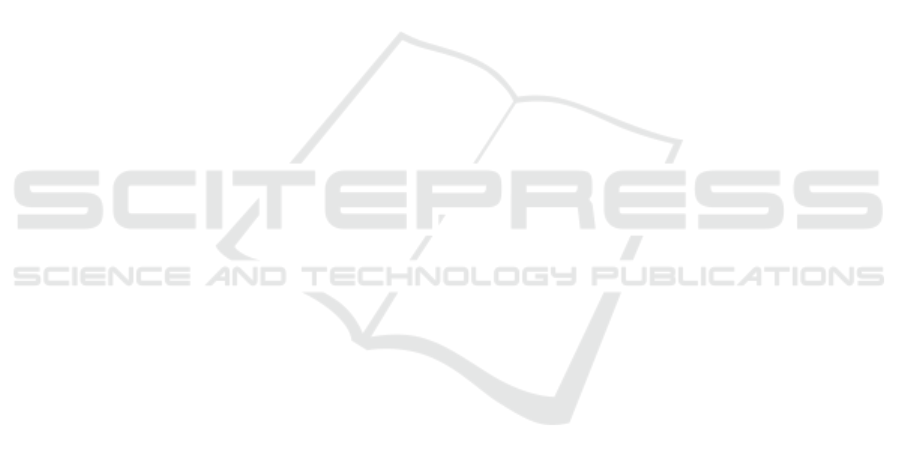
spine surgeries (MISS) using rapid prototyping. Bone
(CT), 226:3071.
Goodfellow, I., Pouget-Abadie, J., Mirza, M., Xu, B.,
Warde-Farley, D., Ozair, S., Courville, A., and Ben-
gio, Y. (2014). Generative adversarial nets. Advances
in neural information processing systems, 27.
Han, X. (2017). MR-based synthetic CT generation using a
deep convolutional neural network method. Medical
physics, 44(4):1408–1419.
Heusel, M., Ramsauer, H., Unterthiner, T., Nessler, B., and
Hochreiter, S. (2017). Gans trained by a two time-
scale update rule converge to a local nash equilibrium.
Advances in neural information processing systems,
30.
Keereman, V., Mollet, P., Berker, Y., Schulz, V., and Van-
denberghe, S. (2013). Challenges and current methods
for attenuation correction in PET/MR. Magnetic Res-
onance Materials in Physics, Biology and Medicine,
26(1):81–98.
Kingma, D. P. and Ba, J. (2014). Adam: A
method for stochastic optimization. arXiv preprint
arXiv:1412.6980.
Leynes, A. P., Yang, J., Wiesinger, F., Kaushik, S. S.,
Shanbhag, D. D., Seo, Y., Hope, T. A., and Larson,
P. E. (2018). Zero-echo-time and dixon deep pseudo-
ct (ZeDD CT): direct generation of pseudo-CT images
for pelvic PET/MRI attenuation correction using deep
convolutional neural networks with multiparametric
MRI. Journal of Nuclear Medicine, 59(5):852–858.
Liu, F., Jang, H., Kijowski, R., Bradshaw, T., and McMil-
lan, A. B. (2017). Deep learning MR imaging–based
attenuation correction for PET/MR imaging. Radiol-
ogy, 286(2):676–684.
Lowekamp, B. C., Chen, D. T., Ib
´
a
˜
nez, L., and Blezek, D.
(2013). The design of SimpleITK. Frontiers in neu-
roinformatics, 7:45.
Mattes, D., Haynor, D. R., Vesselle, H., Lewellen, T. K.,
and Eubank, W. (2003). PET-CT image registration in
the chest using free-form deformations. IEEE trans-
actions on medical imaging, 22(1):120–128.
Mecheter, I., Alic, L., Abbod, M., Amira, A., and Ji, J.
(2020). MR image-based attenuation correction of
brain PET imaging: Review of literature on machine
learning approaches for segmentation. Journal of Dig-
ital Imaging, 33(5):1224.
Nie, D., Cao, X., Gao, Y., Wang, L., and Shen, D. (2016).
