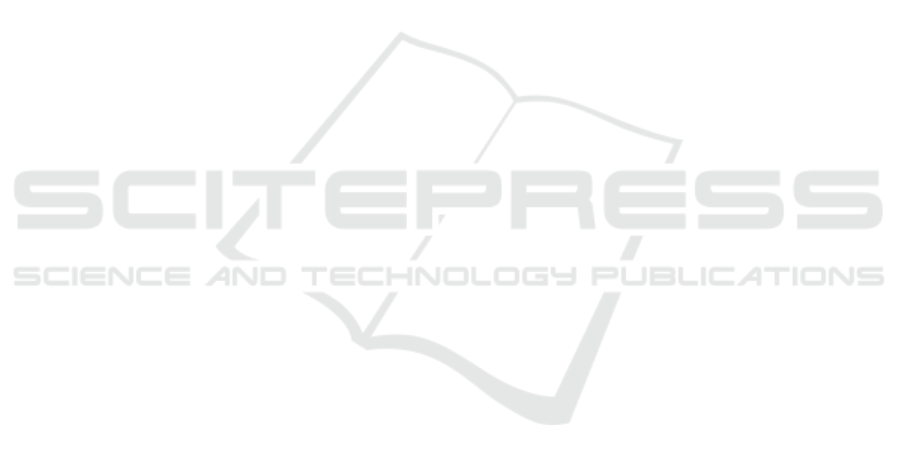
initiative between DfT and the Home Office (Ref:
007CD). This work is made possible by the WOS-
DETC dataset.
REFERENCES
Bergmann, P., Meinhardt, T., and Leal-Taixe, L. (2019).
Tracking without bells and whistles. In 2019
IEEE/CVF International Conference on Computer Vi-
sion, pages 941–951. IEEE.
Breckon, T. and Alsehaim, A. (2021). Not 3d re-id: Sim-
ple single stream 2d convolution for robust video re-
identification. In 2020 25th International Conference
on Pattern Recognition, pages 5190–5197.
Chang, X., Hospedales, T. M., and Xiang, T. (2018). Multi-
level factorisation net for person re-identification. In
Proceedings of the IEEE Conference on Computer Vi-
sion and Pattern Recognition, pages 2109–2118.
Cheng, D., Gong, Y., Zhou, S., Wang, J., and Zheng,
N. (2016). Person re-identification by multi-channel
parts-based cnn with improved triplet loss function.
In Proceedings of the IEEE conference on computer
vision and pattern recognition, pages 1335–1344.
Coluccia, A., Fascista, A., Schumann, A., Sommer, L.,
Ghenescu, M., Piatrik, T., De Cubber, G., Nalamati,
M., Kapoor, A., Saqib, M., et al. (2019). Drone-
vs-bird detection challenge at ieee avss2019. In
2019 16th IEEE International Conference on Ad-
vanced Video and Signal Based Surveillance, pages
1–7. IEEE.
Craye, C. and Ardjoune, S. (2019). Spatio-temporal seman-
tic segmentation for drone detection. In 2019 16th
IEEE International conference on advanced video and
signal based surveillance, pages 1–5. IEEE.
Deng, J., Khokhar, M. S., Aftab, M. U., Cai, J., Kumar,
R., Kumar, J., et al. (2021). Trends in vehicle re-
identification past, present, and future: A comprehen-
sive review. arXiv preprint arXiv:2102.09744.
Dosovitskiy, A., Beyer, L., Kolesnikov, A., Weissenborn,
D., Zhai, X., Unterthiner, T., Dehghani, M., Minderer,
M., Heigold, G., Gelly, S., Uszkoreit, J., and Houlsby,
N. (2021). An image is worth 16x16 words: Trans-
formers for image recognition at scale. In Interna-
tional Conference on Learning Representations.
Fu, Y., Wei, Y., Zhou, Y., Shi, H., Huang, G., Wang, X.,
Yao, Z., and Huang, T. (2019). Horizontal pyramid
matching for person re-identification. In Proceedings
of the AAAI Conference on Artificial Intelligence, vol-
ume 33, pages 8295–8302.
