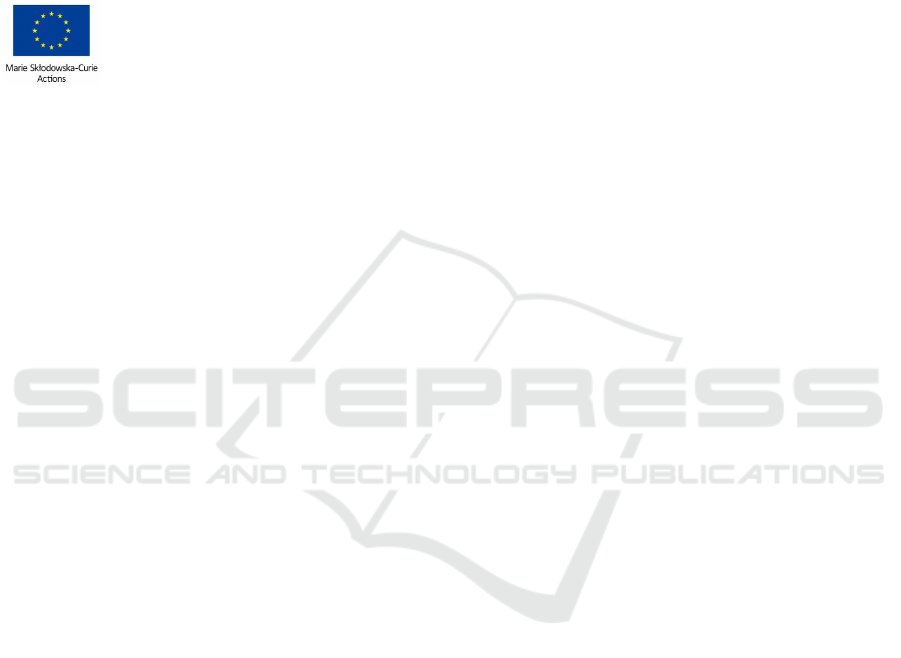
great variety of analysis aspects that may arise dur-
ing the comparison and selection of neural networks
models on 3D part segmentation on point cloud ob-
jects. As future work, we plan to enhance the point
cloud renderings.
ACKNOWLEDGEMENTS
This project has received funding from
the European Union’s Horizon 2020 re-
search and innovation programme under
the Marie Skłodowska-Curie grant agree-
ment No 860843.
REFERENCES
Bello, S. A., Yu, S., Wang, C., Adam, J. M., and Li, J.
(2020). Review: Deep Learning on 3D Point Clouds.
Remote Sensing, 12(11):1729.
Butkus, L. and Luko
ˇ
sevi
ˇ
cius, M. (2018). A Polite Robot:
Visual Handshake Recognition Using Deep Learning.
In CEUR-WS, pages 78–83.
Chang, A. X., Funkhouser, T., Guibas, L., Hanrahan, P.,
Huang, Q., Li, Z., Savarese, S., Savva, M., Song,
S., Su, H., Xiao, J., Yi, L., and Yu, F. (2015).
ShapeNet: An Information-Rich 3D Model Reposi-
tory. arXiv:1512.03012.
Chatzimparmpas, A., Martins, R. M., Jusufi, I., Kucher, K.,
Rossi, F., and Kerren, A. (2020). The State of the Art
in Enhancing Trust in Machine Learning Models with
the Use of Visualizations. Computer Graphics Forum,
39(3):713–756.
Dargan, S., Kumar, M., Ayyagari, M. R., and Kumar, G.
(2019). A Survey of Deep Learning and Its Ap-
plications: A New Paradigm to Machine Learning.
Archives of Computational Methods in Engineering
2019 27:4, 27(4):1071–1092.
Escalera, S., Puig, A., Amoros, O., and Salam
´
o, M. (2011).
Intelligent GPGPU Classification in Volume Visual-
ization: A framework based on Error-Correcting Out-
put Codes. Computer Graphics Forum, 30(7):2107–
2115.
Faulkner, A. and Bhandarkar, S. (2003). An interactive
tool for segmentation, visualization, and navigation of
magnetic resonance images. Proc. of the IEEE Sym-
posium on Computer-Based Medical Systems, pages
340–345.