Estimating CT image from MRI data using 3D fully
convolutional networks. In Deep Learning and Data
Labeling for Medical Applications, pages 170–178.
Springer.
Nie, D., Trullo, R., Lian, J., Petitjean, C., Ruan, S., Wang,
Q., and Shen, D. (2017). Medical image synthesis
with context-aware generative adversarial networks.
In International conference on medical image comput-
ing and computer-assisted intervention, pages 417–
425. Springer.
Ollinger, J. M. and Fessler, J. A. (1997). Positron-
emission tomography. Ieee signal processing maga-
zine, 14(1):43–55.
Paans, A. M. (2006). Positron emission tomography.
Paulus, D. H., Quick, H. H., Geppert, C., Fenchel, M.,
Zhan, Y., Hermosillo, G., Faul, D., Boada, F.,
Friedman, K. P., and Koesters, T. (2015). Whole-
body PET/MR imaging: quantitative evaluation of a
novel model-based MR attenuation correction method
including bone. Journal of Nuclear Medicine,
56(7):1061–1066.
Qi, M., Li, Y., Wu, A., Jia, Q., Li, B., Sun, W., Dai, Z., Lu,
X., Zhou, L., Deng, X., et al. (2020). Multi-sequence
MR image-based synthetic CT generation using a gen-
erative adversarial network for head and neck mri-
only radiotherapy. Medical physics, 47(4):1880–1894.
Radford, A., Metz, L., and Chintala, S. (2015). Unsu-
pervised representation learning with deep convolu-
tional generative adversarial networks. arXiv preprint
arXiv:1511.06434.
Ronneberger, O., Fischer, P., and Brox, T. (2015). U-net:
Convolutional networks for biomedical image seg-
mentation. In International Conference on Medical
image computing and computer-assisted intervention,
pages 234–241. Springer.
Torrado-Carvajal, A., Vera-Olmos, J., Izquierdo-Garcia, D.,
Catalano, O. A., Morales, M. A., Margolin, J., Sori-
celli, A., Salvatore, M., Malpica, N., and Catana,
C. (2019). Dixon-VIBE deep learning (DIVIDE)
pseudo-CT synthesis for pelvis PET/MR attenuation
correction. Journal of nuclear medicine, 60(3):429–
435.
Wang, Z., Bovik, A. C., Sheikh, H. R., and Simoncelli, E. P.
(2004). Image quality assessment: from error visi-
bility to structural similarity. IEEE transactions on
image processing, 13(4):600–612.
West, J., Fitzpatrick, J. M., Wang, M. Y., Dawant, B. M.,
Maurer Jr, C. R., Kessler, R. M., Maciunas, R. J., Bar-
illot, C., Lemoine, D., Collignon, A., et al. (1997).
Comparison and evaluation of retrospective inter-
modality brain image registration techniques. Journal
of computer assisted tomography, 21(4):554–568.
Wolterink, J. M., Dinkla, A. M., Savenije, M. H., Seevinck,
P. R., van den Berg, C. A., and I
ˇ
sgum, I. (2017). Deep
mr to ct synthesis using unpaired data. In Interna-
tional Workshop on Simulation and Synthesis in Med-
ical Imaging, pages 14–23. Springer.
Wu, J., Huang, Z., Thoma, J., Acharya, D., and Van Gool, L.
(2018). Wasserstein divergence for gans. In Proceed-
ings of the European Conference on Computer Vision
(ECCV), pages 653–668.
Yaniv, Z., Lowekamp, B. C., Johnson, H. J., and Beare, R.
(2018). SimpleITK image-analysis notebooks: a col-
laborative environment for education and reproducible
research. Journal of digital imaging, 31(3):290–303.
Zhu, J.-Y., Park, T., Isola, P., and Efros, A. A. (2017).
Unpaired image-to-image translation using cycle-
consistent adversarial networks. In Proceedings of
the IEEE international conference on computer vi-
sion, pages 2223–2232.
BIOIMAGING 2022 - 9th International Conference on Bioimaging
140