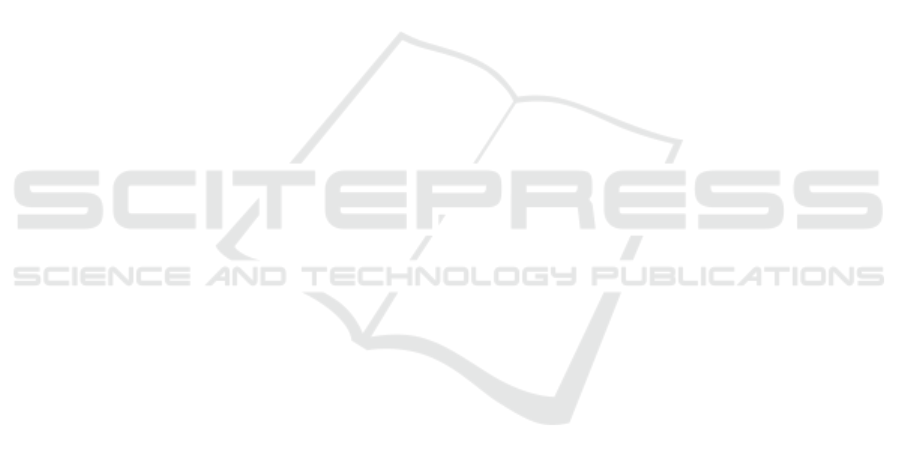
ing habits, such as beverages and alcohol intake.
We can avoid the Bluetooth connection issues be-
tween IMU and smartphone by implementing the RF
model on the smartwatch. Finally, a further test of
the pipeline on more subjects would be required for
validating these results.
ACKNOWLEDGEMENTS
We thank all our subjects who gave data for this study.
We are grateful to the Federal Ministry of Economic
Affairs and Energy for generously funding this project
with funding number ZF4776601HB9. We also ex-
tend our gratitude to Dr.-Ing.Harry Freitas da Cruz
and Pascal Hecker for proofreading the paper.
REFERENCES
Amft, O., Junker, H., and Troster, G. (2005). Detection of
eating and drinking arm gestures using inertial body-
worn sensors. In Ninth IEEE international symposium
on wearable computers (ISWC’05), pages 160–163.
IEEE.
Barandas, M., Folgado, D., Fernandes, L., Santos, S.,
Abreu, M., Bota, P., Liu, H., Schultz, T., and Gam-
boa, H. (2020). Tsfel: Time series feature extraction
library. SoftwareX, 11:100456.
Bedri, A., Li, R., Haynes, M., Kosaraju, R. P., Grover, I.,
Prioleau, T., Beh, M. Y., Goel, M., Starner, T., and
Abowd, G. (2017). Earbit: using wearable sensors to
detect eating episodes in unconstrained environments.
Proceedings of the ACM on interactive, mobile, wear-
able and ubiquitous technologies, 1(3):1–20.
Biallas, M., Andrushevich, A., Kistler, R., Klapproth, A.,
Czuszynski, K., and Bujnowski, A. (2015). Feasibil-
ity study for food intake tasks recognition based on
smart glasses. Journal of Medical Imaging and Health
Informatics, 5(8):1688–1694.
Buitinck, L., Louppe, G., Blondel, M., Pedregosa, F.,
Mueller, A., Grisel, O., Niculae, V., Prettenhofer, P.,
Gramfort, A., Grobler, J., et al. (2013). Api design
for machine learning software: experiences from the
scikit-learn project. arXiv preprint arXiv:1309.0238.
Dong, Y., Hoover, A., and Muth, E. (2009). A device for
detecting and counting bites of food taken by a person
during eating. In 2009 IEEE International Conference
on Bioinformatics and Biomedicine, pages 265–268.
IEEE.
Dong, Y., Scisco, J., Wilson, M., Muth, E., and Hoover, A.
(2013). Detecting periods of eating during free-living
by tracking wrist motion. IEEE journal of biomedical
and health informatics, 18(4):1253–1260.
