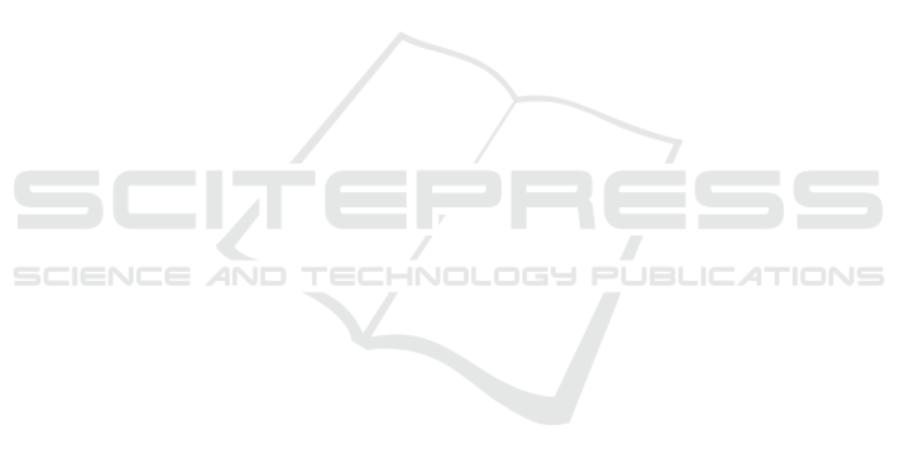
studies, this predictive model has lower performance
than other models. It has low sensitivity although the
reasons behind the low sensitivity could be linked to
the low sample size.
The SVM with linear and radial kernel had
the best performance on the test set for predict-
ing CV worsening events in CHF patients: AUC =
0.77, speci f icity = 0.79, sensitivity = 0.75 and G −
mean = 0.77. This indicate that SVM has a high abil-
ity to avoid false classification.
4 CONCLUSIONS
This work presents and compares predictive models
based on ML algorithms for the early prediction of
CV worsening events in CHF patients using few clin-
ical parameters.
Among the predictive models, SVM with linear
and radial kernel had the best performance on the test
set. As we showed, the decision tree is among all
the trained and tested models the most simple and in-
terpretable mainly by clinicians because it discovers
if-then rules as clinicians do. Conversely, although
SVM has the best performances, it is not “clinician
friendly”. The inability of SVMs in providing a sim-
ple and understandable interpretation of the classifica-
tion decisions is one of the main obstacles impeding
their application in the clinical practice.
As future work, we will reproduce these experi-
mental models on a larger size study and a shorter in-
terval occurring between two consecutive visits. A
follow-up based on shorter intervals could increase
both sensitivity and specificity of the models. Another
possible application could consider remote monitor-
ing, with the active help of either the patients them-
selves or their caregivers, to intervene as early as pos-
sible to avoid events and subsequent hospitalization.
In addition, techniques for rule extraction from SVM
could be adopted to ameliorate the aforementioned is-
sue.
ACKNOWLEDGEMENTS
This work has been partially supported by the indus-
trial research and development project “HEARTNET-
ICS - Advanced Analytics for Heart Diseases Man-
agement” (European Regional Development Fund,
Calabria Region Grant J58C17000150006, Italy,
2017-2020)
REFERENCES
Borer, J. S., B
¨
ohm, M., Ford, I., Komajda, M., Tavazzi,
L., Sendon, J. L., Alings, M., Lopez-de Sa, E., Swed-
berg, K., and Investigators, S. (2012). Effect of ivabra-
dine on recurrent hospitalization for worsening heart
failure in patients with chronic systolic heart failure:
the shift study. European heart journal, 33(22):2813–
2820.
Bredy, C., Ministeri, M., Kempny, A., Alonso-Gonzalez,
R., Swan, L., Uebing, A., Diller, G.-P., Gatzoulis,
M. A., and Dimopoulos, K. (2018). New york heart
association (nyha) classification in adults with con-
genital heart disease: relation to objective measures
of exercise and outcome. European Heart Journal-
Quality of Care and Clinical Outcomes, 4(1):51–58.
Breiman, L. (2001). Random forests. Mach Learn, 45:5–32.
Burges, C. J. (1998). A tutorial on support vector machines
for pattern recognition. Data mining and knowledge
discovery, 2(2):121–167.
Cortes, C. and Vapnik, V. (1995). Support-vector networks.
Machine learning, 20(3):273–297.
Dunlay, S. M., Redfield, M. M., Weston, S. A., Therneau,
T. M., Hall Long, K., Shah, N. D., and Roger, V. L.
(2009). Hospitalizations after heart failure diagnosis:
a community perspective. Journal of the American
College of Cardiology, 54(18):1695–1702.
Eibe, F., Hall, M. A., and Witten, I. H. (2016). The weka
workbench. online appendix for data mining: practi-
cal machine learning tools and techniques. In Morgan
Kaufmann.
Fayyad, U., Piatetsky-Shapiro, G., and Smyth, P. (1996).
The kdd process for extracting useful knowledge from
volumes of data. Communications of the ACM,
39(11):27–34.
Groccia, M. C., Lofaro, D., Guido, R., Conforti, D., and
Sciacqua, A. (2018). Predictive models for risk as-
sessment of worsening events in chronic heart failure
patients. In 2018 Computing in Cardiology Confer-
ence (CinC), volume 45, pages 1–4. IEEE.
Jo, T. (2021). Machine Learning Foundations: Supervised,
Unsupervised, and Advanced Learning. Springer Na-
ture.
Klersy, C., De Silvestri, A., Gabutti, G., Regoli, F., and Au-
ricchio, A. (2009). A meta-analysis of remote moni-
toring of heart failure patients. Journal of the Ameri-
can College of Cardiology, 54(18):1683–1694.
Krenker, A., Be
ˇ
ster, J., and Kos, A. (2011). Introduction
to the artificial neural networks. Artificial Neural Net-
works: Methodological Advances and Biomedical Ap-
plications. InTech, pages 1–18.
McDonagh, T. A., Metra, M., Adamo, M., Gardner, R. S.,
Baumbach, A., B
¨
ohm, M., Burri, H., Butler, J.,
ˇ
Celutkien
˙
e, J., Chioncel, O., Cleland, J. G. F., Coats,
A. J. S., Crespo-Leiro, M. G., Farmakis, D., Gi-
lard, M., Heymans, S., Hoes, A. W., Jaarsma, T.,
Jankowska, E. A., Lainscak, M., Lam, C. S. P., Lyon,
A. R., McMurray, J. J. V., Mebazaa, A., Mindham,
R., Muneretto, C., Francesco Piepoli, M., Price, S.,
Rosano, G. M. C., Ruschitzka, F., Kathrine Skibelund,
HEALTHINF 2022 - 15th International Conference on Health Informatics
480