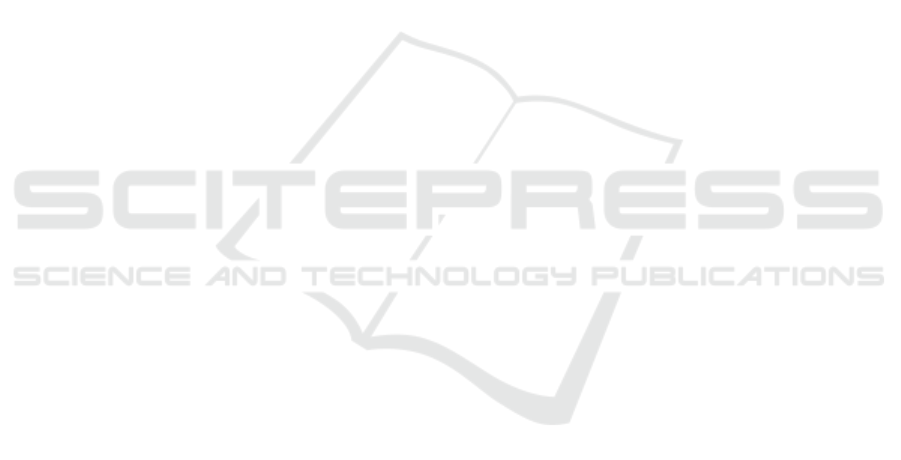
Girdhar, R. and Ramanan, D. (2020). CATER: A diagnos-
tic dataset for Compositional Actions and TEmporal
Reasoning. arXiv:1910.04744 [cs].
Goyal, P., Doll
´
ar, P., Girshick, R., Noordhuis, P.,
Wesolowski, L., Kyrola, A., Tulloch, A., Jia, Y.,
and He, K. (2017). Accurate, large minibatch
sgd: Training imagenet in 1 hour. arXiv preprint
arXiv:1706.02677.
Hara, K., Kataoka, H., and Satoh, Y. (2018). Can spa-
tiotemporal 3d cnns retrace the history of 2d cnns and
imagenet? In Proceedings of the IEEE conference
on Computer Vision and Pattern Recognition, pages
6546–6555.
He, D., Zhou, Z., Gan, C., Li, F., Liu, X., Li, Y., Wang, L.,
and Wen, S. (2019). Stnet: Local and global spatial-
temporal modeling for action recognition. In Proceed-
ings of the AAAI Conference on Artificial Intelligence,
volume 33, pages 8401–8408.
He, K., Zhang, X., Ren, S., and Sun, J. (2015). Delv-
ing deep into rectifiers: Surpassing human-level per-
formance on imagenet classification. In Proceedings
of the IEEE international conference on computer vi-
sion, pages 1026–1034.
He, Y., Shirakabe, S., Satoh, Y., and Kataoka, H. (2016).
Human action recognition without human. In Hua,
G. and J
´
egou, H., editors, Computer Vision – ECCV
2016 Workshops, pages 11–17, Cham. Springer Inter-
national Publishing.
Hinton, G. E., Srivastava, N., Krizhevsky, A., Sutskever, I.,
and Salakhutdinov, R. R. (2012). Improving neural
networks by preventing co-adaptation of feature de-
tectors. arXiv preprint arXiv:1207.0580.
Holtgraves, T. and Srull, T. K. (1990). Ordered and un-
ordered retrieval strategies in person memory. Journal
of Experimental Social Psychology, 26(1):63–81.
Horn, B. K. and Schunck, B. G. (1981). Determining optical
flow. Artificial intelligence, 17(1-3):185–203.
Hu, H., Gu, J., Zhang, Z., Dai, J., and Wei, Y.
(2018). Relation Networks for Object Detection.
arXiv:1711.11575 [cs].
Hutchinson, M. and Gadepally, V. (2020). Video Action
Understanding: A Tutorial. arXiv:2010.06647 [cs].
Jain, M., van Gemert, J., Snoek, C. G., et al. (2014). Univer-
sity of amsterdam at thumos challenge 2014. ECCV
THUMOS Challenge, 2014.
