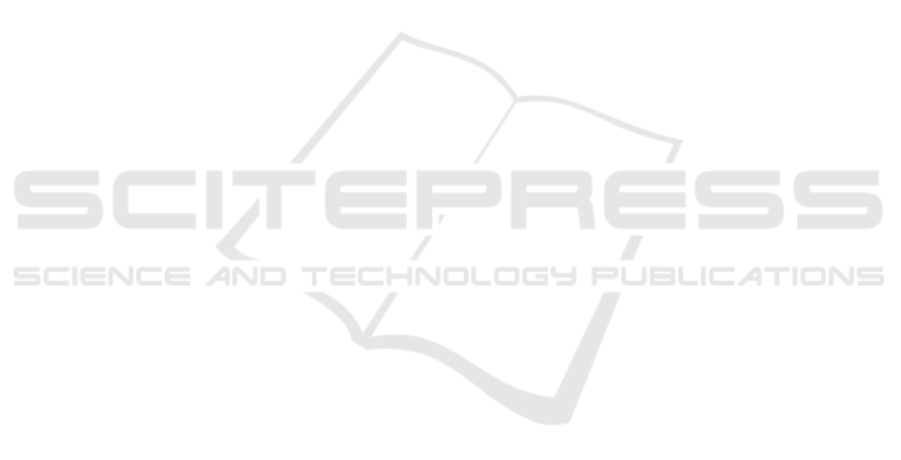
5 CONCLUSION
In this paper, a framework is proposed to learn an effi-
cient low-dimensional representation of fine-grained
actions. The fixed dimensional attribute vector per-
forms on-par when compared with the other super-
vised techniques on JIGSAWS, KSCGR, and MPII
cooking2 datasets. The effectiveness of the attribute
vector for classification on the KSCGR dataset proves
that the proposed method performs better even when
there is a few number of samples for each action. We
demonstrate the generalization of the proposed ap-
proach by evaluating on a wide variety of fine-grained
action datasets. Also, the proposed approach can be
adapted in applications such as medical, elderly assis-
tance, autonomous vehicles etc.
REFERENCES
A, S., K, K., D, D., G, M., and H, S. (2013). Kitchen
scene context based gesture recognition: A contest
in icpr2012. International Workshop on Depth Image
Analysis and Applications, 7854:168–185.
Alexandros, I., Anastastios, T., and Ioannis, P. (2014). Dis-
criminant bag of words based representation for hu-
man action recognition. Pattern Recognition Letters,
49:185–192.
Andrej, K., George, T., Sanketh, S., Thomas, L., Rahul, S.,
and Li, F.-F. (2014). Large-scale video classification
with convolutional neural networks. In Proceedings of
the IEEE conference on Computer Vision and Pattern
Recognition, pages 1725–1732.
Cheng, M., Zhang, Z., Lin, W., and Torr, P. (2014). Bing:
Binarized normed gradients for objectness estimation
at 300fps. In IEEE Conference on Computer Vision
and Pattern Recognition (CVPR), pages 3286–3293.
Fawaz, H. I., Forestier, G., Weber, J., Idoumghar, L., and
Muller, P. (2018). Evaluating surgical skills from
kinematic data using convolutional neural networks.
CoRR, abs/1806.02750.
Forestier, G., Petitjean, F., Senin, P., Despinoy, F., and Jan-
nin, P. (2017). Discovering discriminative and inter-
pretable patterns for surgical motion analysis. In Con-
ference on Artificial Intelligence in Medicine in Eu-
rope, pages 136–145. Springer.
Funke, I., Mees, S. T., Weitz, J., and Speidel, S. (2019).
Video-based surgical skill assessment using 3d con-
volutional neural networks. CoRR, abs/1903.02306.
Gao, Y., Vedula, S. S., Reiley, C. E., Ahmidi, N., Varadara-
jan, B., Lin, H. C., Tao, L., Zappella, L., B
´
ejar, B.,
Yuh, D. D., et al. (2014). Jhu-isi gesture and skill
assessment working set (jigsaws): A surgical activity
dataset for human motion modeling. In Miccai work-
shop: M2cai, volume 3, page 3.
Granada, R. L., Monteiro, J., Barros, R. C., and Meneguzzi,
F. R. (2017). A deep neural architecture for kitchen
activity recognition. In The Thirtieth International
Flairs Conference.
Hao, Y., Chunfeng, Y., Bing, L., Yang, D., Junliang, X.,
Weiming, H., and J, M. S. (2019). Asymmetric 3d
convolutional neural networks for action recognition.
Pattern recognition, 85:1–12.
Hara, K., Kataoka, H., and Satoh, Y. (2018). Can spa-
tiotemporal 3d cnns retrace the history of 2d cnns and
imagenet? In Proceedings of the IEEE conference
on Computer Vision and Pattern Recognition, pages
6546–6555.
Hearst, M. A., Dumais, S. T., Osuna, E., Platt, J., and
Scholkopf, B. (1998). Support vector machines. IEEE
Intelligent Systems and their applications, 13(4):18–
28.
Heeseung, K., Yeonho, K., S, L. J., and Minsu, C. (2018).
First person action recognition via two-stream convnet
with long-term fusion pooling. Pattern Recognition
Letters, 112:161–167.
Herve, J., Florent, P., Matthijs, D., Jorge, S., Patrick, P., and
Cordelia, S. (2011). Aggregating local image descrip-
tors into compact codes. IEEE transactions on pattern
analysis and machine intelligence, 34(9):1704–1716.
Ivan, L. (2005). On space-time interest points. International
journal of computer vision, 64(2-3):107–123.
Lin, J., Gan, C., and Han, S. (2019). Tsm: Temporal shift
module for efficient video understanding. In Proceed-
ings of the IEEE/CVF International Conference on
Computer Vision, pages 7083–7093.
M., M., N., M., Y., L., A., L., and R, S. (2018). Region-
sequence based six-stream cnn features for general
and fine-grained human action recognition in videos.
Pattern Recognition, 76:506–521.
Manel, S., Mahmoud, M., and Ben, A. C. (2015). Human
action recognition based on multi-layer fisher vector
encoding method. Pattern Recognition Letters, 65:37–
43.
Maria, C. J. and Joan, C. (2018). Human action recognition
by means of subtensor projections and dense trajecto-
ries. Pattern Recognition, 81:443–455.
Miao, M., Naresh, M., Yibin, L., Ales, L., and Rustam, S.
(2018). Region-sequence based six-stream cnn fea-
tures for general and fine-grained human action recog-
nition in videos. Pattern Recognition, 76:506–521.
Ni, B., Paramathayalan, V. R., and Moulin, P. (2014). Multi-
ple granularity analysis for fine-grained action detec-
tion. In IEEE Conference on Computer Vision and
Pattern Recognition (CVPR), pages 756–763.
Ni, B., Yang, X., and Gao, S. (2016). Progressively parsing
interactional objects for fine grained action detection.
In IEEE Conference on Computer Vision and Pattern
Recognition (CVPR), pages 1020–1028.
Reynolds, D., Quatieri, T. F., and Dunn, R. B. (2000).
Speaker verification using adapted gaussian mixture
models. In Digital Signal Process., volume 10, pages
19–41.
Rohrbach, M., Rohrbach, A., Regneri, M., Amin, S., An-
driluka, M., Pinkal, M., and Schiele, B. (2016). Rec-
ognizing fine-grained and composite activities using
VISAPP 2022 - 17th International Conference on Computer Vision Theory and Applications
142