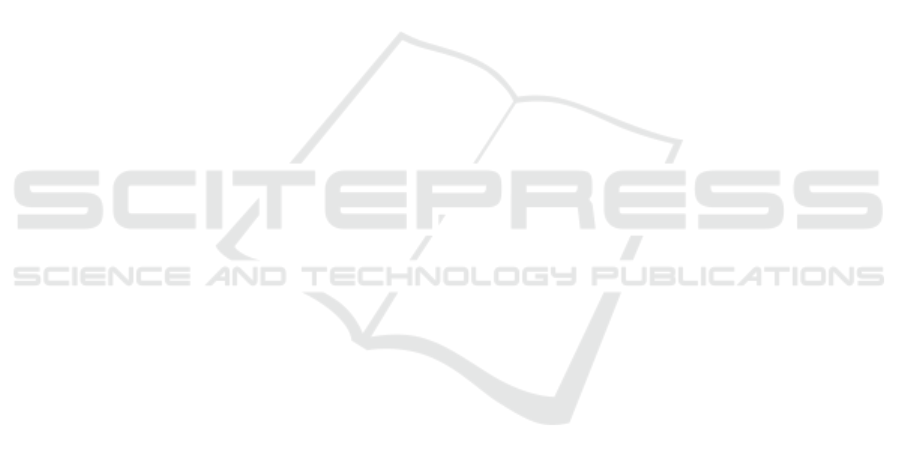
REFERENCES
Barraza, R., Eames, R., Balducci, Y. E., Hinds, J., Hooger-
werf, S., Horvitz, E., Kamar, E., Krones, J., Lovejoy,
J., Mohadjer, P., Noah, B., and Nushi, B. (2019). Er-
ror Terrain Analysis for Machine Learning: Tools and
Visualizations. page 4.
Biederman, I. (1987). Recognition-by-components: A the-
ory of human image understanding. Psychological Re-
view, 94(2):115–147.
Brooks, M., Amershi, S., Lee, B., Drucker, S. M., Kapoor,
A., and Simard, P. (2015). FeatureInsight: Visual sup-
port for error-driven feature ideation in text classifica-
tion. In 2015 IEEE Conference on Visual Analytics
Science and Technology (VAST), pages 105–112.
Chen, C., Yuan, J., Lu, Y., Liu, Y., Su, H., Yuan, S., and Liu,
S. (2021). OoDAnalyzer: Interactive Analysis of Out-
of-Distribution Samples. IEEE Transactions on Visu-
alization and Computer Graphics, 27(7):3335–3349.
Chen, J., Song, L., Wainwright, M. J., and Jordan, M. I.
(2019). L-Shapley and C-Shapley: Efficient Model
Interpretation for Structured Data. In International
Conference on Learning Representations.
Conversation AI (2020). Unintended Bias Analysis.
Dixon, L., Li, J., Sorensen, J., Thain, N., and Vasserman, L.
(2018). Measuring and Mitigating Unintended Bias
in Text Classification. In Proceedings of the 2018
AAAI/ACM Conference on AI, Ethics, and Society,
pages 67–73. ACM.
Fischer, M. (1966). The KWIC index concept: A retrospec-
tive view. American Documentation, 17(2):57–70.
Hoover, B., Strobelt, H., and Gehrmann, S. (2020).
exBERT: A visual analysis tool to explore learned rep-
resentations in Transformer models. In Proceedings of
the 58th Annual Meeting of the Association for Com-
putational Linguistics: System Demonstrations, pages
187–196, Online. Association for Computational Lin-
guistics.
InterpretML (2021). Interpret-Text - Alpha Release.
Jessamyn (2017). I tested 14 sentences for ”perceived tox-
icity” using Perspectives. Least toxic: I am a man.
Most toxic: I am a gay black woman. Come on
https://t.co/M4TF9uYtzE.
Jigsaw (2018). Unintended bias and names of frequently
targeted groups.
