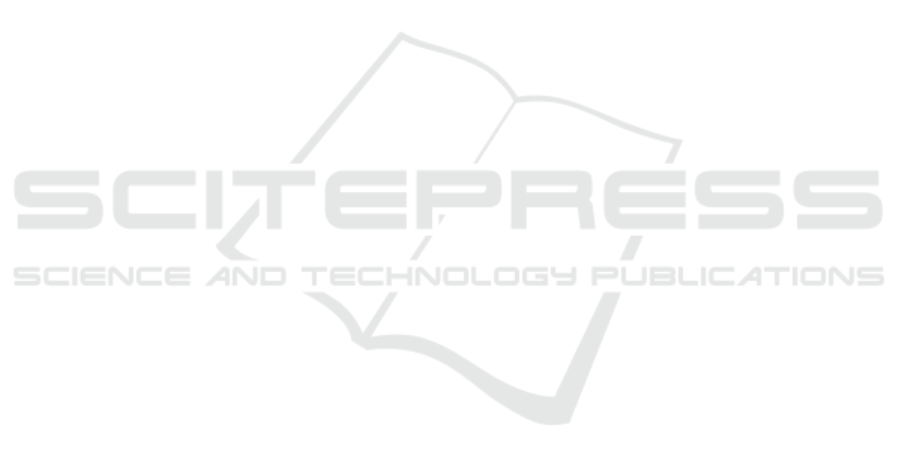
prove detection matching. We found that with the in-
clusion of these appearance features, both MOTA and
MOTP are slightly increased.
In future work, we intend to track 2D skeletons of
pedestrians in each camera (Xiu et al., 2018) instead
of tracking only ground plane points to use this infor-
mation to improve 3D pedestrian tracking.
ACKNOWLEDGEMENTS
The authors would like to thank Conselho Nacional
de Desenvolvimento Cient
´
ıfico e Tecnol
´
ogico (CNPq)
(process 425401/2018-9) for partially funding this re-
search.
REFERENCES
Bernardin, K. and Stiefelhagen, R. (2008). Evaluating mul-
tiple object tracking performance: the clear mot met-
rics. EURASIP Journal on Image and Video Process-
ing, 2008:1–10.
Bewley, A., Ge, Z., Ott, L., Ramos, F., and Upcroft, B.
(2016). Simple online and realtime tracking. In 2016
IEEE international conference on image processing
(ICIP), pages 3464–3468. IEEE.
Chavdarova, T., Baqu
´
e, P., Bouquet, S., Maksai, A., Jose,
C., Bagautdinov, T., Lettry, L., Fua, P., Van Gool, L.,
and Fleuret, F. (2018). Wildtrack: A multi-camera
hd dataset for dense unscripted pedestrian detection.
In Proceedings of the IEEE Conference on Computer
Vision and Pattern Recognition, pages 5030–5039.
Chen, Y., Zhao, Q., An, Z., Lv, P., and Zhao, L. (2016).
Distributed multi-target tracking based on the k-mtscf
algorithm in camera networks. IEEE Sensors Journal,
16(13):5481–5490.
Galil, Z. (1986). Efficient algorithms for finding maxi-
mum matching in graphs. ACM Computing Surveys
(CSUR), 18(1):23–38.
Heindl, C. (2017). py-motmetrics. https://github.com/
cheind/py-motmetrics.
Hellinger, E. (1909). Neue begr
¨
undung der theo-
rie quadratischer formen von unendlichvielen
ver
¨
anderlichen. Journal f
¨
ur die reine und angewandte
Mathematik, 1909(136):210–271.
Kalman, R. E. (1960). A new approach to linear filtering
and prediction problems.
Leal-Taix
´
e, L., Milan, A., Reid, I., Roth, S., and Schindler,
K. (2015). Motchallenge 2015: Towards a bench-
mark for multi-target tracking. arXiv preprint
arXiv:1504.01942.
Lima, J. P., Roberto, R., Figueiredo, L., Simoes, F., and
Teichrieb, V. (2021). Generalizable multi-camera 3d
pedestrian detection. In Proceedings of the IEEE/CVF
Conference on Computer Vision and Pattern Recogni-
tion, pages 1232–1240.
Ong, J., Vo, B. T., Vo, B. N., Kim, D. Y., and Nord-
holm, S. (2020). A bayesian filter for multi-view 3d
multi-object tracking with occlusion handling. arXiv
preprint arXiv:2001.04118.
Papakis, I., Sarkar, A., and Karpatne, A. (2020). Gc-
nnmatch: Graph convolutional neural networks for
multi-object tracking via sinkhorn normalization.
arXiv preprint arXiv:2010.00067.
Song, J., Yang, Y., Song, Y.-Z., Xiang, T., and Hospedales,
T. M. (2019). Generalizable person re-identification
by domain-invariant mapping network. In Proceed-
ings of the IEEE/CVF Conference on Computer Vision
and Pattern Recognition, pages 719–728.
Sun, H., Chen, Y., Aved, A., and Blasch, E. (2020a).
Collaborative multi-object tracking as an edge ser-
vice using transfer learning. In 2020 IEEE 22nd In-
ternational Conference on High Performance Com-
puting and Communications; IEEE 18th Interna-
tional Conference on Smart City; IEEE 6th Inter-
national Conference on Data Science and Systems
(HPCC/SmartCity/DSS), pages 1112–1119. IEEE.
Sun, Z., Chen, J., Chao, L., Ruan, W., and Mukherjee,
M. (2020b). A survey of multiple pedestrian track-
ing based on tracking-by-detection framework. IEEE
Transactions on Circuits and Systems for Video Tech-
nology, 31(5):1819–1833.
Vo, B.-N., Vo, B.-T., and Hoang, H. G. (2016). An effi-
cient implementation of the generalized labeled multi-
bernoulli filter. IEEE Transactions on Signal Process-
ing, 65(8):1975–1987.
Vo, M. P., Yumer, E., Sunkavalli, K., Hadap, S., Sheikh,
Y. A., and Narasimhan, S. G. (2020). Self-supervised
multi-view person association and its applications.
IEEE transactions on pattern analysis and machine
intelligence.
Wojke, N., Bewley, A., and Paulus, D. (2017). Simple on-
line and realtime tracking with a deep association met-
ric. In 2017 IEEE international conference on image
processing (ICIP), pages 3645–3649. IEEE.
Xiu, Y., Li, J., Wang, H., Fang, Y., and Lu, C. (2018). Pose
flow: Efficient online pose tracking. In BMVC.
Ye, M., Shen, J., Lin, G., Xiang, T., Shao, L., and Hoi, S. C.
(2021). Deep learning for person re-identification: A
survey and outlook. IEEE Transactions on Pattern
Analysis and Machine Intelligence.
You, Q. and Jiang, H. (2020). Real-time 3d deep multi-
camera tracking. arXiv preprint arXiv:2003.11753.
Zhou, X., Koltun, V., and Kr
¨
ahenb
¨
uhl, P. (2020). Tracking
objects as points. In European Conference on Com-
puter Vision, pages 474–490. Springer.
Zhu, C. (2019). Multi-camera people detection and track-
ing.
Generalizable Online 3D Pedestrian Tracking with Multiple Cameras
827