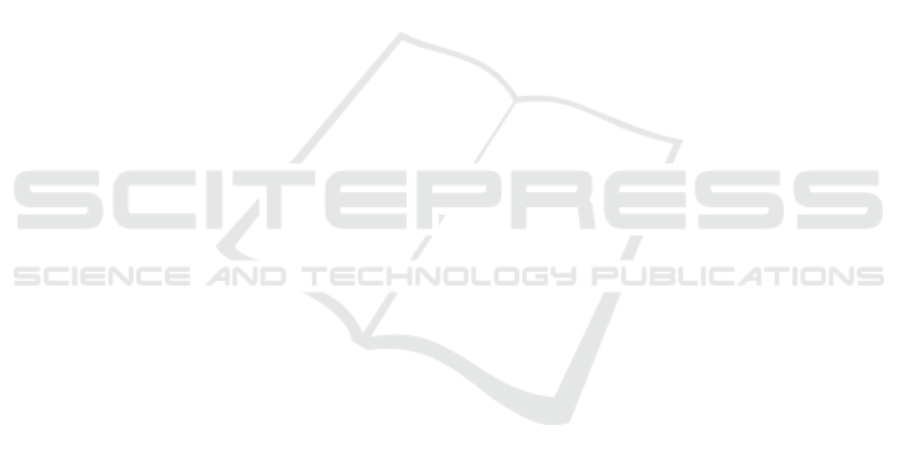
mine the final classification, which makes the system
robust. From this point of view, the method of classi-
fying feature maps using numerical relationships be-
tween weights is inherently flawed. The numerical
relationship of weights can not clearly represent the
category of feature maps.
The ability to activate complete target regions of
feature maps is found in fixed channels. This prop-
erty of neural networks applies to both cancerous and
normal tissue areas, treating both classes as targets
and activating the corresponding area completely. At
the same time, specific channels are invariant to tar-
get changes in the input image, they can stably output
the complete active area. In addition, this capability is
closely tied to channels from which feature maps can
be obtained once the channels are identified. There-
fore, after the training of neural network, the channels
can be screened with a small amount of data to deter-
mine the needed channels.
4 CONCLUSION
In this paper, we have taken an insight into the neu-
ral network. we have explained the weak correlation
between feature map and the weight of output layer.
The feature map directly related to target cannot be
selected out through the numerical characteristics of
weights. It was also found that the feature map in
specific channel has the ability to capture fixed fea-
ture. The conducted experiments prove that the fea-
ture maps which activate the more complete target re-
gion are output in fixed channels. This work can aid
other researchers in understanding and designing net-
works for image processing.
ACKNOWLEDGEMENTS
The authors gratelfully acknowledge financial support
from China Scholarship Council.
REFERENCES
Bazzani, L., Bergamo, A., Anguelov, D., and Torresani, L.
(2016). Self-taught object localization with deep net-
works. In 2016 IEEE Winter Conference on Applica-
tions of Computer Vision (WACV), pages 1–9.
Choe, J. and Shim, H. (2019). Attention-based dropout
layer for weakly supervised object localization. In
2019 IEEE/CVF Conference on Computer Vision and
Pattern Recognition (CVPR), pages 2214–2223.
Kim, D., Cho, D., Yoo, D., and Kweon, I. S. (2017). Two-
phase learning for weakly supervised object localiza-
tion. In 2017 IEEE International Conference on Com-
puter Vision (ICCV), pages 3554–3563.
Simonyan, K. and Zisserman, A. (2014). Very Deep Con-
volutional Networks for Large-Scale Image Recogni-
tion. arXiv e-prints, page arXiv:1409.1556.
Singh, K. K. and Lee, Y. J. (2017). Hide-and-seek: Forc-
ing a network to be meticulous for weakly-supervised
object and action localization. In 2017 IEEE Interna-
tional Conference on Computer Vision (ICCV), pages
3544–3553.
Wei, Y., Feng, J., Liang, X., Cheng, M.-M., Zhao, Y., and
Yan, S. (2017). Object region mining with adversarial
erasing: A simple classification to semantic segmen-
tation approach. In 2017 IEEE Conference on Com-
puter Vision and Pattern Recognition (CVPR), pages
6488–6496.
Xue, H., Liu, C., Wan, F., Jiao, J., Ji, X., and Ye, Q. (2019).
Danet: Divergent activation for weakly supervised ob-
ject localization. In 2019 IEEE/CVF International
Conference on Computer Vision (ICCV), pages 6588–
6597.
Zhou, B., Khosla, A., Lapedriza, A., Oliva, A., and Tor-
ralba, A. (2016). Learning deep features for discrimi-
native localization. In 2016 IEEE Conference on Com-
puter Vision and Pattern Recognition (CVPR), pages
2921–2929.
Zhou, B., Khosla, A., Lapedriza, g., Oliva, A., and Tor-
ralba, A. (2015). Object detectors emerge in deep
scene cnns. In International Conference on Learning
Representations.
Weakly Supervised Segmentation of Histopathology Images: An Insight in Feature Maps Ability for Learning Models Interpretation
427