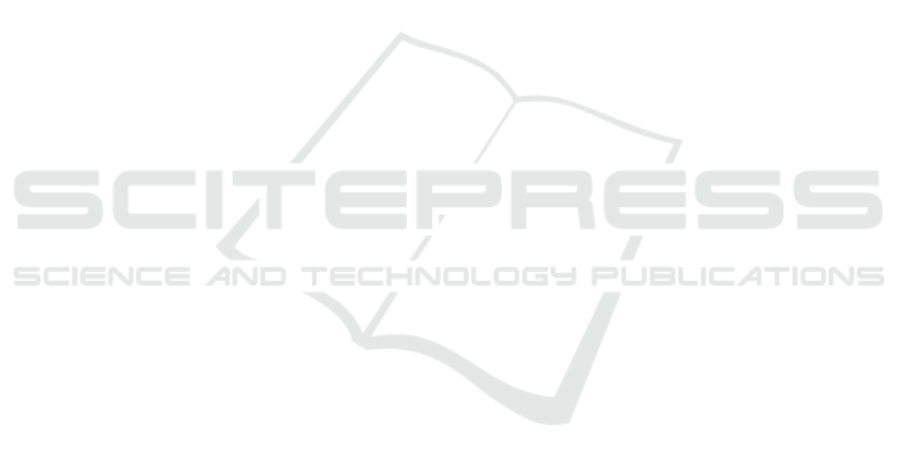
Transportation Systems, 17(10):2718–2729. Confer-
ence Name: IEEE Transactions on Intelligent Trans-
portation Systems.
Bhat, S., Naik, S., Gaonkar, M., Sawant, P., Aswale, S., and
Shetgaonkar, P. (2020). A Survey On Road Crack De-
tection Techniques. In 2020 International Conference
on Emerging Trends in Information Technology and
Engineering (ic-ETITE), pages 1–6.
Coquelle, E., Gautier, J.-L., and Dokl
´
adal, P. (2012). Au-
tomatic assessment of a road surface condition. In
7th Symposium on Pavement Surface Characteristics,
Surf, Norfolk, Virginia.
Duncan, C. (2018). noise. https://github.com/caseman/
noise.
Escalona, U., Arce, F., Zamora, E., and Sossa Azuela, J. H.
(2019). Fully Convolutional Networks for Automatic
Pavement Crack Segmentation. Computaci
´
on y Sis-
temas, 23(2):451–460–460. Number: 2.
Fang, J., Qu, B., and Yuan, Y. (2021). Distribution equal-
ization learning mechanism for road crack detection.
Neurocomputing, 424:193–204.
Fr
´
enay, B. and Verleysen, M. (2013). Classification in the
presence of label noise: a survey. IEEE transactions
on neural networks and learning systems, 25(5):845–
869.
Gao, Z., Peng, B., Li, T., and Gou, C. (2019). Genera-
tive Adversarial Networks for Road Crack Image Seg-
mentation. In 2019 International Joint Conference on
Neural Networks (IJCNN), pages 1–8. ISSN: 2161-
4407.
Kanaeva, I. and Ivanova, J. A. (2021). Road pavement crack
detection using deep learning with synthetic data. In
IOP Conference Series: Materials Science and Engi-
neering, volume 1019, page 012036. IOP Publishing.
Kim, B. and Cho, S. (2018). Automated Vision-Based De-
tection of Cracks on Concrete Surfaces Using a Deep
Learning Technique. Sensors, 18(10):3452. Number:
10 Publisher: Multidisciplinary Digital Publishing In-
stitute.
Liu, Z., Cao, Y., Wang, Y., and Wang, W. (2019). Com-
puter vision-based concrete crack detection using U-
net fully convolutional networks. Automation in Con-
struction, 104:129–139.
Mazzini, D., Napoletano, P., Piccoli, F., and Schettini, R.
(2020). A Novel Approach to Data Augmentation for
Pavement Distress Segmentation. Computers in In-
dustry, 121:103225.
