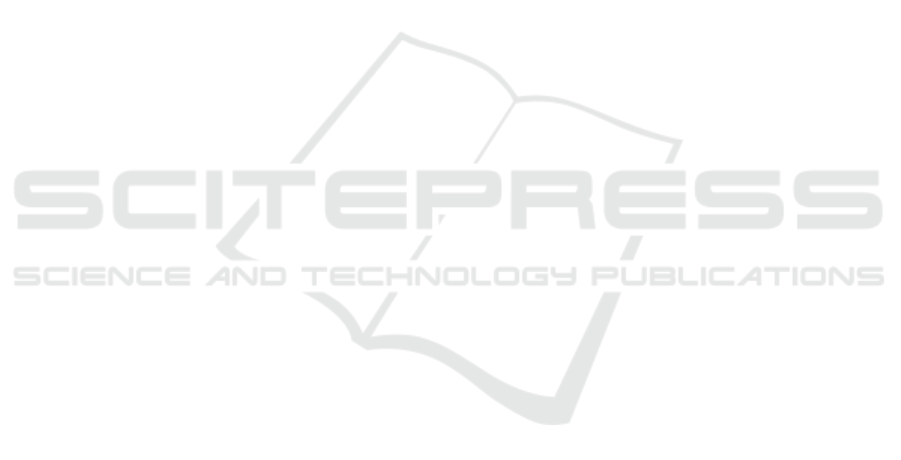
interface. IEEE Transactions on Neural Networks and
Learning Systems, pages 1–13.
Goodfellow, I. J., Pouget-Abadie, J., Mirza, M., Xu, B.,
Warde-Farley, D., Ozair, S., Courville, A., and Ben-
gio, Y. (2014). Generative adversarial nets. In
Proceedings of the 27th International Conference on
Neural Information Processing Systems - Volume 2,
NIPS’14, page 2672–2680, Cambridge, MA, USA.
MIT Press.
Green, M. A. (2018). Use of machine learning approaches
to compare the contribution of different types of data
for predicting an individual’s risk of ill health: an ob-
servational study. The Lancet, 392:p.S40.
Han, C., Murao, K., Noguchi, T., Kawata, Y., Uchiyama,
F., Rundo, L., Nakayama, H., and Satoh, S. (2019).
Learning more with less: Conditional pggan-based
data augmentation for brain metastases detection us-
ing highly-rough annotation on mr images. In Pro-
ceedings of the 28th ACM International Conference
on Information and Knowledge Management, CIKM
’19, page 119–127, New York, NY, USA. Association
for Computing Machinery.
Haradal, S., Hayashi, H., and Uchida, S. (2018). Biosig-
nal data augmentation based on generative adversarial
networks. In 2018 40th Annual International Confer-
ence of the IEEE Engineering in Medicine and Biol-
ogy Society (EMBC), pages 368–371.
Herland, M., Khoshgoftaar, T., and Wald, R. (2014). A re-
view of data mining using big data in health informat-
ics. Journal Of Big Data, 1:2.
Hu, H., Tan, T., and Qian, Y. (2018). Generative adversar-
ial networks based data augmentation for noise robust
speech recognition. In 2018 IEEE International Con-
ference on Acoustics, Speech and Signal Processing
(ICASSP).
Hussain, Z., Gimenez, F., Yi, D., and Rubin, D. (2018).
Differential data augmentation techniques for medical
imaging classification tasks. AMIA ... Annual Sympo-
sium proceedings. AMIA Symposium, 2017:979–984.
Ioffe, S. and Szegedy, C. (2015). Batch normalization:
Accelerating deep network training by reducing in-
ternal covariate shift. In Bach, F. and Blei, D., ed-
itors, Proceedings of the 32nd International Confer-
ence on Machine Learning, volume 37 of Proceedings
of Machine Learning Research, pages 448–456, Lille,
France. PMLR.
Japkowicz, N. (2000a). The class imbalance problem: Sig-
nificance and strategies. In Proc. of the Int’l Conf. on
Artificial Intelligence, volume 56. Citeseer.
