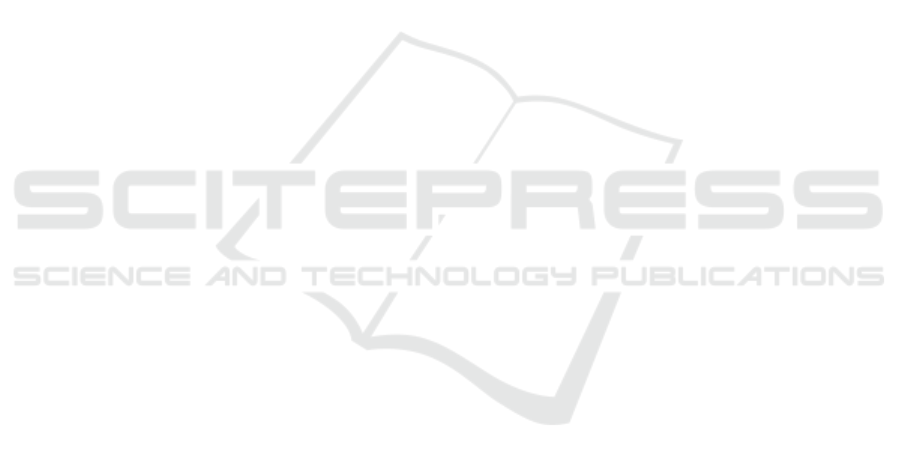
Knopf, A. (2020). Autism prevalence increases from 1 in
60 to 1 in 54: Cdc. The Brown University Child and
Adolescent Behavior Letter, 36(6):4–4.
Lea, C., Vidal, R., Reiter, A., and Hager, G. D. (2016).
Temporal convolutional networks: A unified approach
to action segmentation. In European Conference on
Computer Vision, pages 47–54. Springer.
Lewis, M. H. and Bodfish, J. W. (1998). Repetitive behavior
disorders in autism. Mental retardation and develop-
mental disabilities research reviews, 4(2):80–89.
Li, B., Mehta, S., Aneja, D., Foster, C., Ventola, P., Shic, F.,
and Shapiro, L. (2019). A facial affect analysis system
for autism spectrum disorder. In 2019 IEEE Interna-
tional Conference on Image Processing (ICIP), pages
4549–4553. IEEE.
Li, J., Zhong, Y., Han, J., Ouyang, G., Li, X., and Liu, H.
(2020). Classifying asd children with lstm based on
raw videos. Neurocomputing, 390:226–238.
Li, J., Zhong, Y., and Ouyang, G. (2018). Identification of
asd children based on video data. In 2018 24th In-
ternational conference on pattern recognition (ICPR),
pages 367–372. IEEE.
Liu, W., Li, M., and Yi, L. (2016). Identifying children
with autism spectrum disorder based on their face pro-
cessing abnormality: A machine learning framework.
Autism Research, 9(8):888–898.
Liu, W., Zhou, T., Zhang, C., Zou, X., and Li, M. (2017).
Response to name: A dataset and a multimodal ma-
chine learning framework towards autism study. In
2017 Seventh International Conference on Affective
Computing and Intelligent Interaction (ACII), pages
178–183. IEEE.
Lord, C., Risi, S., Lambrecht, L., Cook, E. H., Leven-
thal, B. L., DiLavore, P. C., Pickles, A., and Rutter,
M. (2000). The autism diagnostic observation sched-
ule—generic: A standard measure of social and com-
munication deficits associated with the spectrum of
autism. Journal of autism and developmental disor-
ders, 30(3):205–223.
Ma, S., Sigal, L., and Sclaroff, S. (2016). Learning activity
progression in lstms for activity detection and early
detection. In Proceedings of the IEEE conference on
computer vision and pattern recognition, pages 1942–
1950.
Marinoiu, E., Zanfir, M., Olaru, V., and Sminchisescu,
C. (2018). 3d human sensing, action and emotion
recognition in robot assisted therapy of children with
autism. In Proceedings of the IEEE conference on
computer vision and pattern recognition, pages 2158–
2167.
