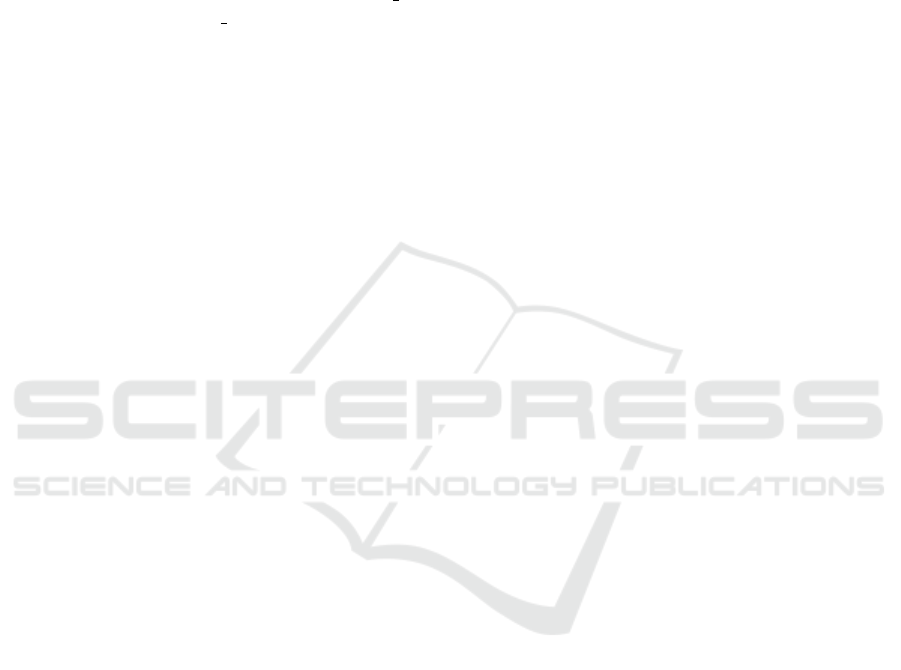
5 CONCLUSIONS
We can now answer the research questions raised in
section3.
• What insights can be obtained from exploratory
data analysis?: The exploratory analysis of the
data shows us a higher predictive value of the in-
ternal performance variables. The dependent vari-
able has a stronger correlation with waiting bed
than visits, and census hosp and visits have the
same strength. This fact verifies our hypothesis
about the higher influence of the hospital perfor-
mance compared to external demand. Other vari-
ables are stronger correlated, especially patient ty-
pologies and critical levels. We notice that car-
diovascular and respiratory variables are stronger
correlated than other categories, we also notice
that level3 is more correlated than other more crit-
ical levels.
• Can we predict the ED occupation without us-
ing the patient demand? Although the influence
of hospital performance is very important, we
achieved better results by characterizing all fac-
tors related to overcrowding, both external and in-
ternal.
• Can we obtain better results using internal hos-
pital performance variables? Definitely, incorpo-
rating the proposed variables improves the results
of the ED overcrowding forecasting models.This
has been confirmed with the ablation study where
these variables were eliminated and the perfor-
mance of the estimation decreased.
All the research objectives have been reached, the
results show that ED occupation can be predicted
from internal performance variables, even excluding
external demand. A better understanding of the corre-
lation of internal hospital performance variables with
overcrowding in emergency departments has been
reached.
The linear regression models used in the experi-
ments are powerful enough to yield good performance
but not so much complex to mask the influence of the
variables in the results. Thus, they allow us to estab-
lish a baseline for future works to explore the use of
different machine learning models like artificial neu-
ral networks and decisions trees. Another possible fu-
ture work is to explore the use of exogenous variables.
REFERENCES
Akoglu, H. (2018). User’s guide to correlation coefficients.
Turkish Journal of Emergency Medicine, 18(3):91–93.
Araz, O., Bentley, D., and Muelleman, R. (2014). Us-
ing google flu trends data in forecasting influenza-
like–illness related emergency department visits in
omaha, nebraska. The American Journal of Emer-
gency Medicine, 32.
Asplin, B. R., Magid, D. J., Rhodes, K. V., Solberg, L. I.,
Lurie, N., and Camargo, C. A. (2003). A conceptual
model of emergency department crowding. Annals of
Emergency Medicine, 42(2):173–180.
Box, G. E. P. and Cox, D. R. (1964). An analysis of trans-
formations. Journal of the Royal Statistical Society.
Series B (Methodological), 26:211–252.
Boyle, J., Jessup, M., Crilly, J., Green, D., Lind, J., Wal-
lis, M., Miller, P., and Fitzgerald, G. (2011). Predict-
ing emergency department admissions. Emergency
medicine journal : EMJ, 29:358–65.
Calegari, R., Fogliatto, F., Lucini, F., Neyeloff, J., Kuchen-
becker, R., and Schaan, B. (2016). Forecasting daily
volume and acuity of patients in the emergency de-
partment. Computational and Mathematical Methods
in Medicine, 2016:1–8.
Derlet, R. W. and Richards, J. R. (2000). Overcrowd-
ing in the nation’s emergency departments: Complex
causes and disturbing effects. Annals of Emergency
Medicine, 35:63–68.
Dickey, D. and Fuller, W. (1979). Distribution of the esti-
mators for autoregressive time series with a unit root.
JASA. Journal of the American Statistical Association,
74.
Division, P. (2019). World population ageing: highlights.
New York : UN, 2019, page 37.
G
¨
ul, M. and Celik, E. (2018). An exhaustive review and
analysis on applications of statistical forecasting in
hospital emergency departments. Health System.
Gul, M. and Guneri, A. (2016). Planning the future of emer-
gency departments: Forecasting ed patient arrivals by
using regression and neural network models. Inter-
national Journal of Industrial Engineering: Theory,
Applications and Practice, 23:137–154.
Hamilton, J. (1994). Time series analysis. Princeton Univ.
Press, Princeton, NJ.
Hertzum, M. (2017). Forecasting hourly patient visits in the
emergency department to counteract crowding. The
Ergonomics Open Journal.
Hirshon, J., Risko, N., Calvello, E., Ramirez, S., Narayan,
M., Theodosis, C., and O’Neill, J. (2013). Health sys-
tems and services: The role of acute care. Bulletin of
the World Health Organization, 91:386–388.
Hoot, N. and Aronsky, D. (2008). Systematic review of
emergency department crowding: Causes, effects, and
solutions. Annals of emergency medicine, 52:126–36.
Islam, M. S., Hasan, M. M., Wang, X., Noor-E-Alam, M.,
and Germack, H. (2018). A systematic review on
healthcare analytics: Application and theoretical per-
spective of data mining. Healthcare, 6.
Jones, S., Thomas, A., Evans, R. S., Welch, S., Haug, P.,
and Snow, G. (2008). Forecasting daily patient vol-
umes in the emergency department. Academic emer-
gency medicine : official journal of the Society for
Academic Emergency Medicine, 15:159–70.
HEALTHINF 2022 - 15th International Conference on Health Informatics
512