Krause, J., Perer, A., and Ng, K. (2016). Interacting with
Predictions: Visual Inspection of Black-box Machine
Learning Models. In Proceedings of the 2016 CHI
Conference on Human Factors in Computing Systems,
CHI ’16, pages 5686–5697, New York, NY, USA. As-
sociation for Computing Machinery.
Li, J., Chen, X., Hovy, E., and Jurafsky, D. (2016). Vi-
sualizing and Understanding Neural Models in NLP.
In Proceedings of the 2016 Conference of the North
American Chapter of the Association for Computa-
tional Linguistics: Human Language Technologies,
pages 681–691, San Diego, California. Association
for Computational Linguistics.
Liu, S., Chen, C., Lu, Y., Ouyang, F., and Wang, B. (2019).
An Interactive Method to Improve Crowdsourced An-
notations. IEEE Transactions on Visualization and
Computer Graphics, 25(1):235–245.
Lundberg, S. M. and Lee, S.-I. (2017). A Unified Ap-
proach to Interpreting Model Predictions. In Guyon,
I., Luxburg, U. V., Bengio, S., Wallach, H., Fergus, R.,
Vishwanathan, S., and Garnett, R., editors, Advances
in Neural Information Processing Systems 30, pages
4765–4774. Curran Associates, Inc.
Maas, A. L., Daly, R. E., Pham, P. T., Huang, D., Ng, A. Y.,
and Potts, C. (2011). Learning Word Vectors for Sen-
timent Analysis. In Proceedings of the 49th Annual
Meeting of the Association for Computational Lin-
guistics: Human Language Technologies, pages 142–
150. Association for Computational Linguistics.
Ribeiro, M. T., Singh, S., and Guestrin, C. (2016). ”Why
Should I Trust You?”: Explaining the Predictions
of Any Classifier. In Proceedings of the 22nd
ACM SIGKDD International Conference on Knowl-
edge Discovery and Data Mining, KDD ’16, pages
1135–1144, New York, NY, USA. ACM.
Rohrdantz, C., Hao, M. C., Dayal, U., Haug, L.-E., and
Keim, D. A. (2012). Feature-Based Visual Sentiment
Analysis of Text Document Streams. ACM Trans. In-
tell. Syst. Technol., 3(2):26:1–26:25.
Stoffel, F., Flekova, L., Oelke, D., Gurevych, I., and Keim,
D. A. (2015). Feature-Based Visual Exploration of
Text Classification. In Symposium on Visualization in
Data Science (VDS) at IEEE VIS 2015.
Tenney, I., Wexler, J., Bastings, J., Bolukbasi, T., Co-
enen, A., Gehrmann, S., Jiang, E., Pushkarna, M.,
Radebaugh, C., Reif, E., and Yuan, A. (2020). The
Language Interpretability Tool: Extensible, Interac-
tive Visualizations and Analysis for NLP Models.
arXiv:2008.05122 [cs].
Thain, N., Dixon, L., and Wulczyn, E. (2017). Wikipedia
talk labels: Toxicity.
Wexler, J., Pushkarna, M., Bolukbasi, T., Wattenberg, M.,
Vi
´
egas, F., and Wilson, J. (2020). The What-If
Tool: Interactive Probing of Machine Learning Mod-
els. IEEE Transactions on Visualization and Com-
puter Graphics, 26(1):56–65.
Yuan, J., Chen, C., Yang, W., Liu, M., Xia, J., and Liu,
S. (2021). A survey of visual analytics techniques
for machine learning. Computational Visual Media,
7(1):3–36.
Zhang, J., Wang, Y., Molino, P., Li, L., and Ebert, D. S.
(2019). Manifold: A Model-Agnostic Framework
for Interpretation and Diagnosis of Machine Learn-
ing Models. IEEE Transactions on Visualization and
Computer Graphics, 25(1):364–373.
IVAPP 2022 - 13th International Conference on Information Visualization Theory and Applications
170