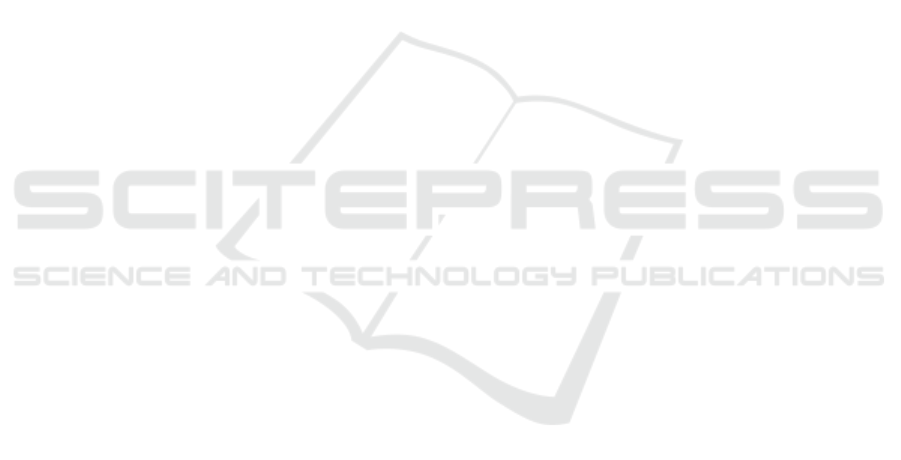
ries, evaluation instruments, prevention programs and
treatments. Frontiers in Psychology, 10:543.
De Haan, A., Landolt, M., Fried, E., Kleinke, K., Alisic,
E., Bryant, R., Salmon, K., Chen, S.-H., Liu, S.-T.,
Dalgleish, T., et al. (2019). Dysfunctional posttrau-
matic cognitions, posttraumatic stress, and depression
in children and adolescents exposed to trauma: A net-
work analysis. Journal of Child Psychology and Psy-
chiatry.
ElShawi, R., Sherif, Y., Al-Mallah, M., and Sakr, S. (2020).
Interpretability in healthcare: A comparative study
of local machine learning interpretability techniques.
Computational Intelligence.
Foland-Ross, L. C., Sacchet, M. D., Prasad, G., Gilbert, B.,
Thompson, P. M., and Gotlib, I. H. (2015). Cortical
thickness predicts the first onset of major depression
in adolescence. International Journal of Developmen-
tal Neuroscience, 46:125 – 131.
Karim, A., Mishra, A., Newton, M., and Sattar, A. (2018).
Machine learning interpretability: A science rather
than a tool. arXiv preprint arXiv:1807.06722.
Karim, M. R., Cochez, M., Beyan, O., Decker, S., and
Lange, C. (2019). Onconetexplainer: explainable pre-
dictions of cancer types based on gene expression
data. In 2019 IEEE 19th International Conference
on Bioinformatics and Bioengineering (BIBE), pages
415–422. IEEE.
Kaur, H., Nori, H., Jenkins, S., Caruana, R., Wallach, H.,
and Wortman Vaughan, J. (2020). Interpreting inter-
pretability: Understanding data scientists’ use of in-
terpretability tools for machine learning. In Proceed-
ings of the 2020 CHI Conference on Human Factors
in Computing Systems, pages 1–14.
Kovacs, M. (2003). Children’s Depression Inventory (CDI):
Technical Manual Update. Multi-Health Systems, In-
corporated.
Lundberg, S. M. and Lee, S.-I. (2017). A unified approach
to interpreting model predictions. In Proceedings of
the 31st international conference on neural informa-
tion processing systems, pages 4768–4777.
Mena, L. J. and Gonzalez, J. A. (2006). Machine learning
for imbalanced datasets: Application in medical diag-
nostic. In Flairs Conference, pages 574–579.
Mokhtari, K. E., Higdon, B. P., and Bas¸ar, A. (2019). In-
terpreting financial time series with shap values. In
Proceedings of the 29th Annual International Confer-
ence on Computer Science and Software Engineering,
pages 166–172.