Milan, A., Leal-Taix
´
e, L., Reid, I., Roth, S., and Schindler,
K. (2016). Mot16: A benchmark for multi-object
tracking. arXiv preprint arXiv:1603.00831.
Negin, F., Ozyer, B., Agahian, S., Kacdioglu, S., and Ozyer,
G. T. (2021). Vision-assisted recognition of stereotype
behaviors for early diagnosis of autism spectrum dis-
orders. Neurocomputing, 446:145–155.
O’Roak, B. J. and State, M. W. (2008). Autism genetics:
strategies, challenges, and opportunities. Autism Re-
search, 1(1):4–17.
Pandey, P., Prathosh, A., Kohli, M., and Pritchard, J. (2020).
Guided weak supervision for action recognition with
scarce data to assess skills of children with autism.
In Proceedings of the AAAI Conference on Artificial
Intelligence, volume 34, pages 463–470.
Rajagopalan, S., Dhall, A., and Goecke, R. (2013). Self-
stimulatory behaviours in the wild for autism diagno-
sis. In Proceedings of the IEEE International Confer-
ence on Computer Vision Workshops, pages 755–761.
Redmon, J. and Farhadi, A. (2018). Yolov3: An incremental
improvement. arXiv preprint arXiv:1804.02767.
Rehg, J., Abowd, G., Rozga, A., Romero, M., Clements,
M., Sclaroff, S., Essa, I., Ousley, O., Li, Y., Kim, C.,
et al. (2013). Decoding children’s social behavior. In
Proceedings of the IEEE conference on computer vi-
sion and pattern recognition, pages 3414–3421.
Shao, S., Zhao, Z., Li, B., Xiao, T., Yu, G., Zhang,
X., and Sun, J. (2018). Crowdhuman: A bench-
mark for detecting human in a crowd. arXiv preprint
arXiv:1805.00123.
Simonyan, K. and Zisserman, A. (2014). Two-stream con-
volutional networks for action recognition in videos.
arXiv preprint arXiv:1406.2199.
Tanaka, J. W. and Sung, A. (2016). The “eye avoidance” hy-
pothesis of autism face processing. Journal of autism
and developmental disorders, 46(5):1538–1552.
Teed, Z. and Deng, J. (2020). Raft: Recurrent all-pairs field
transforms for optical flow. In European conference
on computer vision, pages 402–419. Springer.
Tian, Y., Min, X., Zhai, G., and Gao, Z. (2019). Video-
based early asd detection via temporal pyramid net-
works. In 2019 IEEE International Conference on
Multimedia and Expo (ICME), pages 272–277. IEEE.
Tran, D., Bourdev, L., Fergus, R., Torresani, L., and Paluri,
M. (2015). Learning spatiotemporal features with 3d
convolutional networks. In Proceedings of the IEEE
international conference on computer vision, pages
4489–4497.
Tran, D., Wang, H., Torresani, L., and Feiszli, M. (2019).
Video classification with channel-separated convolu-
tional networks. In Proceedings of the IEEE/CVF
International Conference on Computer Vision, pages
5552–5561.
Wang, L., Xiong, Y., Wang, Z., and Qiao, Y. (2015a). To-
wards good practices for very deep two-stream con-
vnets. arXiv preprint arXiv:1507.02159.
Wang, S., Jiang, M., Duchesne, X. M., Laugeson, E. A.,
Kennedy, D. P., Adolphs, R., and Zhao, Q. (2015b).
Atypical visual saliency in autism spectrum disorder
quantified through model-based eye tracking. Neuron,
88(3):604–616.
Wojke, N., Bewley, A., and Paulus, D. (2017). Simple on-
line and realtime tracking with a deep association met-
ric. In 2017 IEEE international conference on image
processing (ICIP), pages 3645–3649. IEEE.
Zhang, Y., Tian, Y., Wu, P., and Chen, D. (2021). Applica-
tion of skeleton data and long short-term memory in
action recognition of children with autism spectrum
disorder. Sensors, 21(2):411.
Video-based Behavior Understanding of Children for Objective Diagnosis of Autism
483