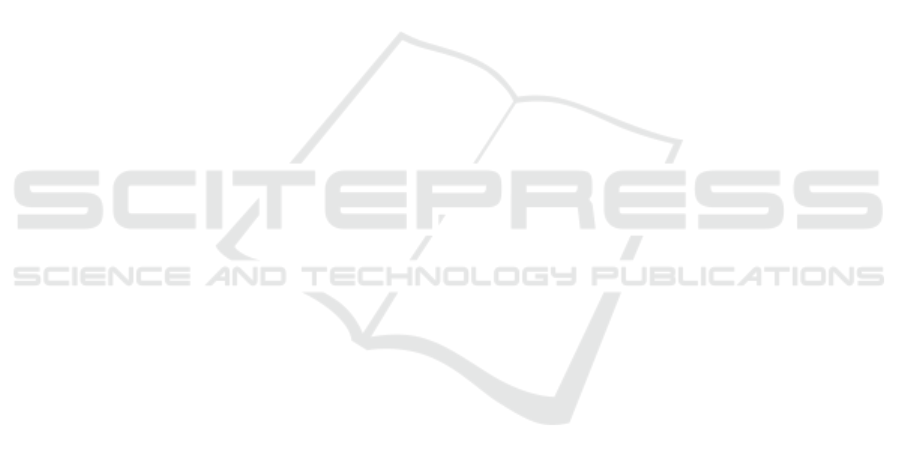
We demonstrated that DPEN is able to accurately
infer the intensity of the distortions affecting the in-
put sequences, and compared MdVRNet with another
existing state-of-the-art method for video restoration,
showing both quantitatively and qualitatively the su-
periority of the proposed approach in restoring multi-
distorted videos. Additionally, we provided an abla-
tion study in which we demonstrated that the DPEN
and MRB modules, as well as the two-stage restora-
tion process of MdVRNet, are all essential to obtain
the best restoration performance.
As future developments, we plan to investigate
other types of degradation operators, such as blur
caused by motion, and to improve the model via neu-
ral architecture search (Bianco et al., 2020).
REFERENCES
Bianco, S., Buzzelli, M., Ciocca, G., and Schettini, R.
(2020). Neural architecture search for image saliency
fusion. Information Fusion, 57:89–101.
Caballero, J., Ledig, C., Aitken, A., Acosta, A., Totz, J.,
Wang, Z., and Shi, W. (2017). Real-time video super-
resolution with spatio-temporal networks and motion
compensation. In 2017 IEEE Conference on Com-
puter Vision and Pattern Recognition (CVPR), pages
2848–2857.
Deng, J., Wang, L., Pu, S., and Zhuo, C. (2020).
Spatio-temporal deformable convolution for com-
pressed video quality enhancement. Proceedings
of the AAAI Conference on Artificial Intelligence,
34:10696–10703.
Guan, Z., Xing, Q., Xu, M., Yang, R., Liu, T., and Wang, Z.
(2019). Mfqe 2.0: A new approach for multi-frame
quality enhancement on compressed video. IEEE
Transactions on Pattern Analysis and Machine Intel-
ligence, PP:1–1.
Hu, J., Shen, L., and Sun, G. (2018). Squeeze-and-
excitation networks. In 2018 IEEE/CVF Conference
on Computer Vision and Pattern Recognition, pages
7132–7141.
Ioffe, S. and Szegedy, C. (2015). Batch normalization:
Accelerating deep network training by reducing inter-
nal covariate shift. In Proceedings of the 32nd Inter-
national Conference on International Conference on
Machine Learning - Volume 37, page 448–456.
Jaderberg, M., Simonyan, K., Zisserman, A., and
Kavukcuoglu, K. (2015). Spatial transformer net-
works. Advances in Neural Information Processing
Systems 28 (NIPS 2015).
Jo, Y., Oh, S. W., Kang, J., and Kim, S. J. (2018). Deep
video super-resolution network using dynamic upsam-
pling filters without explicit motion compensation. In
2018 IEEE/CVF Conference on Computer Vision and
Pattern Recognition, pages 3224–3232.
Kingma, D. and Ba, J. (2014). Adam: A method for
stochastic optimization. International Conference on
Learning Representations.
Mehta, S., Kumar, A., Reda, F., Nasery, V., Mulukutla, V.,
Ranjan, R., and Chandra, V. (2021). Evrnet: Efficient
video restoration on edge devices. In Proceedings of
the 29th ACM International Conference on Multime-
dia, pages 983–992.
Nah, S., Timofte, R., Gu, S., Baik, S., Hong, S., Moon,
G., Son, S., and Mu Lee, K. (2019). Ntire 2019
challenge on video super-resolution: Methods and re-
sults. In Proceedings of the IEEE/CVF Conference on
Computer Vision and Pattern Recognition Workshops,
pages 0–0.
Nair, V. and Hinton, G. E. (2010). Rectified linear units im-
prove restricted boltzmann machines. ICML’10, page
807–814, Madison, WI, USA. Omnipress.
Pont-Tuset, J., Perazzi, F., Caelles, S., Arbel
´
aez, P.,
Sorkine-Hornung, A., and Van Gool, L. (2017). The
2017 davis challenge on video object segmentation.
arXiv:1704.00675.
Shi, W., Caballero, J., Husz
´
ar, F., Totz, J., Aitken, A. P.,
Bishop, R., Rueckert, D., and Wang, Z. (2016). Real-
time single image and video super-resolution using an
efficient sub-pixel convolutional neural network. In
Proceedings of the IEEE conference on computer vi-
sion and pattern recognition, pages 1874–1883.
Su, S., Delbracio, M., Wang, J., Sapiro, G., Heidrich, W.,
and Wang, O. (2017). Deep video deblurring for hand-
held cameras. In 2017 IEEE Conference on Computer
Vision and Pattern Recognition (CVPR), pages 237–
246.
Tassano, M., Delon, J., and Veit, T. (2019). Dvdnet: A fast
network for deep video denoising. In 2019 IEEE In-
ternational Conference on Image Processing (ICIP),
pages 1805–1809.
Tassano, M., Delon, J., and Veit, T. (2020). Fastdvdnet: To-
wards real-time deep video denoising without flow es-
timation. In Proceedings of the IEEE/CVF Conference
on Computer Vision and Pattern Recognition, pages
1354–1363.
Wang, X., Chan, K. C., Yu, K., Dong, C., and Change Loy,
C. (2019). Edvr: Video restoration with enhanced de-
formable convolutional networks. In Proceedings of
the IEEE/CVF Conference on Computer Vision and
Pattern Recognition Workshops, pages 1954–1963.
Wang, Z., Bovik, A., Sheikh, H., and Simoncelli, E. (2004).
Image quality assessment: From error visibility to
structural similarity. Image Processing, IEEE Trans-
actions on, 13:600 – 612.
Xue, T., Chen, B., Wu, J., Wei, D., and Freeman, W. (2019).
Video enhancement with task-oriented flow. Interna-
tional Journal of Computer Vision, 127.
Yu, K., Dong, C., Lin, L., and Loy, C. C. (2018). Crafting a
toolchain for image restoration by deep reinforcement
learning. In Proceedings of the IEEE conference on
computer vision and pattern recognition, pages 2443–
2452.
VISAPP 2022 - 17th International Conference on Computer Vision Theory and Applications
426