Friendly, M. (2008). A Brief History of Data Visualization.
Handbook of Data Visualization, pages 15–56.
Garcia-Garcia, A., Orts-Escolano, S., Oprea, S., Villena-
Martinez, V., Martinez-Gonzalez, P., and Garcia-
Rodriguez, J. (2018). A survey on deep learning tech-
niques for image and video semantic segmentation.
Applied Soft Computing, 70:41–65.
Hanhan, I. and Sangid, M. D. (2019). ModLayer: A MAT-
LAB GUI Drawing Segmentation Tool for Visualizing
and Classifying 3D Data. Integrating Materials and
Manufacturing Innovation, 1:3.
Liu, Y., Fan, B., Xiang, S., and Pan, C. (2019). Relation-
shape convolutional neural network for point cloud
analysis. In Proc. of the IEEE CVPR, volume 2019-
June, pages 8887–8896. IEEE Computer Society.
Liu, Z., Hu, H., Cao, Y., Zhang, Z., and Tong, X. (2020). A
Closer Look at Local Aggregation Operators in Point
Cloud Analysis. In LNCS, volume 12368, pages 326–
342.
Nguyen, A. and Le, B. (2013). 3D point cloud segmenta-
tion: A survey. In 2013 6th IEEE Conf. on Robotics,
Automation and Mechatronics (RAM), pages 225–
230. IEEE.
Nystr
¨
om, I., Malmberg, F., Vidholm, E., and Bengtsson, E.
(2009). Segmentation and Visualization of 3D Med-
ical Images through Haptic Rendering. In Pattern
Recognition and Information Processing.
Pappas, L. and Whitman, L. (2011). Riding the Technol-
ogy Wave: Effective Dashboard Data Visualization.
LNCS, 6771(PART 1):249–258.
Qi, C. R., Su, H., Mo, K., and Guibas, L. J. (2017a). Point-
Net: Deep learning on point sets for 3D classification
and segmentation. In Proc. - CVPR 2017, volume
2017-Janua, pages 77–85. IEEE Inc.
Qi, C. R., Yi, L., Su, H., Guibas, L. J., Li, C. R. Q., Hao,
Y., Leonidas, S., and Guibas, J. (2017b). PointNet++:
Deep hierarchical feature learning on point sets in a
metric space. In Advances in Neural Information Pro-
cessing Systems, volume 2017, pages 5100–5109.
Richter, R. and D
¨
ollner, J. (2014). Concepts and techniques
for integration, analysis and visualization of massive
3D point clouds. Computers, Environment and Urban
Systems, 45:114–124.
Sampathkmar, U., Surya Prasath, V. B., Meenay, S., and
Palaniappan, K. (2017). Assisted ground truth gener-
ation using interactive segmentation on a visualization
and annotation tool. Proc. - Applied Imagery Pattern
Recognition Workshop.
Thomas, H., Qi, C. R., Deschaud, J. E., Marcotegui, B.,
Goulette, F., and Guibas, L. (2019). KPConv: Flexible
and deformable convolution for point clouds. In Proc.
of the IEEE Int. Conf. on Computer Vision, volume
2019-Octob, pages 6410–6419. IEEE Inc.
Yan, X., Zheng, C., Li, Z., Wang, S., and Cui, S. (2020).
PointASNL: Robust Point Clouds Processing Using
Nonlocal Neural Networks With Adaptive Sampling.
In Proc. of the IEEE CVPR, pages 5588–5597. IEEE.
Yi, L., Kim, V. G., Ceylan, D., Shen, I. C., Yan, M., Su, H.,
Lu, C., Huang, Q., Sheffer, A., and Guibas, L. (2016).
A scalable active framework for region annotation in
3D shape collections. ACM Trans. on Graphics, 35(6).
Zhou, J. and Chen, F. (2018). 2D Transparency
Space—Bring Domain Users and Machine Learning
Experts Together. pages 3–19.
Zoumpekas, T., Molina, G., Salam
´
o, M., and Puig, A.
(2021). Benchmarking deep learning models on point
cloud segmentation. volume 339 of Frontiers in Ar-
tificial Intelligence and Applications, pages 335–344.
IOS Press.
VISAPP 2022 - 17th International Conference on Computer Vision Theory and Applications
410