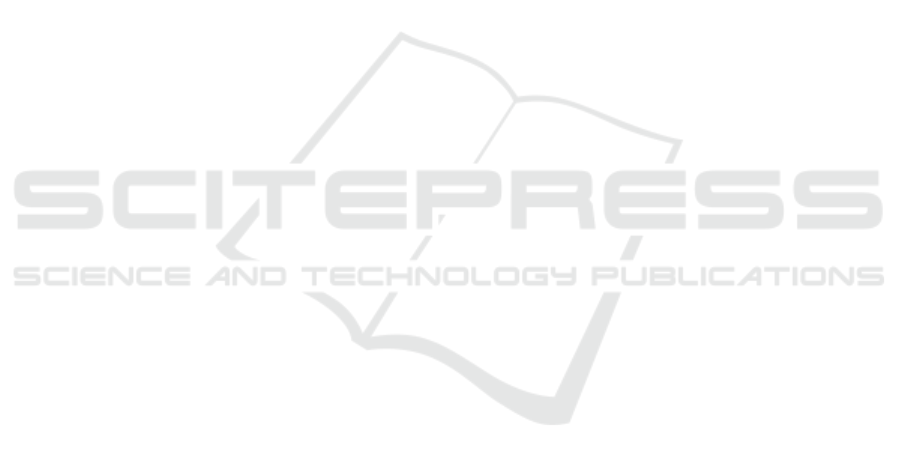
ACKNOWLEDGEMENTS
This work has been carried out within the French-
Canadian project DOMAID which is funded by the
National Agency for Research (ANR-20-CE26-0014-
01) and the FRQSC.
REFERENCES
Cao, X., Yao, J., Xu, Z., and Meng, D. (2020). Hyperspec-
tral image classification with convolutional neural net-
work and active learning. IEEE Transactions on Geo-
science and Remote Sensing, 58(7):4604–4616.
Delamare, M., Laville, C., Cabani, A., and Chafouk, H.
(2021). Graph convolutional networks skeleton-based
action recognition for continuous data stream: A slid-
ing window approach.
Du, Y., Wang, W., and Wang, L. (2015). Hierarchical recur-
rent neural network for skeleton based action recogni-
tion. In Proceedings of the IEEE Conference on Com-
puter Vision and Pattern Recognition (CVPR).
Duong, T., Phung, D., Bui, H., and Venkatesh, S. (2009).
Efficient duration and hierarchical modeling for hu-
man activity recognition. Artificial Intelligence,
173:830–856.
Ferguson, M., ak, R., Lee, Y.-T., and Law, K. (2017). Au-
tomatic localization of casting defects with convolu-
tional neural networks. pages 1726–1735.
Kulkarni, K., Evangelidis, G., Cech, J., and Horaud, R.
(2014). Continuous action recognition based on se-
quence alignment. International Journal of Computer
Vision, 112(1):90–114.
Laraba, S., Brahimi, M., Tilmanne, J., and Dutoit, T. (2017).
3d skeleton-based action recognition by representing
motion capture sequences as 2d-rgb images. Com-
puter Animation and Virtual Worlds, 28.
LeCun, Y., Bengio, Y., and Hinton, G. (2015). Deep learn-
ing. Nature, 521:436–44.
Li, Y., Lan, C., Xing, J., Zeng, W., Yuan, C., and
Liu, J. (2016). Online human action detection us-
ing joint classification-regression recurrent neural net-
works. volume 9911, pages 203–220.
Liu, J., Shahroudy, A., Wang, G., Duan, L.-Y., and Kot, A.
(2019). Skeleton-based online action prediction using
scale selection network. IEEE Transactions on Pattern
Analysis and Machine Intelligence, PP.
Liu, J., Shahroudy, A., Xu, D., Kot, A. C., and Wang,
G. (2017a). Skeleton-based action recognition using
spatio-temporal lstm network with trust gates.
Liu, J., Wang, G., Hu, P., Duan, L.-Y., and Kot, A. C.
(2017b). Global context-aware attention lstm net-
works for 3d action recognition. In 2017 IEEE Con-
ference on Computer Vision and Pattern Recognition
(CVPR), pages 3671–3680.
Liu, M., Liu, H., and Chen, C. (2017c). Enhanced skeleton
visualization for view invariant human action recogni-
tion. Pattern Recognition, 68:346–362.
Ludl, D., Gulde, T., and Curio, C. (2019). Simple yet effi-
cient real-time pose-based action recognition.
Manghisi, V. M., Uva, A. E., Fiorentino, M., Bevilacqua,
V., Trotta, G. F., and Monno, G. (2017). Real time
rula assessment using kinect v2 sensor. Applied Er-
gonomics, 65:481–491.
Martins, V., Kaleita, A., Gelder, B., Silveira, H., and Abe,
C. (2020). Exploring multiscale object-based convo-
lutional neural network (multi-ocnn) for remote sens-
ing image classification at high spatial resolution. IS-
PRS Journal of Photogrammetry and Remote Sensing,
168:56–73.
Mustaqeem and Kwon, S. (2020). Mlt-dnet: Speech emo-
tion recognition using 1d dilated cnn based on multi-
learning trick approach. Expert Systems with Applica-
tions, 167.
Pham, H.-H. (2019). Architectures d’apprentissage pro-
fond pour la reconnaissance d’actions humaines dans
des s
´
equences vid
´
eo rgb-d monoculaires: application
`
a la surveillance dans les transports publics. HAL
https://hal.inria.fr/hal-01678006.
Ronao, C. A. and Cho, S.-B. (2016). Human activity recog-
nition with smartphone sensors using deep learning
neural networks. Expert Systems with Applications,
59:235–244.
Simonyan, K. and Zisserman, A. (2015). Very deep convo-
lutional networks for large-scale image recognition.
Tang, C., Wang, P., and Li, W. (2017). Online action recog-
nition based on incremental learning of weighted co-
variance descriptors.
Wang, J., Chen, Y., Hao, S., Peng, X., and Hu, L. (2019).
Deep learning for sensor-based activity recognition: A
survey. Pattern Recognition Letters, 119:3–11.
Weng, J., Weng, C., and Yuan, J. (2017). Spatio-temporal
naive-bayes nearest-neighbor (st-nbnn) for skeleton-
based action recognition.
Yan, S., Xiong, Y., and Lin, D. (2018). Spatial temporal
graph convolutional networks for skeleton-based ac-
tion recognition.
Zhang, N., Wang, J., Wei, W., Qu, X., Cheng, N., and Xiao,
J. (2021). Cacnet: Cube attentional cnn for automatic
speech recognition. In 2021 International Joint Con-
ference on Neural Networks (IJCNN), pages 1–7.
Zhu, W., Lan, C., Xing, J., Zeng, W., Li, Y., Shen, L.,
and Xie, X. (2016). Co-occurrence feature learning
for skeleton based action recognition using regular-
ized deep lstm networks.
Human Activity Recognition: A Spatio-temporal Image Encoding of 3D Skeleton Data for Online Action Detection
455