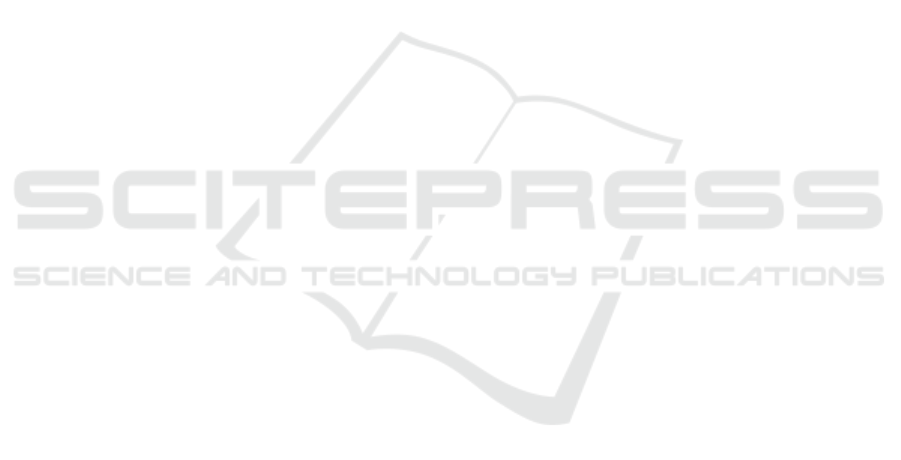
dividual. However, certain medical conditions such
as depression can impact the mobility pattern. Conse-
quently, the affected individual’s movements are sig-
nificantly altered compared to their healthy counter-
parts. However, the degradation in mobility can be
used as a vital parameter in characterizing the disor-
der. In the past, physicians assessed the depression
by an observation followed by self-reported feedback
from the patients. Yet, with the latest innovations in
wearable devices, it is possible to diagnose the ill-
ness by collecting mobility data from depressed pa-
tients using wearable sensors. In this study, we pro-
posed and built a correlation network model by utiliz-
ing the movement data collected from the group con-
sisting of depressed as well as healthy subjects. Ear-
lier studies predominantly focused on prediction of
the depression by incorporating known labels. How-
ever, our hypothesis is built on the concept of pop-
ulation analysis and correlation network by utilizing
the mobility data. We treated all the subjects belong-
ing to one group then explored similarities and differ-
ences between each pair of subjects by utilizing their
movement data. Then we constructed a correlation
network model that has the potential to discover the
subgroups of those who are suffering from depression
and healthy subjects. We have extracted three differ-
ent granularity of features and we found that hour-
wise features are the best set of feature parameters
that can fairly identify the subgroups.
REFERENCES
Abuse, S. (2018). Mental health services administra-
tion.(2017). key substance use and mental health in-
dicators in the united states: Results from the 2016
national survey on drug use and health (hhs publica-
tion no. sma 17-5044, nsduh series h-52). rockville,
md: Center for behavioral health statistics and qual-
ity. Substance Abuse and Mental Health Services
Administration. Retrieved from https://www. samhsa.
gov/data.
Ali, N., Neagu, D., and Trundle, P. (2019). Evaluation of
k-nearest neighbour classifier performance for hetero-
geneous data sets. SN Applied Sciences, 1(12):1–15.
Curtin, S. C., Warner, M., and Hedegaard, H. (2016). In-
crease in suicide in the United States, 1999-2014.
Number 2016. US Department of Health and Human
Services, Centers for Disease Control and . . . .
Dongen, S. (2000). Performance criteria for graph cluster-
ing and Markov cluster experiments. CWI (Centre for
Mathematics and Computer Science).
Galv
´
an-Tejada, C. E., Zanella-Calzada, L. A., Gamboa-
Rosales, H., Galv
´
an-Tejada, J. I., Ch
´
avez-Lamas,
N. M., Gracia-Cort
´
es, M., Magallanes-Quintanar, R.,
Celaya-Padilla, J. M., et al. (2019). Depression
episodes detection in unipolar and bipolar patients: A
methodology with feature extraction and feature se-
lection with genetic algorithms using activity motion
signal as information source. Mobile Information Sys-
tems, 2019.
Garcia-Ceja, E., Riegler, M., Jakobsen, P., Tørresen, J.,
Nordgreen, T., Oedegaard, K. J., and Fasmer, O. B.
(2018). Depresjon: a motor activity database of de-
pression episodes in unipolar and bipolar patients. In
Proceedings of the 9th ACM multimedia systems con-
ference, pages 472–477.
Girvan, M. and Newman, M. E. (2002). Community struc-
ture in social and biological networks. Proceedings of
the national academy of sciences, 99(12):7821–7826.
Heinze, F., Hesels, K., Breitbach-Faller, N., Schmitz-Rode,
T., and Disselhorst-Klug, C. (2010). Movement anal-
ysis by accelerometry of newborns and infants for the
early detection of movement disorders due to infantile
cerebral palsy. Medical & biological engineering &
computing, 48(8):765–772.
Montgomery, S. A. and
˚
Asberg, M. (1979). A new depres-
sion scale designed to be sensitive to change. The
British journal of psychiatry, 134(4):382–389.
Radloff, L. S. (1977). The ces-d scale: A self-report de-
pression scale for research in the general population.
Applied psychological measurement, 1(3):385–401.
Rodr
´
ıguez-Ruiz, J. G., Galv
´
an-Tejada, C. E., Zanella-
Calzada, L. A., Celaya-Padilla, J. M., Galv
´
an-
Tejada, J. I., Gamboa-Rosales, H., Luna-Garc
´
ıa, H.,
Magallanes-Quintanar, R., and Soto-Murillo, M. A.
(2020). Comparison of night, day and 24 h motor ac-
tivity data for the classification of depressive episodes.
Diagnostics, 10(3):162.
Sobin, C. and Sackeim, H. A. (1997). Psychomotor symp-
toms of depression. American Journal of Psychiatry,
154(1):4–17.
The National Institute of Mental Health (NIMH) (2021).
Depression. [Online; accessed 8-August-2021].
The World Health Organization(WHO) (2021). Depression.
[Online; accessed 6-October-2021].
Zanella-Calzada, L. A., Galv
´
an-Tejada, C. E., Ch
´
avez-
Lamas, N. M., Gracia-Cort
´
es, M., Magallanes-
Quintanar, R., Celaya-Padilla, J. M., Galv
´
an-Tejada,
J. I., and Gamboa-Rosales, H. (2019). Feature extrac-
tion in motor activity signal: Towards a depression
episodes detection in unipolar and bipolar patients.
Diagnostics, 9(1):8.
The Comparison of Various Correlation Network Models in Studying Mobility Data for the Analysis of Depression Episodes
207