Ji, S., Xu, W., Yang, M., and Yu, K. (2012). 3d convolu-
tional neural networks for human action recognition.
IEEE transactions on pattern analysis and machine
intelligence, 35(1):221–231.
Jiang, B., Wang, M., Gan, W., Wu, W., and Yan, J. (2019).
Stm: Spatiotemporal and motion encoding for action
recognition. In Proceedings of the IEEE/CVF Interna-
tional Conference on Computer Vision, pages 2000–
2009.
Johnson, J., Hariharan, B., van der Maaten, L., Fei-Fei, L.,
Lawrence Zitnick, C., and Girshick, R. (2017). Clevr:
A diagnostic dataset for compositional language and
elementary visual reasoning. In Proceedings of the
IEEE Conference on Computer Vision and Pattern
Recognition (CVPR).
Karpathy, A., Toderici, G., Shetty, S., Leung, T., Suk-
thankar, R., and Fei-Fei, L. (2014a). Large-scale video
classification with convolutional neural networks. In
Proceedings of the IEEE conference on Computer Vi-
sion and Pattern Recognition, pages 1725–1732.
Karpathy, A., Toderici, G., Shetty, S., Leung, T., Suk-
thankar, R., and Fei-Fei, L. (2014b). Large-scale
video classification with convolutional neural net-
works. In CVPR.
Kuehne, H., Jhuang, H., Garrote, E., Poggio, T., and Serre,
T. (2011). HMDB: a large video database for human
motion recognition. In Proceedings of the Interna-
tional Conference on Computer Vision (ICCV).
Lan, Z., Lin, M., Li, X., Hauptmann, A. G., and Raj, B.
(2015). Beyond gaussian pyramid: Multi-skip fea-
ture stacking for action recognition. In Proceedings
of the IEEE conference on computer vision and pat-
tern recognition, pages 204–212.
Levi, H. and Ullman, S. (2018). Efficient coarse-to-fine
non-local module for the detection of small objects.
arXiv preprint arXiv:1811.12152.
Luo, C. and Yuille, A. L. (2019). Grouped spatial-temporal
aggregation for efficient action recognition. In Pro-
ceedings of the IEEE/CVF International Conference
on Computer Vision, pages 5512–5521.
Peng, X., Zou, C., Qiao, Y., and Peng, Q. (2014). Ac-
tion recognition with stacked fisher vectors. In Euro-
pean Conference on Computer Vision, pages 581–595.
Springer.
Ren, S., He, K., Girshick, R., and Sun, J. (2015). Faster
r-cnn: Towards real-time object detection with region
proposal networks. Advances in neural information
processing systems, 28:91–99.
Rezatofighi, S. H., BG, V. K., Milan, A., Abbasnejad, E.,
Dick, A., and Reid, I. (2017). Deepsetnet: Predicting
sets with deep neural networks. In 2017 IEEE Interna-
tional Conference on Computer Vision (ICCV), pages
5257–5266. IEEE.
Santoro, A., Raposo, D., Barrett, D. G. T., Malinowski, M.,
Pascanu, R., Battaglia, P., and Lillicrap, T. (2017). A
simple neural network module for relational reason-
ing. arXiv:1706.01427 [cs].
Shamsian, A., Kleinfeld, O., Globerson, A., and Chechik,
G. (2020). Learning object permanence from video.
In European Conference on Computer Vision, pages
35–50. Springer.
Shanahan, M., Nikiforou, K., Creswell, A., Kaplanis,
C., Barrett, D., and Garnelo, M. (2020). An
Explicitly Relational Neural Network Architecture.
arXiv:1905.10307 [cs, stat].
Shoham, Y. (1987). Reasoning about change: time and
causation from the standpoint of artificial intelligence.
PhD thesis, Yale University.
Simonyan, K. and Zisserman, A. (2014). Two-stream con-
volutional networks for action recognition in videos.
arXiv preprint arXiv:1406.2199.
Soomro, K., Zamir, A. R., and Shah, M. (2012). Ucf101:
A dataset of 101 human actions classes from videos in
the wild. arXiv preprint arXiv:1212.0402.
Deep Set Conditioned Latent Representations for Action Recognition
465