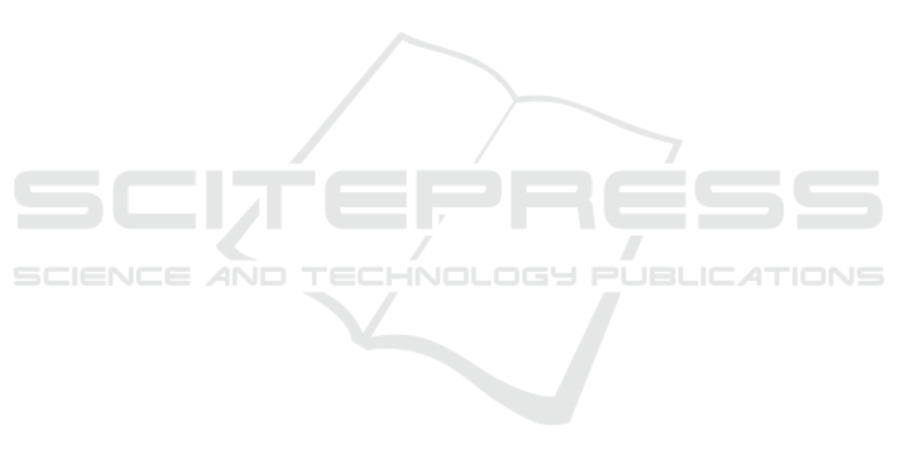
Govind, D., Santo, B. A., Ginley, B., Yacoub, R., Rosen-
berg, A. Z., Jen, K.-Y., Walavalkar, V., Wilding, G. E.,
Worral, A. M., Mohammad, I., and Sarder, P. (2021b).
Automated detection and quantification of Wilms’ Tu-
mor 1-positive cells in murine diabetic kidney dis-
ease. In Tomaszewski, J. E. and Ward, A. D., editors,
Medical Imaging 2021: Digital Pathology, volume
11603, pages 76–82. International Society for Optics
and Photonics, SPIE.
Huang, G., Liu, Z., van der Maaten, L., and Weinberger,
K. Q. (2018). Densely connected convolutional net-
works.
James, G., Witten, D., Hastie, T., and Tibshirani, R. (2013).
An Introduction to Statistical Learning. Springer texts
in statistics.
Jayapandian, C. P., Chen, Y., Janowczyk, A. R., Palmer,
M. B., Cassol, C. A., Sekulic, M., Hodgin, J. B.,
Zee, J., Hewitt, S. M., and Toole, J. O. (2021). De-
velopment and evaluation of deep learning-based seg-
mentation of histologic structures in the kidney cortex
with multiple histologic stains. Kidney international,
99(1)(June 2020):86–101.
Jiang, L., Chen, W., Dong, B., Mei, K., Zhu, C.,
Liu, J., Cai, M., Yan, Y., Wang, G., and Zuo,
L. (2021). MACHINE LEARNING , COMPU-
TATIONAL PATHOLOGY , AND BIOPHYSICAL
IMAGING A Deep Learning-Based Approach for
Glomeruli Instance Segmentation from Multistained
Renal Biopsy Pathologic Images. The American Jour-
nal of Pathology, 191(8):1431–1441.
Kannan, S., Morgan, L. A., Liang, B., Cheung, M. G., Lin,
C. Q., Mun, D., Nader, R. G., Belghasem, M. E., Hen-
derson, J. M., Francis, J. M., Chitalia, V. C., and Ko-
lachalama, V. B. (2019). Segmentation of Glomeruli
Learning. Kidney International Reports, 4(7):955–
962.
Maraszek, K. E., Santo, B. A., Yacoub, R., Tomaszewski,
J. E., Mohammad, I., Worral, A. M., and Sarder,
P. (2020). The presence and location of podocytes
in glomeruli as affected by diabetes mellitus. In
Tomaszewski, J. E. and Ward, A. D., editors, Medi-
cal Imaging 2020: Digital Pathology, volume 11320,
pages 298–307. International Society for Optics and
Photonics, SPIE.
Mathur, P., Ayyar, M. P., Shah, R. R., and Sharma, S. G.
(2019). Exploring classification of histological dis-
ease biomarkers from renal biopsy images. In 2019
IEEE Winter Conference on Applications of Computer
Vision (WACV), pages 81–90.
