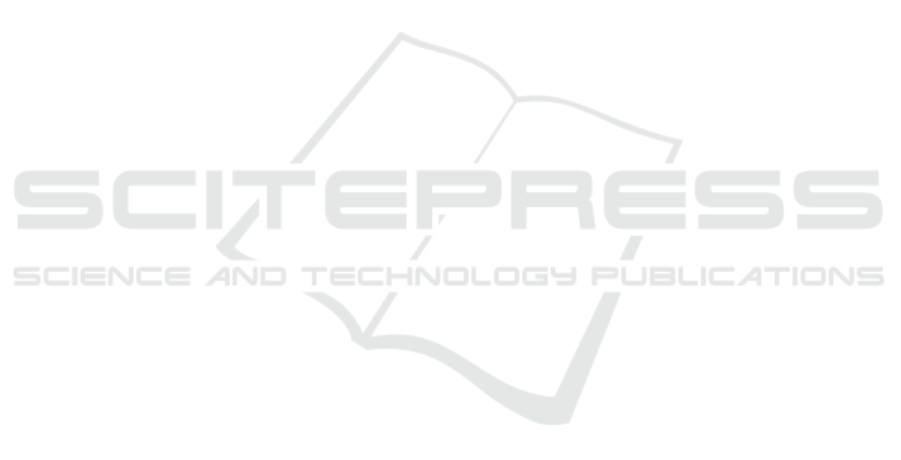
computational performance. Results show that by
using audio as the first processing block, it was
possible to obtain an accuracy score higher than the
state-of-the-art, along with a significant reduction in
processing/inference time.
The obtained results are interesting and reveal a
high potential for further improvement. Modifica-
tions to the individual processing sub-modules could
contribute to even higher accuracies while further re-
ducing computational weight. The latter may benefit
from a combination with model compression and ac-
celeration techniques, such as quantisation, avoiding
likely losses in accuracy due to compression.
The proposed strategy demonstrated benefits from
cascading the processing modules. Other early mod-
ules may bring other benefits by filtering out incom-
ing audio-visual data, without relevant content (e. g.,
without people present or without movement/sound).
ACKNOWLEDGEMENTS
This work was supported by: European Struc-
tural and Investment Funds in the FEDER com-
ponent, through the Operational Competitiveness
and Internationalization Programme (COMPETE
2020) [Project no. 039334; Funding Reference:
POCI-01-0247-FEDER-039334], by National Funds
through the Portuguese funding agency, FCT -
Fundac¸
˜
ao para a Ci
ˆ
encia e a Tecnologia within
project UIDB/50014/2020, and within PhD grants
“SFRH/BD/137720/2018” and “2021.06945.BD”.
The authors wish to thank the authors of the MMIT
database and pretrained models.
REFERENCES
Augusto, P., Cardoso, J. S., and Fonseca, J. (2020). Au-
tomotive interior sensing - towards a synergetic ap-
proach between anomaly detection and action recog-
nition strategies. In Fourth IEEE International Con-
ference on Image Processing, Applications and Sys-
tems (IPAS 2020), pages 162–167, Genova, Italy.
Carreira, J. and Zisserman, A. (2017). Quo vadis, action
recognition? a new model and the kinetics dataset. In
2017 IEEE Conference on Computer Vision and Pat-
tern Recognition (CVPR), pages 4724–4733. IEEE.
Cosbey, R., Wusterbarth, A., and Hutchinson, B. (2019).
Deep Learning for Classroom Activity Detection from
Audio. In ICASSP 2019 - 2019 IEEE International
Conference on Acoustics, Speech and Signal Process-
ing (ICASSP), pages 3727–3731. IEEE.
Feichtenhofer, C., Fan, H., Malik, J., and He, K. (2019).
SlowFast Networks for Video Recognition. In 2019
IEEE/CVF International Conference on Computer Vi-
sion (ICCV), pages 6201–6210.
He, K., Zhang, X., Ren, S., and Sun, J. (2016). Deep resid-
ual learning for image recognition. In 2016 IEEE Con-
ference on Computer Vision and Pattern Recognition
(CVPR), pages 770–778.
Heilbron, F. C., Escorcia, V., Ghanem, B., and Niebles, J. C.
(2015). ActivityNet: A large-scale video benchmark
for human activity understanding. In 2015 IEEE Con-
ference on Computer Vision and Pattern Recognition
(CVPR), pages 961–970.
Hu, J. F., Zheng, W. S., Ma, L., Wang, G., Lai, J., and
Zhang, J. (2018). Early action prediction by soft re-
gression. IEEE Transactions on Pattern Analysis and
Machine Intelligence, 41(11):2568–2583.
Kazakos, E., Nagrani, A., Zisserman, A., and Damen,
D. (2019). EPIC-Fusion: Audio-Visual Temporal
Binding for Egocentric Action Recognition. In 2019
IEEE/CVF International Conference on Computer Vi-
sion (ICCV), pages 5491–5500.
Kong, Y., Tao, Z., and Fu, Y. (2017). Deep sequential con-
text networks for action prediction. In 2017 IEEE
Conference on Computer Vision and Pattern Recog-
nition (CVPR), pages 3662–3670.
Liang, D. and Thomaz, E. (2019). Audio-based activities of
daily living (ADL) recognition with large-scale acous-
tic embeddings from online videos. Proc. ACM Inter-
act. Mob. Wearable Ubiquitous Technol., 3(1).
Mirsamadi, S., Barsoum, E., and Zhang, C. (2017). Auto-
matic speech emotion recognition using recurrent neu-
ral networks with local attention. In 2017 IEEE Inter-
national Conference on Acoustics, Speech and Signal
Processing (ICASSP), pages 2227–2231. IEEE.
Monfort, M., Ramakrishnan, K., Andonian, A., McNamara,
B. A., Lascelles, A., Pan, B., Fan, Q., Gutfreund,
D., Feris, R., and Oliva, A. (2019). Multi-moments
in time: Learning and interpreting models for multi-
action video understanding.
Pang, G., Wang, X., Hu, J.-F., Zhang, Q., and Zheng, W.-
S. (2019). DBDNet: Learning Bi-directional Dynam-
ics for Early Action Prediction. In Proceedings of the
Twenty-Eighth International Joint Conference on Ar-
tificial Intelligence, IJCAI-19, pages 897–903.
Pinto, J. R., Gonc¸alves, T., Pinto, C., Sanhudo, L., Fon-
seca, J., Gonc¸alves, F., Carvalho, P., and Cardoso, J. S.
(2020). Audiovisual classification of group emotion
valence using activity recognition networks. In 2020
IEEE 4th International Conference on Image Process-
ing, Applications and Systems (IPAS), pages 114–119.
Qi, M., Wang, Y., Qin, J., Li, A., Luo, J., and Van Gool,
L. (2020). stagNet: An Attentive Semantic RNN for
Group Activity and Individual Action Recognition.
IEEE Transactions on Circuits and Systems for Video
Technology, 30(2):549–565.
VISAPP 2022 - 17th International Conference on Computer Vision Theory and Applications
474