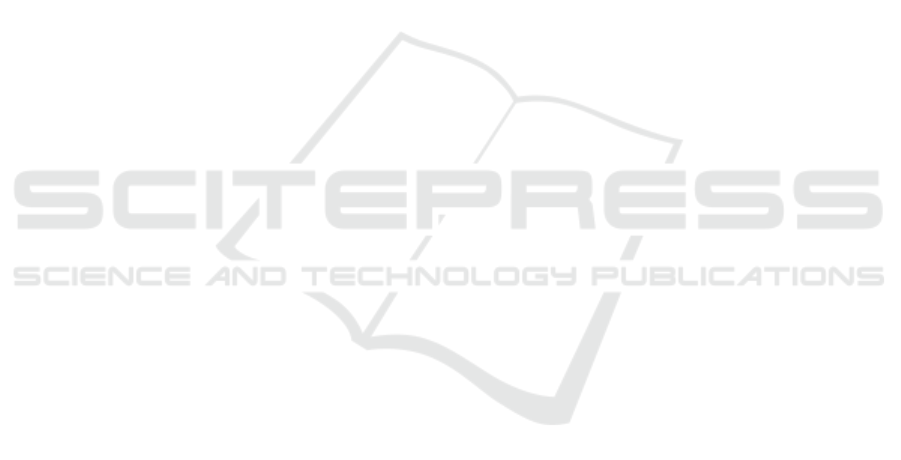
sometimes originate in the detected positional coor-
dinates closing aboard the BB. Besides, unannotated
high-intensity regions subjectively similar to an ICH
cause FP detections (Fig. 2 F).
Despite the possibility of false results, the detec-
tion ability denotes the high potential of the algo-
rithm to minimize the probability of missing an ICH
by oversight in clinical practice. Incorporation of the
method in a computer-aided diagnostic system might
warn a radiologist by highlighting the possible loca-
tions of ICHs while examining axial slices of a CT
scan. Besides, the algorithm might significantly de-
crease the examination time as the processing of a
slice takes only a few seconds. Considering the afore-
mentioned arguments, the clinical use of the algo-
rithm could help to prevent permanent disability or
even death.
4 CONCLUSIONS
This paper demonstrates a fully automated, weakly
supervised method for the localization of ICHs in ax-
ial head CT slices. The proposed algorithm is based
on local extrema detection in attention maps that are
obtained by a deep learning model and represent the
likelihood of ICH’s presence in the given slice. The
main advantage of the algorithm is the proposed MIL
position-free learning method, which is used for the
attention map generation. Our approach showed the
ability to localize ICHs using only slice-level annota-
tions. The Dice coefficient of 58.08 % was achieved
on data from publicly available dataset.
ACKNOWLEDGEMENTS
Computational resources were supplied by the project
"e-Infrastruktura CZ" (e-INFRA LM2018140) pro-
vided within the program Projects of Large Research,
Development and Innovation Infrastructures.
REFERENCES
Arbabshirani, M. R., Fornwalt, B. K., Mongelluzzo, G. J.,
Suever, J. D., Geise, B. D., Patel, A. A., and Moore,
G. J. (2018). Advanced machine learning in action:
identification of intracranial hemorrhage on computed
tomography scans of the head with clinical workflow
integration. NPJ digital medicine, 1(1):1–7.
Caceres, J. A. and Goldstein, J. N. (2012). Intracranial hem-
orrhage. Emergency Medicine Clinics of North Amer-
ica, 30(3):771–794.
Chang, P. D., Kuoy, E., Grinband, J., Weinberg, B. D.,
Thompson, M., Homo, R., Chen, J., Abcede, H.,
Shafie, M., Sugrue, L., et al. (2018). Hybrid 3d/2d
convolutional neural network for hemorrhage evalua-
tion on head ct. American Journal of Neuroradiology,
39(9):1609–1616.
Chilamkurthy, S., Ghosh, R., Tanamala, S., Biviji, M.,
Campeau, N. G., Venugopal, V. K., Mahajan, V., Rao,
P., and Warier, P. (2018). Development and validation
of deep learning algorithms for detection of critical
findings in head CT scans. CoRR, abs/1803.05854.
Cho, J., Park, K.-S., Karki, M., Lee, E., Ko, S., Kim,
J. K., Lee, D., Choe, J., Son, J., Kim, M., et al.
(2019). Improving sensitivity on identification and de-
lineation of intracranial hemorrhage lesion using cas-
caded deep learning models. Journal of digital imag-
ing, 32(3):450–461.
Flanders, A. E., Prevedello, L. M., Shih, G., Halabi, S. S.,
Kalpathy-Cramer, J., Ball, R., Mongan, J. T., Stein,
A., Kitamura, F. C., Lungren, M. P., Choudhary,
G., Cala, L., Coelho, L., Mogensen, M., Morón, F.,
Miller, E., Ikuta, I., Zohrabian, V., McDonnell, O.,
Lincoln, C., Shah, L., Joyner, D., Agarwal, A., Lee,
R. K., and Nath, J. (2020). Construction of a ma-
chine learning dataset through collaboration. Radiol-
ogy: Artificial Intelligence, 2(3).
Goldberger, A. L., Amaral, L. A. N., Glass, L., Haus-
dorff, J. M., Ivanov, P. C., Mark, R. G., Mietus,
J. E., Moody, G. B., Peng, C.-K., and Stanley,
H. E. (2000). PhysioBank, PhysioToolkit, and
PhysioNet: Components of a new research resource
for complex physiologic signals. Circulation,
101(23):e215–e220. Circulation Electronic Pages:
http://circ.ahajournals.org/content/101/23/e215.full
PMID:1085218; doi: 10.1161/01.CIR.101.23.e215.
He, K., Zhang, X., Ren, S., and Sun, J. (2016). Deep resid-
ual learning for image recognition. In 2016 IEEE Con-
ference on Computer Vision and Pattern Recognition
(CVPR), pages 770–778. IEEE.
Ilse, M., Tomczak, J., and Welling, M. (2018). Attention-
based deep multiple instance learning. In Dy, J. and
Krause, A., editors, Proceedings of the 35th Interna-
tional Conference on Machine Learning, volume 80
of Proceedings of Machine Learning Research, pages
2127–2136. PMLR.
Ker, J., Singh, S. P., Bai, Y., Rao, J., Lim, T., and Wang, L.
(2019). Image thresholding improves 3-dimensional
convolutional neural network diagnosis of different
acute brain hemorrhages on computed tomography
scans. Sensors, 19(9):2167.
Kingma, D. P. and Ba, J. (2014). Adam: A
method for stochastic optimization. arXiv preprint
arXiv:1412.6980.
Koenderink, J. J. and van Doorn, A. J. (1992). Surface shape
and curvature scales. Image and Vision Computing,
10(8):557–564.
Lee, H., Yune, S., Mansouri, M., Kim, M., Tajmir, S. H.,
Guerrier, C. E., Ebert, S. A., Pomerantz, S. R.,
Romero, J. M., Kamalian, S., et al. (2019). An ex-
plainable deep-learning algorithm for the detection of
Weakly Supervised Deep Learning-based Intracranial Hemorrhage Localization
115