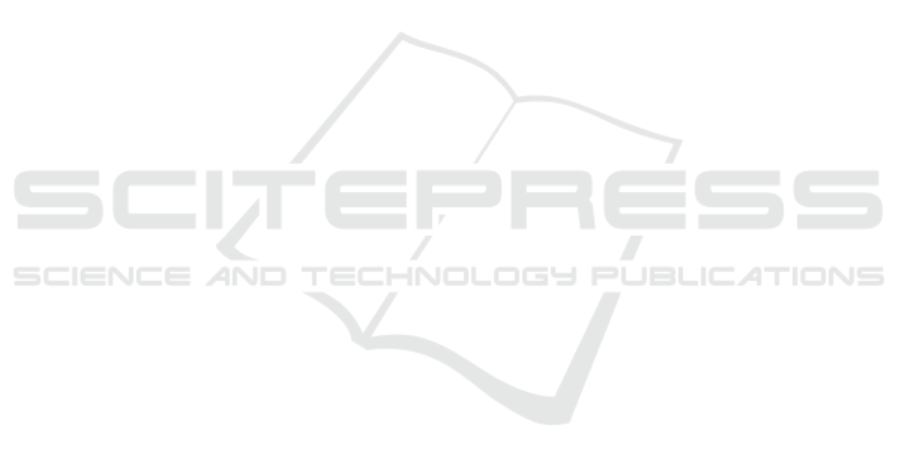
5 CONCLUSIONS
In this paper, we propose an end-to-end multi-task
network for semantic segmentation and depth com-
pletion. It combines a modified version of two
bench-marking models, more specifically, we used
the model proposed by Chen et al. (2020) for depth
completion and our semantic segmentation branch is
based on the semantic segmentation branch of the
EfficientPS model proposed by Mohan and Valada
(2020).
With the proposed model, we successfully pro-
vide further evidence that multi-task networks can
significantly improve the performance of each indi-
vidual task by learning features jointly. Our model
successfully predicts the fully dense depth map as
well as the semantic segmentation image in a scene,
given an RGB image and a sparse depth image as in-
puts to our model. In addition to that, our ablation
studies demonstrate quantitatively, that our multi-task
network outperforms, by a large margin, equivalent
single-task networks.
REFERENCES
Cabon, Y., Murray, N., and Humenberger, M. (2020). Vir-
tual kitti 2.
Chen, L., Papandreou, G., Kokkinos, I., Murphy, K.,
and Yuille, A. L. (2016). Deeplab: Semantic
image segmentation with deep convolutional nets,
atrous convolution, and fully connected crfs. CoRR,
abs/1606.00915.
Chen, L., Zhu, Y., Papandreou, G., Schroff, F., and Adam,
H. (2018a). Encoder-decoder with atrous separable
convolution for semantic image segmentation. CoRR,
abs/1802.02611.
Chen, L.-C., Collins, M. D., Zhu, Y., Papandreou, G., Zoph,
B., Schroff, F., Adam, H., and Shlens, J. (2018b).
Searching for efficient multi-scale architectures for
dense image prediction.
Chen, Y., Yang, B., Liang, M., and Urtasun, R. (2020).
Learning joint 2d-3d representations for depth com-
pletion.
Cheng, B., Collins, M. D., Zhu, Y., Liu, T., Huang, T. S.,
Adam, H., and Chen, L.-C. (2020). Panoptic-deeplab:
A simple, strong, and fast baseline for bottom-up
panoptic segmentation.
Chollet, F. (2016). Xception: Deep learning with depthwise
separable convolutions. CoRR, abs/1610.02357.
Dauphin, Y. and Grangier, D. (2015). Predicting dis-
tributions with linearizing belief networks. CoRR,
abs/1511.05622.
Dauphin, Y. N., Fan, A., Auli, M., and Grangier, D.
(2016). Language modeling with gated convolutional
networks. CoRR, abs/1612.08083.
Eigen, D. and Fergus, R. (2014). Predicting depth,
surface normals and semantic labels with a com-
mon multi-scale convolutional architecture. CoRR,
abs/1411.4734.
Godard, C., Aodha, O. M., Firman, M., and Brostow, G.
(2019). Digging into self-supervised monocular depth
estimation.
Guizilini, V., Ambrus, R., Pillai, S., Raventos, A., and
Gaidon, A. (2020). 3d packing for self-supervised
monocular depth estimation.
Hazirbas, C., Ma, L., Domokos, C., and Cremers, D.
(2016). Fusenet: Incorporating depth into semantic
segmentation via fusion-based cnn architecture. In
Asian Conference on Computer Vision (ACCV).
He, K., Zhang, X., Ren, S., and Sun, J. (2015). Deep resid-
ual learning for image recognition.
He, L., Lu, J., Wang, G., Song, S., and Zhou, J. (2021).
Sosd-net: Joint semantic object segmentation and
depth estimation from monocular images. CoRR,
abs/2101.07422.
Huang, Z., Fan, J., Cheng, S., Yi, S., Wang, X., and Li, H.
(2020). Hms-net: Hierarchical multi-scale sparsity-
invariant network for sparse depth completion.
Imran, S., Long, Y., Liu, X., and Morris, D. (2019). Depth
coefficients for depth completion.
Kendall, A., Gal, Y., and Cipolla, R. (2017). Multi-task
learning using uncertainty to weigh losses for scene
geometry and semantics. CoRR, abs/1705.07115.
Kim, D., Woo, S., Lee, J.-Y., and Kweon, I. S. (2020).
Video panoptic segmentation.
Kreso, I., Causevic, D., Krapac, J., and Segvic, S. (2016).
Convolutional scale invariance for semantic segmen-
tation. In GCPR.
Liebel, L. and K
¨
orner, M. (2018). Auxiliary tasks in multi-
task learning.
Lin, G., Milan, A., Shen, C., and Reid, I. (2016). Refinenet:
Multi-path refinement networks for high-resolution
semantic segmentation.
Lin, T.-Y., Doll
´
ar, P., Girshick, R., He, K., Hariharan, B.,
and Belongie, S. (2017). Feature pyramid networks
for object detection.
Long, J., Shelhamer, E., and Darrell, T. (2014). Fully
convolutional networks for semantic segmentation.
CoRR, abs/1411.4038.
Ma, F., Cavalheiro, G. V., and Karaman, S. (2018). Self-
supervised sparse-to-dense: Self-supervised depth
completion from lidar and monocular camera.
Mohan, R. and Valada, A. (2020). Efficientps: Efficient
panoptic segmentation. CoRR, abs/2004.02307.
Qiu, J., Cui, Z., Zhang, Y., Zhang, X., Liu, S., Zeng,
B., and Pollefeys, M. (2018). Deeplidar: Deep sur-
face normal guided depth prediction for outdoor scene
from sparse lidar data and single color image. CoRR,
abs/1812.00488.
Redmon, J. and Farhadi, A. (2018). Yolov3: An incremental
improvement. CoRR, abs/1804.02767.
Ren, S., He, K., Girshick, R., and Sun, J. (2016). Faster
r-cnn: Towards real-time object detection with region
proposal networks.
VISAPP 2022 - 17th International Conference on Computer Vision Theory and Applications
162