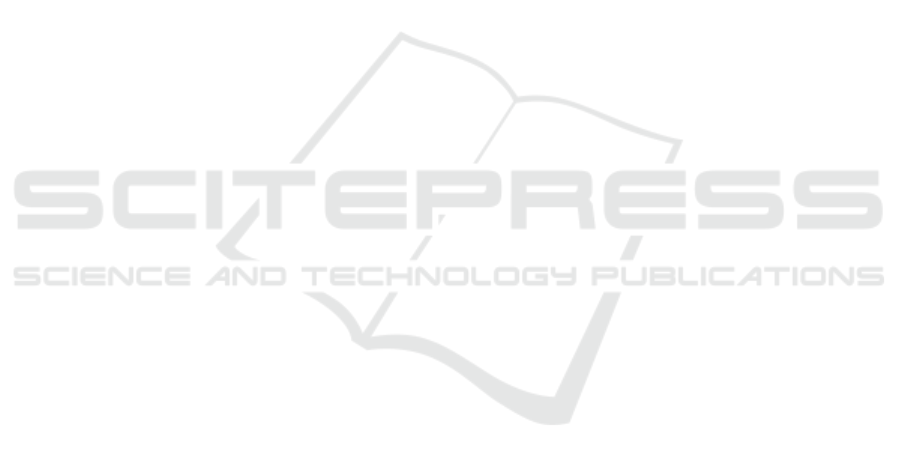
Budai, A., Bock, R., Maier, A., Hornegger, J., and Michel-
son, G. (2013). Robust vessel segmentation in fundus
images. International journal of biomedical imaging,
2013.
Fielder, A. R., Wallace, D. K., Stahl, A., Reynolds, J. D.,
Chiang, M. F., and Quinn, G. E. (2019). Describing
retinopathy of prematurity: Current limitations and
new challenges. Ophthalmology, 126(5):652–654.
Gilbert, C., Malik, A. N., and Vinekar, A. (2021). Artificial
intelligence for rop screening and to assess quality of
care: Progress and challenges. Pediatrics, 147(3).
Guo, C., Szemenyei, M., Yi, Y., Wang, W., Chen, B.,
and Fan, C. (2020a). Sa-unet: Spatial attention u-
net for retinal vessel segmentation. arXiv preprint
arXiv:2004.03696.
Guo, X., Chen, C., Lu, Y., Meng, K., Chen, H., Zhou,
K., Wang, Z., and Xiao, R. (2020b). Retinal ves-
sel segmentation combined with generative adversar-
ial networks and dense u-net. IEEE Access, 8:194551–
194560.
Holm, S., Russell, G., Nourrit, V., and McLoughlin, N.
(2017). Dr hagis—a fundus image database for
the automatic extraction of retinal surface vessels
from diabetic patients. Journal of Medical Imaging,
4(1):014503.
Hoover, A. D., Kouznetsova, V., and Goldbaum, M. (2000).
Locating blood vessels in retinal images by piecewise
threshold probing of a matched filter response. IEEE
Transactions on Medical Imaging, 19(3):203–210.
Isola, P., Zhu, J.-Y., Zhou, T., and Efros, A. A. (2018).
Image-to-image translation with conditional adversar-
ial networks.
Kamran, S. A., Hossain, K. F., Tavakkoli, A., Zuckerbrod,
S. L., Sanders, K. M., and Baker, S. A. (2021). Rv-
gan: Segmenting retinal vascular structure in fundus
photographs using a novel multi-scale generative ad-
versarial network. In International Conference on
Medical Image Computing and Computer-Assisted In-
tervention, pages 34–44. Springer.
Krestanova, A., Kubicek, J., Penhaker, M., and Timkovic,
J. (2020). Premature infant blood vessel segmentation
of retinal images based on hybrid method for the de-
termination of tortuosity. L
´
eka
ˇ
r a technika-Clinician
and Technology, 50(2):49–57.
