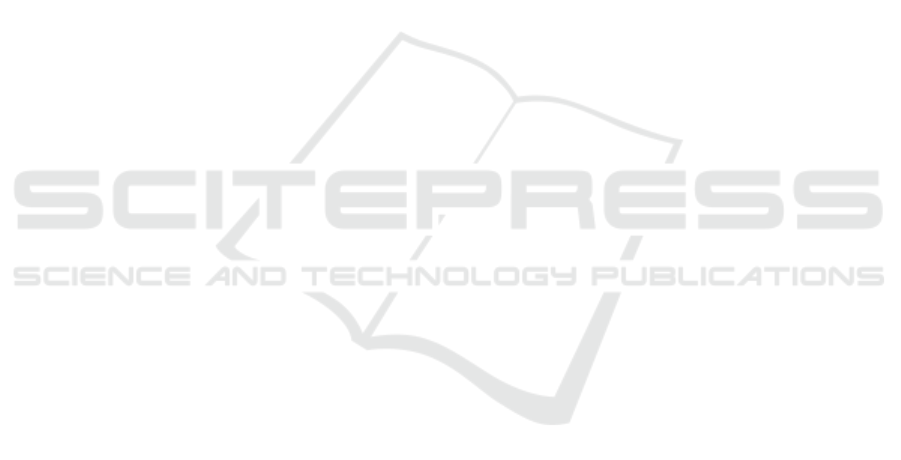
bigger than the one in the simulation and, ideally, the
simulation should be able to run using only a wire-
free headset so that the users can move around freely
without worrying about cables.
Future work will include a deeper analysis of the
acquired biosignals and relate them to user move-
ments and events responses recorded for the whole
group of participants. It would also be interesting to
use a signal to detect anxiety such as eyes movements.
REFERENCES
Al-Yacoub, A., Buerkle, A., Flanagan, M., Ferreira, P.,
Hubbard, E.-M., and Lohse, N. (2020). Effec-
tive human-robot collaboration through wearable sen-
sors. In 2020 25th IEEE International Conference
on Emerging Technologies and Factory Automation
(ETFA), volume 1, pages 651–658.
Aqajari, S. A. H., Naeini, E. K., Mehrabadi, M. A., Labbaf,
S., Rahmani, A. M., and Dutt, N. (2020). Gsr analy-
sis for stress: Development and validation of an open
source tool for noisy naturalistic gsr data.
Benedek, M. and Kaernbach, C. (2010a). A continuous
measure of phasic electrodermal activity. Journal of
Neuroscience Methods, 190(1):80–91.
Benedek, M. and Kaernbach, C. (2010b). Decomposition
of skin conductance data by means of nonnegative de-
convolution. Psychophysiology, 47(4):647–658.
Bi, L., xin’an, F., and Liu, Y. (2013). Eeg-based brain-
controlled mobile robots: A survey. Human-Machine
Systems, IEEE Transactions on, 43:161–176.
Blow, M., Dautenhahn, K., Appleby, A., Nehaniv, C. L.,
and Lee, D. (2006). The art of designing robot faces:
Dimensions for human-robot interaction. In Proceed-
ings of the 1st ACM SIGCHI/SIGART Conference on
Human-Robot Interaction, HRI ’06, page 331–332,
New York, NY, USA. Association for Computing Ma-
chinery.
da Silva, H. P., Guerreiro, J., Lourenc¸o, A., Fred, A., and
Martins, R. (2014). Bitalino: A novel hardware frame-
work for physiological computing. In Proceedings of
the International Conference on Physiological Com-
puting Systems - Volume 1: PhyCS,, pages 246–253.
INSTICC, SciTePress.
de Giorgio, A., Romero, M., Onori, M., and Wang, L.
(2017). Human-machine collaboration in virtual re-
ality for adaptive production engineering. Procedia
Manufacturing, 11:1279 – 1287. 27th International
Conference on Flexible Automation and Intelligent
Manufacturing, FAIM2017, 27-30 June 2017, Mod-
ena, Italy.
Duguleana, M., Barbuceanu, F. G., and Mogan, G. (2011).
Evaluating human-robot interaction during a manipu-
lation experiment conducted in immersive virtual re-
ality. In Shumaker, R., editor, Virtual and Mixed Real-
ity - New Trends, pages 164–173, Berlin, Heidelberg.
Springer Berlin Heidelberg.
Hussein, A., Garcia, F., and Olaverri Monreal, C. (2018).
Ros and unity based framework for intelligent vehi-
cles control and simulation.
Kidd, C. D. and Breazeal, C. (2008). Robots at home:
Understanding long-term human-robot interaction. In
2008 IEEE/RSJ International Conference on Intelli-
gent Robots and Systems, pages 3230–3235.
Knudsen, M. and Kaivo-oja, J. (2020). Collaborative
robots: Frontiers of current literature. Journal of In-
telligent Systems: Theory and Applications, 3:13–20.
Kr
¨
uger, J., Lien, T., and Verl, A. (2009). Cooperation of
human and machines in assembly lines. CIRP Annals,
58(2):628–646.
Laugwitz, B., Held, T., and Schrepp, M. (2008). Construc-
tion and evaluation of a user experience questionnaire.
volume 5298, pages 63–76.
Liu, H., Qu, D., Xu, F., Zou, F., Song, J., and Jia, K. (2019).
A human-robot collaboration framework based on hu-
man motion prediction and task model in virtual envi-
ronment. In 2019 IEEE 9th Annual International Con-
ference on CYBER Technology in Automation, Con-
trol, and Intelligent Systems (CYBER), pages 1044–
1049.
Lutin, E., Hashimoto, R., De Raedt, W., and Van Hoof, C.
(2021). Feature extraction for stress detection in elec-
trodermal activity. In BIOSIGNALS, pages 177–185.
Maragkos, C., Vosniakos, G.-C., and Matsas, E. (2019).
Virtual reality assisted robot programming for hu-
man collaboration. Procedia Manufacturing, 38:1697
– 1704. 29th International Conference on Flexible
Automation and Intelligent Manufacturing ( FAIM
2019), June 24-28, 2019, Limerick, Ireland, Beyond
Industry 4.0: Industrial Advances, Engineering Edu-
cation and Intelligent Manufacturing.
Matsas, E. and Vosniakos, G.-C. (2017). Design of a vir-
tual reality training system for human–robot collabo-
ration in manufacturing tasks. International Journal
on Interactive Design and Manufacturing (IJIDeM),
11(2):139–153.
PLUX - Wireless Biosignals (2020). Electro-
dermal activity (eda) sensor user manual.
https://bitalino.com/storage/uploads/media/
electrodermal-activity-eda-user-manual.pdf. Ac-
cessed: 2021-09-27.
Rheinberg, F., Vollmeyer, R., and Engeser, S. (2006). Die
erfassung des flow-erlebens.
C¸
¨
ur
¨
ukl
¨
u, B., Dodig-Crnkovic, G., and Akan, B. (2010).
Towards industrial robots with human-like moral re-
sponsibilities. In 2010 5th ACM/IEEE International
Conference on Human-Robot Interaction (HRI), pages
85–86.
Stanford Artificial Intelligence Laboratory et al. (2020).
Robotic operating system.
Swartz Center for Computational Neuroscience (2021).
Labstreaminglayer. https://github.com/sccn/
labstreaminglayer. Accessed: 2021-09-27.
GRAPP 2022 - 17th International Conference on Computer Graphics Theory and Applications
78