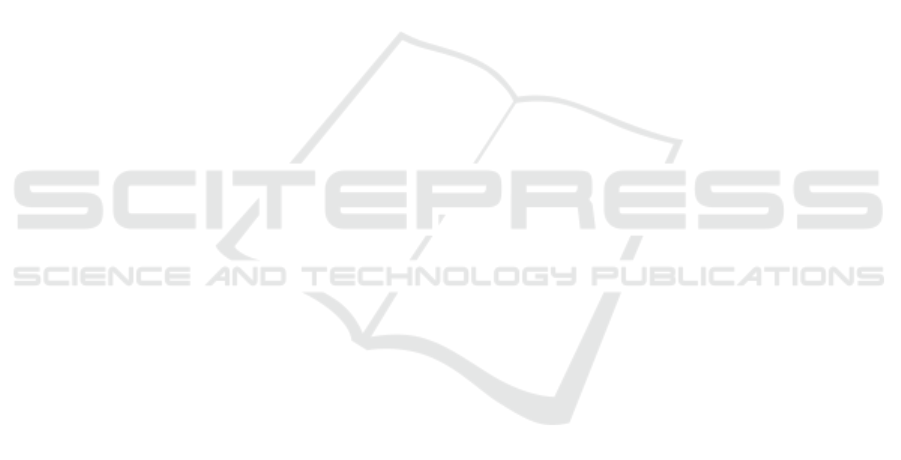
REFERENCES
Armeni, I., Sax, S., Zamir, A. R., and Savarese, S. (2017).
Joint 2d-3d-semantic data for indoor scene under-
standing. 1
Barnes, C., Shechtman, E., Finkelstein, A., and Goldman,
D. B. (2009). PatchMatch: A randomized corre-
spondence algorithm for structural image editing, vol-
ume 28. 3
Chang, A., Dai, A., Funkhouser, T., Halber, M., Niebner,
M., Savva, M., Song, S., Zeng, A., and Zhang, Y.
(2017). Matterport3d: Learning from rgb-d data in in-
door environments. In 2017 International Conference
on 3D Vision (3DV), pages 667–676. IEEE Computer
Society. 1
Chen, X., Kundu, K., Zhu, Y., Berneshawi, A. G., Ma, H.,
Fidler, S., and Urtasun, R. (2015). 3d object propos-
als for accurate object class detection. In Advances in
Neural Information Processing Systems, pages 424–
432. Citeseer. 2
Gatys, L. A., Ecker, A. S., and Bethge, M. (2016). Image
style transfer using convolutional neural networks. In
Proceedings of the IEEE conference on computer vi-
sion and pattern recognition, pages 2414–2423. 6
Gidaris, S. and Komodakis, N. (2015). Object detection
via a multi-region and semantic segmentation-aware
cnn model. In Proceedings of the IEEE international
conference on computer vision, pages 1134–1142. 2
Gkitsas, V., Sterzentsenko, V., Zioulis, N., Albanis, G., and
Zarpalas, D. (2021). Panodr: Spherical panorama di-
minished reality for indoor scenes. In Proceedings of
the IEEE/CVF Conference on Computer Vision and
Pattern Recognition, pages 3716–3726. 1, 2, 3, 4, 6,
7, 8
Goodfellow, I. J., Pouget-Abadie, J., Mirza, M., Xu, B.,
Warde-Farley, D., Ozair, S., Courville, A., and Ben-
gio, Y. (2014). Generative adversarial networks. 1
Iizuka, S., Simo-Serra, E., and Ishikawa, H. (2017). Glob-
ally and locally consistent image completion, vol-
ume 36. ACM New York, NY, USA. 3
Isola, P., Zhu, J.-Y., Zhou, T., and Efros, A. A. (2017).
Image-to-image translation with conditional adversar-
ial networks. In Proceedings of the IEEE conference
on computer vision and pattern recognition, pages
1125–1134. 2
Jaderberg, M., Mnih, V., Czarnecki, W. M., Schaul, T.,
Leibo, J. Z., Silver, D., and Kavukcuoglu, K. (2016).