Gallego, J. A., Rocon, E., Roa, J. O., Moreno, J. C., and
Pons, J. L. (2010). Real-time estimation of patholog-
ical tremor parameters from gyroscope data. Sensors,
10(3):2129–2149.
Guyon, I. and Elisseeff, A. (2003). An introduction to vari-
able and feature selection. Journal of machine learn-
ing research, 3(Mar):1157–1182.
Helander, E., Kaipainen, K., Korhonen, I., and Wansink, B.
(2014). Factors related to sustained use of a free mo-
bile app for dietary self-monitoring with photography
and peer feedback: retrospective cohort study. Journal
of medical Internet research, 16(4):e109.
Kappattanavar, A. M., Da Cruz, H. F., Arnrich, B., and
B
¨
ottinger, E. (2020). Position matters: Sensor place-
ment for sitting posture classification. In 2020 IEEE
International Conference on Healthcare Informatics
(ICHI), pages 1–6. IEEE.
Paul, S. (2020). psambit9791/jdsp. https://doi.org/10.5281/
zenodo.5137347. Accessed :2021-09-14.
Pedregosa, F., Varoquaux, G., Gramfort, A., Michel, V.,
Thirion, B., Grisel, O., Blondel, M., Prettenhofer, P.,
Weiss, R., Dubourg, V., et al. (2011). Scikit-learn:
Machine learning in python. the Journal of machine
Learning research, 12:2825–2830.
Porr, B. (2021). An efficient iir filter library written in java.
https://github.com/berndporr/iirj. Accessed :2021-09-
14.
Ruusmann, V. (2014). Jpmml-evaluator. https://github.com/
jpmml/jpmml-evaluator. Accessed :2021-09-14.
Ruusmann, V. (2016). Jpmml-sklearn. https://github.com/
jpmml/jpmml-sklearn. Accessed :2021-09-14.
Ruusmann, V. (2017). Jpmml-android. https://github.com/
jpmml/jpmml-android. Accessed :2021-09-14.
Sen, S., Subbaraju, V., Misra, A., Balan, R., and
Lee, Y. (2018). Annapurna: building a real-
world smartwatch-based automated food journal. In
2018 IEEE 19th International Symposium on” A
World of Wireless, Mobile and Multimedia Net-
works”(WoWMoM), pages 1–6. IEEE.
Shim, J.-S., Oh, K., and Kim, H. C. (2014). Dietary assess-
ment methods in epidemiologic studies. Epidemiology
and health, 36.
Stankoski, S., Jordan, M., Gjoreski, H., and Lu
ˇ
strek, M.
(2021). Smartwatch-based eating detection: Data se-
lection for machine learning from imbalanced data
with imperfect labels. Sensors, 21(5):1902.
Stankoski, S., Resc¸ic¸, N., Mezic, G., and Lustrek, M.
(2020). Real-time eating detection using a smart-
watch. In EWSN, pages 247–252.
Thomaz, E., Essa, I., and Abowd, G. D. (2015). A practical
approach for recognizing eating moments with wrist-
mounted inertial sensing. In Proceedings of the 2015
ACM international joint conference on pervasive and
ubiquitous computing, pages 1029–1040.
Thompson, F. E., Subar, A. F., Loria, C. M., Reedy, J. L.,
and Baranowski, T. (2010). Need for technological in-
novation in dietary assessment. Journal of the Ameri-
can Dietetic Association, 110(1):48–51.
WHO (2021). Diabetes. https://www.who.int/news-room/
fact-sheets/detail/diabetes. Accessed: 2021-09-06.
Zhou, L., Tunca, C., Fischer, E., Brahms, C. M., Ersoy,
C., Granacher, U., and Arnrich, B. (2020). Valida-
tion of an imu gait analysis algorithm for gait moni-
toring in daily life situations. In 2020 42nd Annual
International Conference of the IEEE Engineering in
Medicine & Biology Society (EMBC), pages 4229–
4232. IEEE.
Have Your Cake and Log it Too: A Pilot Study Leveraging IMU Sensors for Real-time Food Journaling Notifications
541