Gaszczak, A., Breckon, T., and Han, J. (2011). Real-time
people and vehicle detection from UAV imagery. In
Proc. SPIE Conference Intelligent Robots and Com-
puter Vision XXVIII: Algorithms and Techniques, vol-
ume 7878.
G
¨
okstorp, S. and Breckon, T. (2021). Temporal and
non-temporal contextual saliency analysis for general-
ized wide-area search within unmanned aerial vehicle
(uav) video. The Visual Computer. to appear.
Grigorev, A., Tian, Z., Rho, S., Xiong, J., Liu, S., and Jiang,
F. (2019). Deep person re-identification in UAV im-
ages. EURASIP Journal on Advances in Signal Pro-
cessing, 2019(1):54.
He, K., Zhang, X., Ren, S., and Sun, J. (2016). Deep resid-
ual learning for image recognition. In Proceedings of
the IEEE conference on computer vision and pattern
recognition, pages 770–778.
Hermans, A., Beyer, L., and Leibe, B. (2017). In defense
of the triplet loss for person re-identification. arXiv
preprint arXiv:1703.07737.
Hoffer, E. and Ailon, N. (2015). Deep metric learn-
ing using triplet network. In International workshop
on similarity-based pattern recognition, pages 84–92.
Springer.
Hu, J., Shen, L., and Sun, G. (2018). Squeeze-and-
excitation networks. In Proceedings of the IEEE con-
ference on computer vision and pattern recognition,
pages 7132–7141.
Ioffe, S. and Szegedy, C. (2015). Batch normalization: Ac-
celerating deep network training by reducing inter-
nal covariate shift. In Proceedings of the 32nd In-
ternational Conference on International Conference
on Machine Learning - Volume 37, ICML’15, page
448–456. JMLR.org.
Isaac-Medina, B. K. S., Poyser, M., Organisciak, D., Will-
cocks, C. G., Breckon, T. P., and Shum, H. P. H.
(2021). Unmanned aerial vehicle visual detection and
tracking using deep neural networks: A performance
benchmark.
Jiang, N., Wang, K., Peng, X., Yu, X., Wang, Q., Xing, J.,
Li, G., Zhao, J., Guo, G., and Han, Z. (2021). Anti-
UAV: A large multi-modal benchmark for UAV track-
ing.
Karanam, S., Gou, M., Wu, Z., Rates-Borras, A., Camps,
O., and Radke, R. J. (2019). A systematic evaluation
and benchmark for person re-identification: Features,
metrics, and datasets. IEEE Transactions on Pattern
Analysis and Machine Intelligence, 41(3):523–536.
Kuhn, H. (2012). The hungarian method for the assignment
problem. Naval Research Logistic Quarterly, 2.
Kuma, R., Weill, E., Aghdasi, F., and Sriram, P. (2019).
Vehicle re-identification: an efficient baseline using
triplet embedding. In 2019 International Joint Con-
ference on Neural Networks, pages 1–9. IEEE.
Li, W., Zhu, X., and Gong, S. (2018). Harmonious attention
network for person re-identification. In Proceedings of
the IEEE conference on computer vision and pattern
recognition, pages 2285–2294.
Li, Y., Fu, C., Ding, F., Huang, Z., and Lu, G. (2020). Auto-
track: Towards high-performance visual tracking for
UAV with automatic spatio-temporal regularization.
In Proceedings of the IEEE/CVF Conference on Com-
puter Vision and Pattern Recognition.
Liu, L., Ouyang, W., Wang, X., Fieguth, P., Chen, J., Liu,
X., and Pietik
¨
ainen, M. (2020a). Deep learning for
generic object detection: A survey. International jour-
nal of computer vision, 128(2):261–318.
Liu, M., Wang, X., Zhou, A., Fu, X., Ma, Y., and Piao,
C. (2020b). Uav-yolo: Small object detection on un-
UAV-ReID: A Benchmark on Unmanned Aerial Vehicle Re-identification in Video Imagery
145