Park, A. L., Fuhrer, R., and Quesnel-Vall
´
ee, A. (2013). Par-
ents’ education and the risk of major depression in
early adulthood. Social Psychiatry and Psychiatric
Epidemiology, 48(11):1829–1839.
Pasini, A. (2015). Artificial neural networks for small
dataset analysis. Journal of thoracic disease,
7(5):953.
Patel, M. J., Andreescu, C., Price, J. C., Edelman, K. L.,
Reynolds, C. F., and Aizenstein, H. J. (2015). Ma-
chine learning approaches for integrating clinical and
imaging features in late-life depression classification
and response prediction. International Journal of
Geriatric Psychiatry, 30(10):1056–1067.
Pavlova, B. and Uher, R. (2020). Assessment of psy-
chopathology: Is asking questions good enough?
JAMA psychiatry, 77(6):557–558.
Quevedo, J., Nardi, A. E., and da Silva, A. G. (2018). De-
press
˜
ao-: Teoria e Cl
´
ınica. Artmed Editora, Brasil.
Rav
`
ı, D., Wong, C., Deligianni, F., Berthelot, M., Andreu-
Perez, J., Lo, B., and Yang, G.-Z. (2017). Deep learn-
ing for health informatics. IEEE journal of biomedical
and health informatics, 21(1):4–21.
Schwan, S. and Ramires, V. R. R. (2017). Depress
˜
ao em
crianc¸as: Uma breve revis
˜
ao de literatura. Psicologia
Argumento, 29(67).
Scivoletto, S. and Tarelho, L. G. (2002). Depress
¨
ao
na inf
ˆ
ancia e adolesc
ˆ
encia. RBM rev. bras. med,
59(8):555–558.
Sendi, I., Chouikh, A., Ammar, A., and Bouafia, N. (2018).
Depression in a sample of tunisian adolescents: preva-
lence, associated factors and comorbidity with anx-
iety disorders. International journal of adolescent
medicine and health.
Singhal, S. and Jena, M. (2013). A study on weka tool for
data preprocessing, classification and clustering. In-
ternational Journal of Innovative technology and ex-
ploring engineering (IJItee), 2(6):250–253.
Vinnakota, A. and Kaur, R. (2018). A study of depres-
sion, externalizing, and internalizing behaviors among
adolescents living in institutional homes. Interna-
tional Journal of Applied and Basic Medical Re-
search, 8(2):89.
WHO, W. H. O. (2017). Depression and other common
mental disorders: global health estimates. http://www.
who.int/iris/handle/10665/254610.
Wu, M.-J., Wu, H. E., Mwangi, B., Sanches, M., Selvaraj,
S., Zunta-Soares, G. B., and Soares, J. C. (2015). Pre-
diction of pediatric unipolar depression using multiple
neuromorphometric measurements: A pattern classi-
fication approach. Journal of Psychiatric Research,
62:84 – 91.
Yang, L., Jiang, D., He, L., Pei, E., Oveneke, M. C., and
Sahli, H. (2016). Decision Tree Based Depression
Classification from Audio Video and Language Infor-
mation. In Proceedings of the 6th International Work-
shop on Audio/Visual Emotion Challenge - AVEC ’16,
AVEC ’16, pages 89–96, New York, NY, USA. ACM.
Yoon, S., Taha, B., and Bakken, S. (2014). Using a data
mining approach to discover behavior correlates of
chronic disease: A case study of depression. In Stud-
ies in Health Technology and Informatics, volume
201, pages 71–78.
Zavaschi, M. L. S., Satler, F., Poester, D., Vargas, C. F., Pi-
azenski, R., Rohde, L. A. P., and Eizirik, C. L. (2002).
Associac¸
˜
ao entre trauma por perda na inf
ˆ
ancia e de-
press
˜
ao na vida adulta. Revista brasileira de psiquia-
tria= Brazilian journal of psychiatry. S
˜
ao Paulo, SP.
Vol. 24, n. 4 (out. 2002), p. 189-195.
Predicting Depression in Children and Adolescents using the SHAP Approach
521