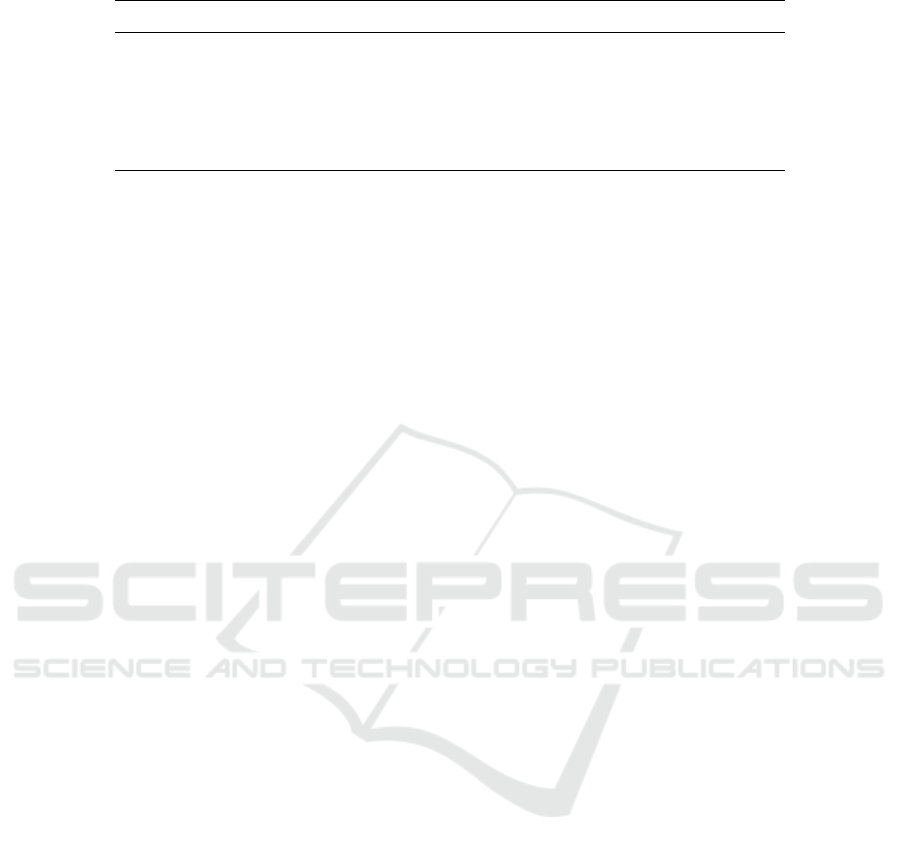
Table 5: The Accuracy (%) of Each Facial Expression in Qualitative Evaluation.
Facial Expression Patch-Based Isola’s Yi’s Ours Real Image Average
Neutral 100 100 100 100 90 98
Happy 95 85 55 75 100 82
Angry 100 95 100 100 100 99
Sad 95 95 90 95 95 94
Surprise 90 100 100 100 100 98
Average 96 95 89 94 97
7 LIMITATIONS
The limitations of this study are that the result may
get worse if the given face image is wearing a mask
different from the one used in the training dataset and
that the method does not support video yet. Therefore,
as future research, we need to develop a more robust
program that can handle any mask input and do real-
time face completion using video input.
8 CONCLUSION
In this study, we proposed a machine learning based
approach to complete hidden parts by face masks in
consideration of facial landmarks and pixel errors.
We utilized a GAN-based model as a baseline and
modified the loss function formula of the generator
to calculate and update the errors in the coordinates of
facial feature points and pixel values in the masked re-
gion, which enabled us to generate images with higher
quality than existing methods.
REFERENCES
Anwar, A. and Raychowdhury, A. (2020). Masked
face recognition for secure authentication. ArXiv,
abs/2008.11104.
Cai, J., Han, H., Shan, S., and Chen, X. (2020). Fcsr-gan:
Joint face completion and super-resolution via multi-
task learning. IEEE Transactions on Biometrics, Be-
havior, and Identity Science, 2(2):109–121.
Goodfellow, I., Pouget-Abadie, J., Mirza, M., Xu, B.,
Warde-Farley, D., Ozair, S., Courville, A., and Ben-
gio, Y. (2014). Generative adversarial nets. In Ad-
vances in Neural Information Processing Systems,
volume 27, pages 2672–2680. Curran Associates, Inc.
Isola, P., Zhu, J., Zhou, T., and Efros, A. A. (2017). Image-
to-image translation with conditional adversarial net-
works. In 2017 IEEE Conference on Computer Vision
and Pattern Recognition, pages 5967–5976.
Karras, T., Laine, S., and Aila, T. (2019). A style-based
generator architecture for generative adversarial net-
works. In 2019 IEEE/CVF Conference on Computer
Vision and Pattern Recognition, pages 4396–4405.
Karras, T., Laine, S., Aittala, M., Hellsten, J., Lehtinen, J.,
and Aila, T. (2020). Analyzing and improving the
image quality of stylegan. In 2020 IEEE/CVF Con-
ference on Computer Vision and Pattern Recognition,
pages 8107–8116.
King, D. E. (2009). Dlib-ml: A machine learning toolkit.
Journal of Machine Learning Research, 10(60):1755–
1758.
Lundqvist, D., Flykt, A., and
¨
Ohman, A. (1998). The
Karolinska Directed Emotional Faces. Karolinska In-
stitutet.
Mirza, M. and Osindero, S. (2014). Conditional generative
adversarial nets. ArXiv, abs/1411.1784.
Ronneberger, O., P.Fischer, and Brox, T. (2015). U-
net: Convolutional networks for biomedical im-
age segmentation. In Medical Image Computing
and Computer-Assisted Intervention, volume 9351 of
LNCS, pages 234–241. Springer.
Telea, A. (2004). An image inpainting technique based on
the fast marching method. Journal of Graphics Tools,
9.
Tsujimura, Y., Nishimura, S., Iijima, A., Kobayashi, R., and
Miyajima, N. (2020). Comparing different levels of
smiling with and without a surgical mask. Journal of
Comprehensive Nursing Research, 19(2):3–9.
Wang, M., Wen, X., and Hu, S. (2019). Faithful face image
completion for hmd occlusion removal. In 2019 IEEE
International Symposium on Mixed and Augmented
Reality Adjunct, pages 251–256.
Xie, J., Xu, L., and Chen, E. (2012). Image denoising and
inpainting with deep neural networks. In Advances in
Neural Information Processing Systems, volume 25,
pages 341–349.
Yi, J., Ud Din, N., Javed, K., and Bae, S. (2020). A
novel gan-based network for unmasking of masked
face. IEEE Access, 8:44276–44287.
Yu, J., Lin, Z., Yang, J., Shen, X., Lu, X., and Huang, T.
(2019). Free-form image inpainting with gated convo-
lution. In 2019 IEEE/CVF International Conference
on Computer Vision, pages 4470–4479.
Yu, J., Lin, Z., Yang, J., Shen, X., Lu, X., and Huang, T. S.
(2018). Generative image inpainting with contextual
attention. In 2018 IEEE/CVF Conference on Com-
puter Vision and Pattern Recognition, pages 5505–
5514.
Zhang, Z., Song, Y., and Qi, H. (2017). Age pro-
gression/regression by conditional adversarial autoen-
coder. In 2017 IEEE Conference on Computer Vision
and Pattern Recognition, pages 4352–4360.
GAN-based Face Mask Removal using Facial Landmarks and Pixel Errors in Masked Region
133