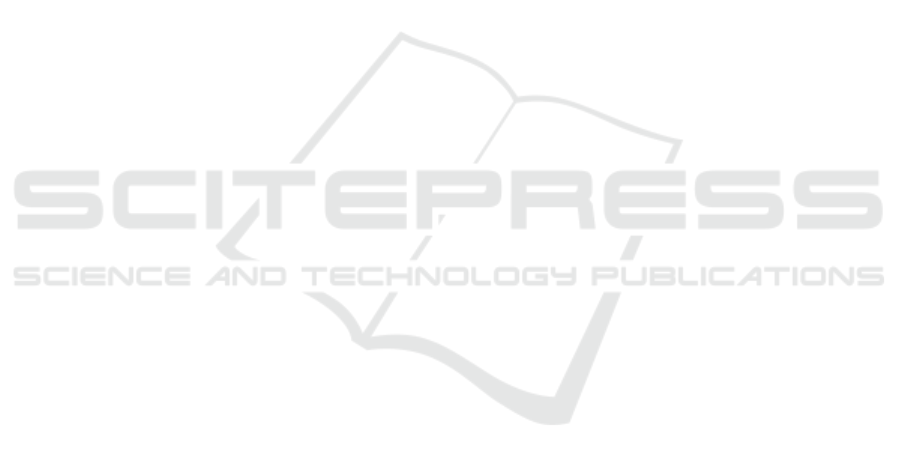
lies on composite material-constructed wind turbine
blades using drone imagery. BladeNet utilises an
instance-segmentation method of blade extraction
which is far more precise at fitting the blade edges
than conventional object detection models both qual-
ititively and quantitatively obtaining a Average Pre-
cision (AP) of 0.995 together with a suite of semi-
supervised generative anomaly detection methods
across extracted SLIC super-pixel blade parts to de-
tect anomalies with an AUC of 0.639. We hope that
this work can aid engineers and wind farm inspectors
to detect surface faults of composite wind turbines.
ACKNOWLEDGEMENTS
Thank you to EPSRC and Ørsted for funding support
towards this work.
REFERENCES
Achanta, R., Shaji, A., Smith, K., Lucchi, A., Fua, P., and
S
¨
usstrunk, S. (2012). Slic superpixels compared to
state-of-the-art superpixel methods. IEEE Transac-
tions on Pattern Analysis and Machine Intelligence,
34(11):2274–2282.
Akcay, S., Atapour-Abarghouei, A., and Breckon, T.
(2019a). Skip-ganomaly: Skip connected and adver-
sarially trained encoder-decoder anomaly detection.
Proceedings of the International Joint Conference on
Neural Networks, July.
Akcay, S., Atapour-Abarghouei, A., and Breckon, T. P.
(2019b). Ganomaly : semi-supervised anomaly detec-
tion via adversarial training. In 14th Asian Conference
on Computer Vision, number 11363 in Lecture notes
in computer science, pages 622–637. Springer.
Anne Juengert, C. U. G. (2009). Inspection techniques
for wind turbine blades using ultrasound and sound
waves. In Non-Destructive Testing in Civil Engineer-
ing.
Archer, C. L. and Jacobson, M. Z. (2005). Evaluation of
global wind power. Journal of Geophysical Research:
Atmospheres, 110(D12).
Barker, J. W. and Breckon, T. P. (2021). Panda: Perceptually
aware neural detection of anomalies. In International
Joint Conference on Neural Networks, IJCNN.
Baur, C., Wiestler, B., Albarqouni, S., and Navab, N.
(2018). Deep Autoencoding Models for Unsupervised
Anomaly Segmentation in Brain MR Images. Lecture
Notes in Computer Science (including subseries Lec-
ture Notes in Artificial Intelligence and Lecture Notes
in Bioinformatics), 11383:161–169.
Bolya, D., Zhou, C., Xiao, F., and Lee, Y. J. (2019). Yolact:
Real-time instance segmentation. In ICCV.
Bolya, D., Zhou, C., Xiao, F., and Lee, Y. J. (2020).
Yolact++: Better real-time instance segmentation.
IEEE Transactions on Pattern Analysis and Machine
Intelligence.
Cai, Z. and Vasconcelos, N. (2018). Cascade r-cnn: Delv-
ing into high quality object detection. 2018 IEEE/CVF
Conference on Computer Vision and Pattern Recogni-
tion, pages 6154–6162.
Denhof, D., Staar, B., Lujen, M., and Freitag, M. (2019).
Automatic optical surface inspection of wind turbine
rotor blades using convolutional neural networks. In
52nd CIRP Conference on Manufacturing Systems,
volume 81, pages 1177–1170.
Donahue, J., Darrell, T., and Philipp, K. (2019). Adver-
sarial feature learning. In 5th International Confer-
ence on Learning Representations, ICLR 2017 - Con-
ference Track Proceedings. International Conference
on Learning Representations, ICLR.
Girshick, R. (2015). Fast r-cnn. CoRR, abs/1504.08083.
Girshick, R., Donahue, J., Darrell, T., and Malik, J. (2014).
Rich feature hierarchies for accurate object detection
and semantic segmentation. In Proceedings of the
2014 IEEE Conference on Computer Vision and Pat-
tern Recognition, page 580–587. IEEE Computer So-
ciety.
Goodfellow, I., Pouget-Abadie, J., Mirza, M., Xu, B.,
Warde-Farley, D., Ozair, S., Courville, A., and Ben-
gio, Y. (2014). Generative adversarial nets. In Ad-
vances in Neural Information Processing Systems,
volume 3, pages 2672–2680.
GWEC (2008). Global wind energy outlook 2008. Tech-
nical report, Global Wind Energy Council, Brussels,
Belgium.
He, K., Gkioxari, G., Doll
´
ar, P., and Girshick, R. (2017).
Mask r-cnn. In 2017 IEEE International Conference
on Computer Vision (ICCV), pages 2980–2988.
Lin, T.-Y., Doll
´
ar, P., Girshick, R., He, K., Hariharan, B.,
and Belongie, S. (2017). Feature pyramid networks
for object detection. In 2017 IEEE Conference on
Computer Vision and Pattern Recognition (CVPR),
pages 936–944.
Meng, H., Lien, F.-S., Yee, E., and Shen, J. (2020). Mod-
elling of anisotropic beam for rotating composite wind
turbine blade by using finite-difference time-domain
(fdtd) method. Renewable Energy, 162:2361–2379.
Miller, G. A. (1995). Wordnet: A lexical database for en-
glish. Communications ACM, 38(11):39–41.
Mishnaevsky, L., Branner, K., Petersen, H. N., Beauson, J.,
McGugan, M., and Sørensen, B. F. (2017). Materials
for wind turbine blades: An overview. In Materials,
volume 10.
Reddy, A., Indragandhi, V., Ravi, L., and Subra-
maniyaswamy, V. (2019). Detection of cracks
and damage in wind turbine blades using artificial
intelligence-based image analytics. In Measurement,
volume 147.
Redmon, J., Divvala, S., Girshick, R. B., and Farhadi, A.
(2016). You only look once: Unified, real-time object
detection. 2016 IEEE Conference on Computer Vision
and Pattern Recognition (CVPR), pages 779–788.
Redmon, J. and Farhadi, A. (2017). Yolo9000: Better,
faster, stronger. In 2017 IEEE Conference on Com-
Semi-supervised Surface Anomaly Detection of Composite Wind Turbine Blades from Drone Imagery
875