Reinforcement learning with unsupervised auxiliary
tasks. 2
Johnson, J., Alahi, A., and Fei-Fei, L. (2016). Perceptual
losses for real-time style transfer and super-resolution.
In European conference on computer vision, pages
694–711. Springer. 6
Kingma, D. P. and Ba, J. (2014). Adam: A method for
stochastic optimization. 6
Li, J., Wang, N., Zhang, L., Du, B., and Tao, D. (2020). Re-
current feature reasoning for image inpainting. In Pro-
ceedings of the IEEE/CVF Conference on Computer
Vision and Pattern Recognition, pages 7760–7768. 3,
6, 8
Lim, J. H. and Ye, J. C. (2017). Geometric gan. 6
Lin, T.-Y., Goyal, P., Girshick, R., He, K., and Doll
´
ar, P.
(2017). Focal loss for dense object detection. In
Proceedings of the IEEE international conference on
computer vision, pages 2980–2988. 6
Liu, X., Gao, J., He, X., Deng, L., Duh, K., and Wang, Y.-
Y. (2015). Representation learning using multi-task
deep neural networks for semantic classification and
information retrieval. 2
Mori, S., Ikeda, S., and Saito, H. (2017). A survey of
diminished reality: Techniques for visually conceal-
ing, eliminating, and seeing through real objects, vol-
ume 9. SpringerOpen. 3
Nazeri, K., Ng, E., Joseph, T., Qureshi, F., and Ebrahimi,
M. (2019). Edgeconnect: Structure guided image in-
painting using edge prediction. In Proceedings of the
IEEE/CVF International Conference on Computer Vi-
sion Workshops, pages 0–0. 3
Park, T., Liu, M.-Y., Wang, T.-C., and Zhu, J.-Y. (2019).
Semantic image synthesis with spatially-adaptive nor-
malization. In Proceedings of the IEEE/CVF Con-
ference on Computer Vision and Pattern Recognition,
pages 2337–2346. 2
Paszke, A., Gross, S., Chintala, S., Chanan, G., Yang, E.,
DeVito, Z., Lin, Z., Desmaison, A., Antiga, L., and
Lerer, A. (2017). Automatic differentiation in pytorch.
6
Pinheiro, P. O., Lin, T.-Y., Collobert, R., and Doll
´
ar, P.
(2016). Learning to refine object segments. In Eu-
ropean conference on computer vision, pages 75–91.
Springer. 2
Ren, Y., Yu, X., Zhang, R., Li, T. H., Liu, S., and
Li, G. (2019). Structureflow: Image inpainting via
structure-aware appearance flow. In Proceedings of
the IEEE/CVF International Conference on Computer
Vision, pages 181–190. 3
Ronneberger, O., Fischer, P., and Brox, T. (2015). U-net:
Convolutional networks for biomedical image seg-
mentation. In International Conference on Medical
image computing and computer-assisted intervention,
pages 234–241. Springer. 7
Simonyan, K. and Zisserman, A. (2014). Very deep convo-
lutional networks for large-scale image recognition. 5
Sun, J., Yuan, L., Jia, J., and Shum, H.-Y. (2005). Image
completion with structure propagation. In ACM SIG-
GRAPH 2005 Papers, pages 861–868. 3
Xu, J. and Zheng, C. (2021). Linear semantics in gen-
erative adversarial networks. In Proceedings of the
IEEE/CVF Conference on Computer Vision and Pat-
tern Recognition, pages 9351–9360. 2, 3
Yu, J., Lin, Z., Yang, J., Shen, X., Lu, X., and Huang, T. S.
(2018). Generative image inpainting with contextual
attention. In Proceedings of the IEEE conference on
computer vision and pattern recognition, pages 5505–
5514. 3
Yu, J., Lin, Z., Yang, J., Shen, X., Lu, X., and Huang, T. S.
(2019). Free-form image inpainting with gated con-
volution. In Proceedings of the IEEE International
Conference on Computer Vision, pages 4471–4480. 3
VISAPP 2022 - 17th International Conference on Computer Vision Theory and Applications
460