Merkel, D. (2014). Docker: Lightweight linux containers
for consistent development and deployment. Linux J.,
2014(239).
Nagata, M. (2016). Podocyte injury and its consequences.
Kidney international, 89(6):1221–1230.
O’Malley, T., Bursztein, E., Long, J., Chollet, F., Jin,
H., Invernizzi, L., et al. (2019). Keras Tuner.
https://github.com/keras-team/keras-tuner.
Russakovsky, O., Deng, J., Su, H., Krause, J., Satheesh,
S., Ma, S., Huang, Z., Karpathy, A., Khosla, A.,
Bernstein, M., Berg, A. C., and Fei-Fei, L. (2015).
ImageNet Large Scale Visual Recognition Challenge.
International Journal of Computer Vision (IJCV),
115(3):211–252.
Saga, N., Sakamoto, K., Matsusaka, T., and Nagata, M.
(2021). Glomerular filtrate affects the dynamics of
podocyte detachment in a model of diffuse toxic
podocytopathy. Kidney International.
Salas, J., de Barros Vidal, F., and Martinez-Trinidad, F.
(2019). Deep learning: Current state. IEEE Latin
America Transactions, 17(12):1925–1945.
Silva, P., Luz, E., Silva, G., Moreira, G., Wanner, E., Vidal,
F., and Menotti, D. (2020). Towards better heartbeat
segmentation with deep learning classification. Scien-
tific Reports, 10(1):20701.
Srinidhi, C. L., Ciga, O., and Martel, A. L. (2021). Deep
neural network models for computational histopathol-
ogy: A survey. Medical Image Analysis, 67:101813.
Szegedy, C., Vanhoucke, V., Ioffe, S., Shlens, J., and Wojna,
Z. (2015). Rethinking the inception architecture for
computer vision.
Thomas, S. M., Lefevre, J. G., Baxter, G., and Hamil-
ton, N. A. (2021). Interpretable deep learning sys-
tems for multi-class segmentation and classification of
non-melanoma skin cancer. Medical Image Analysis,
68:101915.
Tipping, M. E. and Bishop, C. M. (2006). Mixtures of
Probabilistic Principal Component Analysers. Neural
Computation 11(2).
Uchino, E., Suzuki, K., Sato, N., Kojima, R., and Tamada,
Y. (2020). Classification of glomerular pathological
findings using deep learning and nephrologist – AI
collective intelligence approach. International Jour-
nal of Medical Informatics, pages 1–14.
Ullah, A., Ahmad, J., Muhammad, K., Sajjad, M., and Baik,
S. W. (2018). Action recognition in video sequences
using deep bi-directional lstm with cnn features. IEEE
Access, 6:1155–1166.
Yang, C.-K., Lee, C.-Y., Wang, H.-S., Huang, S.-C., Liang,
P.-I., Chen, J.-S., Kuo, C.-F., Tu, K.-H., Yeh, C.-Y.,
and Chen, T.-D. (2021). Glomerular disease classifi-
cation and lesion identification by machine learning.
Biomedical Journal.
Yari, Y., Nguyen, T. V., and Nguyen, H. T. (2020). Deep
learning applied for histological diagnosis of breast
cancer. IEEE Access, 8:162432–162448.
Zeng, C., Nan, Y., Xu, F., Lei, Q., Li, F., Chen, T., Liang,
S., Hou, X., Lv, B., Liang, D., Luo, W., Lv, C., Li, X.,
Xie, G., and Liu, Z. (2020). Identification of glomeru-
lar lesions and intrinsic glomerular cell types in kid-
ney diseases via deep learning. The Journal of Pathol-
ogy, 252(1):e5491.
Zimmermann, M., Klaus, M., Wong, M. N., Thebille, A.-
K., Gernhold, L., Kuppe, C., Halder, M., Kranz, J.,
Wanner, N., Braun, F., Wulf, S., Wiech, T., Panzer,
U., Krebs, C. F., Hoxha, E., Kramann, R., Huber,
T. B., Bonn, S., and Puelles, V. G. (2021). Deep learn-
ing–based molecular morphometrics for kidney biop-
sies. JCI Insight, 6(7).
VISAPP 2022 - 17th International Conference on Computer Vision Theory and Applications
412