Kubicek, J., Timkovic, J., Penhaker, M., Oczka, D., Ko-
varova, V., Krestanova, A., Augustynek, M., and
Cerny, M. (2019). Detection and segmentation of reti-
nal lesions in retcam 3 images based on active con-
tours driven by statistical local features. Advances
in Electrical and Electronic Engineering, 17(2):194–
201.
Kumar, V., Patel, H., Paul, K., Surve, A., Azad, S., and
Chawla, R. (2021). Deep learning assisted retinopathy
of prematurity screening technique. In HEALTHINF,
pages 234–243.
Kumar, V. and Paul, K. (2016). mnetra: A fundoscopy
based optometer. In HEALTHINF, pages 83–92.
Li, L., Verma, M., Nakashima, Y., Nagahara, H., and
Kawasaki, R. (2020). Iternet: Retinal image segmen-
tation utilizing structural redundancy in vessel net-
works. In Proceedings of the IEEE/CVF Winter Con-
ference on Applications of Computer Vision, pages
3656–3665.
Luo, Y., Chen, K., Mao, J., Shen, L., and Sun, M. (2020).
A fusion deep convolutional neural network based on
pathological features for diagnosing plus disease in
retinopathy of prematurity. Investigative Ophthalmol-
ogy & Visual Science, 61(7):2017–2017.
Megrabov, E., Jamshidi, A., and Patange, S. Retinel vessel
segmentation using u-net and gans.
Moshfeghi, D. M. and Capone, A. (2018). Economic barri-
ers in retinopathy of prematurity management. Oph-
thalmology Retina, 2(12):1177–1178.
Organization, W. H. et al. (2019). World report on vision.
Technical report, Geneva: World Health Organization.
Patel, T. P., Aaberg, M. T., Paulus, Y. M., Lieu, P.,
Dedania, V. S., Qian, C. X., Besirli, C. G., Mar-
golis, T., Fletcher, D. A., and Kim, T. N. (2019).
Smartphone-based fundus photography for screening
of plus-disease retinopathy of prematurity. Graefe’s
Archive for Clinical and Experimental Ophthalmol-
ogy, 257(11):2579–2585.
Paul, K. and Kumar, V. (2015). Fundus imaging based af-
fordable eye care. In HEALTHINF, pages 634–641.
Ronneberger, O., Fischer, P., and Brox, T. (2015). U-net:
Convolutional networks for biomedical image seg-
mentation.
Staal, J., Abramoff, M., Niemeijer, M., Viergever, M., and
van Ginneken, B. (2004). Ridge based vessel segmen-
tation in color images of the retina. IEEE Transactions
on Medical Imaging, 23(4):501–509.
Uysal, E. S., Bilici, M. S¸., Zaza, B. S.,
¨
Ozgenc¸, M. Y.,
and Boyar, O. (2021). Exploring the limits of data
augmentation for retinal vessel segmentation. arXiv
preprint arXiv:2105.09365.
Wang, Z., Bovik, A. C., Sheikh, H. R., and Simoncelli, E. P.
(2004). Image Quality Assessment: From Error Vis-
ibility to Structural Similarity. IEEE Transactions on
Image Processing, 13(4):600–612.
Wang, Z., She, Q., and Ward, T. E. (2021). Generative
adversarial networks in computer vision: A survey
and taxonomy. ACM Computing Surveys (CSUR),
54(2):1–38.
Yildiz, V. M., Tian, P., Yildiz, I., Brown, J. M., Kalpathy-
Cramer, J., Dy, J., Ioannidis, S., Erdogmus, D.,
Ostmo, S., Kim, S. J., et al. (2020). Plus disease in
retinopathy of prematurity: Convolutional neural net-
work performance using a combined neural network
and feature extraction approach. Translational Vision
Science & Technology, 9(2):10–10.
Improved Blood Vessels Segmentation of Retinal Image of Infants
153