Japkowicz, N. (2000b). The class imbalance problem: Sig-
nificance and strategies. Proceedings of the 2000 In-
ternational Conference on Artificial Intelligence ICAI.
Karadag, O. O. and Erdas¸ Cicek, O. (2019). Experimen-
tal assessment of the performance of data augmenta-
tion with generative adversarial networks in the image
classification problem. In 2019 Innovations in Intel-
ligent Systems and Applications Conference (ASYU),
pages 1–4.
Lima, G. P., Rozenbaum, D., Pimentel, C., Frota, A. C. C.,
Vivacqua, D., Machado, E. S., das Neves Sztajnbok,
F. C., Abreu, T., Soares, R. A., and Hofer, C. B.
(2019). Factors associated with the development of
congenital zika syndrome: a case-control study. In
BMC Infectious Diseases.
Mariani, G., Scheidegger, F., Istrate, R., Bekas, C., and
Malossi, A. C. I. (2018). Bagan: Data augmentation
with balancing gan. arXiv, pages 1–9.
Mehta, K., Kobti, Z., Pfaff, K., and Fox, S. (2019). Data
augmentation using ca evolved gans. 2019 IEEE Sym-
posium on Computers and Communications (ISCC),
pages 1087–1092.
Milovic, B. and Milovic, M. (2012). Prediction and decision
making in health care using data mining. International
Journal of Public Health Science (IJPHS), 1.
Mullick, S. S., Datta, S., and Das, S. (2019). Generative ad-
versarial minority oversampling. In 2019 IEEE/CVF
International Conference on Computer Vision (ICCV),
pages 1695–1704.
Pan, Z., Yu, W., Yi, X., Khan, A., Yuan, F., and Zheng,
Y. (2019). Recent progress on generative adversarial
networks (gans): A survey. IEEE Access, 7:36322–
36333.
Ribeiro, I. G., Andrade, M. R. d., Silva, J. d. M. S., Silva,
Z. M., Costa, M. A. d. O., Vieira, M. A. d. C. e. S.,
Batista, F. M. d. A., Guimar
˜
aes, H., Wada, M. Y.,
and Saad, E. (2018). Microcefalia no Piau
´
ı, Brasil:
estudo descritivo durante a epidemia do v
´
ırus Zika,
2015-2016. Epidemiologia e Servic¸o de Sa
´
ude, 27.
Salimans, T., Goodfellow, I., Zaremba, W., Cheung, V.,
Radford, A., Chen, X., and Chen, X. (2016). Im-
proved techniques for training gans. In Lee, D.,
Sugiyama, M., Luxburg, U., Guyon, I., and Garnett,
R., editors, Advances in Neural Information Process-
ing Systems, volume 29. Curran Associates, Inc.
Sheng, P., Yang, Z., and Qian, Y. (2019). Gans for children:
A generative data augmentation strategy for children
speech recognition. In 2019 IEEE Automatic Speech
Recognition and Understanding Workshop (ASRU),
pages 129–135.
Tomar, D. (2013). A survey on data mining approaches for
healthcare. International Journal of Bio - Science and
Bio - Technology, 5:241–266.
Wang, S., Lv, Y.-D., Sui, Y., Liu, S., Wang, S.-J., and
Zhang, Y.-D. (2017). Alcoholism detection by data
augmentation and convolutional neural network with
stochastic pooling. Journal of Medical Systems, 42.
Weng, S. F. R., J.; Kai, J. G., and J. M.; Qureshi, N. (2017).
Can machinelearning improve cardiovascular risk pre-
diction using routine clinical data? Public Library of
Science, 12(4):e0174944.
Yu, X., Wu, X., Luo, C., and Ren, P. (2017). Deep learning
in remote sensing scene classification: a data augmen-
tation enhanced convolutional neural network frame-
work. GIScience & Remote Sensing, 54:1–18.
Zanluca, C., Noronha, L., and Santos, C. (2017). Maternal-
fetal transmission of the zika virus: An intriguing in-
terplay. Tissue Barriers, 6:00–00.
HEALTHINF 2022 - 15th International Conference on Health Informatics
102