Ar-Rashid, M., Mahmud, G., Rahman, M. M., and
Hossain, A. D. (2020). Classification of st segment in
ecg signals based on cross correlated supervised data.
SN Applied Sciences, 2(7):1–18.
Huang, C., Ye, S., Chen, H., Li, D., He, F., and
Tu, Y. (2010). A novel method for detection of
the transition between atrial fibrillation and sinus
rhythm. IEEE Transactions on Biomedical Engineer-
ing, 58(4):1113–1119.
Jeong, G.-Y. and Yu, K.-H. (2006). Design of ambula-
tory ecg monitoring system to detect st pattern change.
In 2006 SICE-ICASE International Joint Conference,
pages 5873–5877. IEEE.
Kawamoto, K., Houlihan, C. A., Balas, E. A., and Lobach,
D. F. (2005). Information in practice. bmj, 330:765–
768.
Khan, M. A., Hashim, M. J., Mustafa, H., Baniyas, M. Y.,
Al Suwaidi, S. K. B. M., AlKatheeri, R., Alblooshi, F.
M. K., Almatrooshi, M. E. A. H., Alzaabi, M. E. H.,
Al Darmaki, R. S., et al. (2020). Global epidemiology
of ischemic heart disease: Results from the global bur-
den of disease study. Cureus, 12(7).
Lake, D. E. and Moorman, J. R. (2011). Accurate esti-
mation of entropy in very short physiological time
series: the problem of atrial fibrillation detection
in implanted ventricular devices. American Jour-
nal of Physiology-Heart and Circulatory Physiology,
300(1):H319–H325.
Laudato, G., Scalabrino, S., Colavita, A., Chiacchiari, Q.,
D’Orazio, R., Donadelli, R., De Vito, L., Picariello, F.,
Tudosa, I., Malatesta, R., Gallo, L., and R, O. (2021).
ATTICUS: Ambient-intelligent Tele-monitoring and
Telemetry for Incepting and Catering over hUman
Sustainability. Frontiers in Human Dynamics - Dig-
ital Impacts (Frontiers).
Leonarduzzi, R. F., Schlotthauer, G., and Torres, M. E.
(2010). Wavelet leader based multifractal analysis
of heart rate variability during myocardial ischaemia.
In 2010 Annual International Conference of the IEEE
Engineering in Medicine and Biology, pages 110–113.
IEEE.
Maglaveras, N., Stamkopoulos, T., Pappas, C., and
Strintzis, M. G. (1998). An adaptive backpropaga-
tion neural network for real-time ischemia episodes
detection: development and performance analysis us-
ing the european st-t database. IEEE Transactions on
Biomedical Engineering, 45(7):805–813.
Pan, J. and Tompkins, W. J. (1985). A real-time qrs de-
tection algorithm. IEEE transactions on biomedical
engineering, (3):230–236.
Rosa, G., Laudato, G., Colavita, A. R., Scalabrino, S., and
Oliveto, R. (2021a). Automatic real-time beat-to-beat
detection of arrhythmia conditions. In HEALTHINF,
pages 212–222.
Rosa, G., Russodivito, M., Laudato, G., Scalabrino, S.,
Colavita, A., and Oliveto, R. (2-4 October 2021b).
A multi-class approach for the automatic detection of
congestive heart failure in windowed ecg. In MED-
INFO ’21). Online Streaming.
Sintchenko, V., Coiera, E., Iredell, J. R., and Gilbert, G. L.
(2004). Comparative impact of guidelines, clinical
data, and decision support on prescribing decisions:
an interactive web experiment with simulated cases.
Journal of the American Medical Informatics Associ-
ation, 11(1):71–77.
Sol
´
e, X., Ramisa, A., and Torras, C. (2014). Evaluation of
random forests on large-scale classification problems
A Robust Approach for a Real-time Accurate Screening of ST Segment Anomalies
79