Oliveira, H. and Correia, P. L. (2014). CrackIT — An im-
age processing toolbox for crack detection and char-
acterization. In 2014 IEEE International Conference
on Image Processing (ICIP), pages 798–802. ISSN:
2381-8549.
Pal, N. R. and Bhandari, D. (1993). Image thresholding:
Some new techniques. Signal Processing, 33(2):139–
158.
Perlin, K. (1985). An image synthesizer. ACM Siggraph
Computer Graphics, 19(3):287–296.
Ronneberger, O., Fischer, P., and Brox, T. (2015). U-Net:
Convolutional Networks for Biomedical Image Seg-
mentation.
Shi, Y., Cui, L., Qi, Z., Meng, F., and Chen, Z. (2016). Au-
tomatic Road Crack Detection Using Random Struc-
tured Forests. IEEE Transactions on Intelligent Trans-
portation Systems, 17(12):3434–3445. Conference
Name: IEEE Transactions on Intelligent Transporta-
tion Systems.
Simonyan, K. and Zisserman, A. (2015). Very Deep Con-
volutional Networks for Large-Scale Image Recogni-
tion. arXiv:1409.1556 [cs]. arXiv: 1409.1556.
Smirnov, N. V. (1939). On the estimation of the discrepancy
between empirical curves of distribution for two inde-
pendent samples. Bull. Math. Univ. Moscou, 2(2):3–
14.
Sun, M., Guo, R., Zhu, J., and Fan, W. (2020). Road-
way Crack Segmentation Based on an Encoder-
decoder Deep Network with Multi-scale Convolu-
tional Blocks. In 2020 10th Annual Computing and
Communication Workshop and Conference (CCWC),
pages 0869–0874.
Yang, F., Zhang, L., Yu, S., Prokhorov, D., Mei, X., and
Ling, H. (2020). Feature Pyramid and Hierarchi-
cal Boosting Network for Pavement Crack Detection.
IEEE Transactions on Intelligent Transportation Sys-
tems, 21(4):1525–1535. Conference Name: IEEE
Transactions on Intelligent Transportation Systems.
Yang, X., Li, H., Yu, Y., Luo, X., Huang, T., and
Yang, X. (2018). Automatic Pixel-Level Crack De-
tection and Measurement Using Fully Convolutional
Network. Computer-Aided Civil and Infrastruc-
ture Engineering, 33(12):1090–1109. https://online
library.wiley.com/doi/pdf/10.1111/mice.12412.
Zhang, H., Fritts, J. E., and Goldman, S. A. (2008). Image
segmentation evaluation: A survey of unsupervised
methods. Computer Vision and Image Understanding,
110(2):260–280.
Zhang, K., Zhang, Y., and Cheng, H.-D. (2020a). Crack-
GAN: Pavement Crack Detection Using Partially Ac-
curate Ground Truths Based on Generative Adversar-
ial Learning. IEEE Transactions on Intelligent Trans-
portation Systems, pages 1–14. Conference Name:
IEEE Transactions on Intelligent Transportation Sys-
tems.
Zhang, L., Shen, J., and Zhu, B. (2020b). A research
on an improved Unet-based concrete crack detec-
tion algorithm. Structural Health Monitoring, page
1475921720940068. Publisher: SAGE Publications.
Zhang, L., Yang, F., Daniel Zhang, Y., and Zhu, Y. J. (2016).
Road crack detection using deep convolutional neural
network. In 2016 IEEE International Conference on
Image Processing (ICIP), pages 3708–3712. ISSN:
2381-8549.
Zhou, Z.-H. (2017). A brief introduction to weakly super-
vised learning. National Science Review, 5(1):44–53.
Zou, Q., Cao, Y., Li, Q., Mao, Q., and Wang, S. (2012).
CrackTree: Automatic crack detection from pavement
images. Pattern Recognition Letters, 33(3):227–238.
VISAPP 2022 - 17th International Conference on Computer Vision Theory and Applications
158