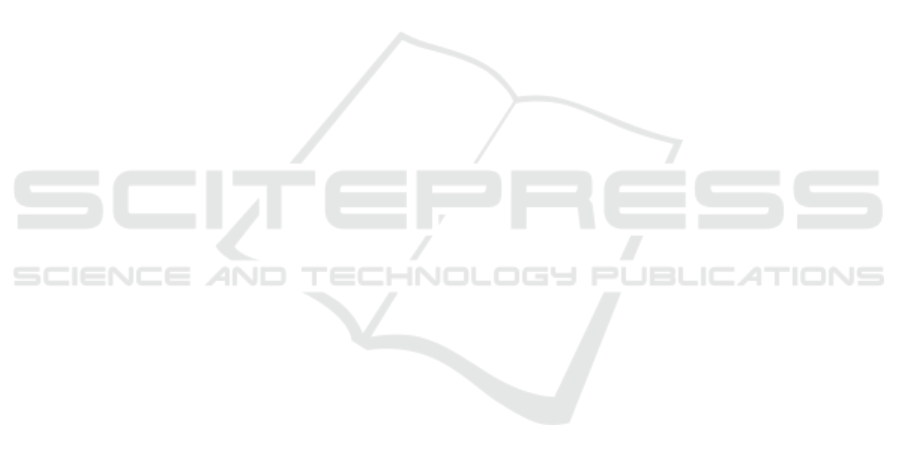
In Proceedings of the IEEE/CVF Conference on Com-
puter Vision and Pattern Recognition (CVPR).
Erfurt, J., Helmrich, C. R., Bosse, S., Schwarz, H., Marpe,
D., and Wiegand, T. (2019). A study of the per-
ceptually weighted peak signal-to-noise ratio (wpsnr)
for image compression. In 2019 IEEE International
Conference on Image Processing (ICIP), pages 2339–
2343.
He, K., Gkioxari, G., Doll
´
ar, P., and Girshick, R. (2017).
Mask r-cnn. In 2017 IEEE International Conference
on Computer Vision (ICCV), pages 2980–2988.
Hu, X., Naiel, M. A., Wong, A., Lamm, M., and Fieguth, P.
(2019). Runet: A robust unet architecture for image
super-resolution. In 2019 IEEE/CVF Conference on
Computer Vision and Pattern Recognition Workshops
(CVPRW), pages 505–507.
Huang, Y., Shao, L., and Frangi, A. F. (2017). Simultane-
ous super-resolution and cross-modality synthesis of
3d medical images using weakly-supervised joint con-
volutional sparse coding. In 2017 IEEE Conference
on Computer Vision and Pattern Recognition (CVPR),
pages 5787–5796.
Isaac, J. S. and Kulkarni, R. (2015). Super resolution tech-
niques for medical image processing. In 2015 Inter-
national Conference on Technologies for Sustainable
Development (ICTSD), pages 1–6.
Jin, S., Xu, L., Xu, J., Wang, C., Liu, W., Qian, C., Ouyang,
W., and Luo, P. (2020). Whole-body human pose
estimation in the wild. In Vedaldi, A., Bischof, H.,
Brox, T., and Frahm, J.-M., editors, Computer Vision
– ECCV 2020, pages 196–214, Cham. Springer Inter-
national Publishing.
Khan, N. U. and Wan, W. (2018). A review of human pose
estimation from single image. In 2018 International
Conference on Audio, Language and Image Process-
ing (ICALIP), pages 230–236.
Ledig, C., Theis, L., Huszar, F., Caballero, J., Cunning-
ham, A., Acosta, A., Aitken, A., Tejani, A., Totz, J.,
Wang, Z., and Shi, W. (2017). Photo-realistic single
image super-resolution using a generative adversarial
network. pages 105–114.
Lin, T., Doll
´
ar, P., Girshick, R., He, K., Hariharan, B., and
Belongie, S. (2017). Feature pyramid networks for
object detection. In 2017 IEEE Conference on Com-
puter Vision and Pattern Recognition (CVPR), pages
936–944.
Lin, T.-Y., Maire, M., Belongie, S., Hays, J., Perona, P., Ra-
manan, D., Doll
´
ar, P., and Zitnick, C. L. (2014). Mi-
crosoft coco: Common objects in context. In Fleet,
D., Pajdla, T., Schiele, B., and Tuytelaars, T., edi-
tors, Computer Vision – ECCV 2014, pages 740–755,
Cham. Springer International Publishing.
Luvizon, D., Tabia, H., and Picard, D. (2020). Multi-task
deep learning for real-time 3d human pose estimation
and action recognition. IEEE transactions on pattern
analysis and machine intelligence.
Na, B. and Fox, G. (2020). Object classifications by image
super-resolution preprocessing for convolutional neu-
ral networks. Advances in Science, Technology and
Engineering Systems Journal, 5:476–483.
Ngiam, J., Chen, Z., Chia, D., Koh, P., Le, Q., and Ng, A.
(2010). Tiled convolutional neural networks. In Laf-
ferty, J., Williams, C., Shawe-Taylor, J., Zemel, R.,
and Culotta, A., editors, Advances in Neural Infor-
mation Processing Systems, volume 23. Curran Asso-
ciates, Inc.
Noord, N. and Postma, E. (2016). Learning scale-variant
and scale-invariant features for deep image classifica-
tion. Pattern Recognition, 61.
Rasti, P., Uiboupin, T., Escalera, S., and Anbarjafari, G.
(2016). Convolutional neural network super resolu-
tion for face recognition in surveillance monitoring.
In Perales, F. J. and Kittler, J., editors, Articulated Mo-
tion and Deformable Objects, pages 175–184, Cham.
Springer International Publishing.
Shermeyer, J. and Etten, A. (2019). The effects of super-
resolution on object detection performance in satellite
imagery. pages 1432–1441.
Sun, K., Xiao, B., Liu, D., and Wang, J. (2019). Deep high-
resolution representation learning for human pose es-
timation. 2019 IEEE/CVF Conference on Computer
Vision and Pattern Recognition (CVPR), pages 5686–
5696.
Takahashi, R., Matsubara, T., and Uehara, K. (2017). Scale-
invariant recognition by weight-shared cnns in paral-
lel. In Zhang, M.-L. and Noh, Y.-K., editors, Proceed-
ings of the Ninth Asian Conference on Machine Learn-
ing, volume 77 of Proceedings of Machine Learning
Research, pages 295–310. PMLR.
Tompson, J. J., Jain, A., LeCun, Y., and Bregler, C. (2014).
Joint training of a convolutional network and a graphi-
cal model for human pose estimation. In Ghahramani,
Z., Welling, M., Cortes, C., Lawrence, N., and Wein-
berger, K. Q., editors, Advances in Neural Information
Processing Systems, volume 27. Curran Associates,
Inc.
Wang, B., Lu, T., and Zhang, Y. (2020). Feature-driven
super-resolution for object detection. In 2020 5th In-
ternational Conference on Control, Robotics and Cy-
bernetics (CRC), pages 211–215.
Wang, X., Yu, K., Wu, S., Gu, J., Liu, Y., Dong, C., Qiao,
Y., and Loy, C. C. (2019). Esrgan: Enhanced super-
resolution generative adversarial networks. In Leal-
Taix
´
e, L. and Roth, S., editors, Computer Vision –
ECCV 2018 Workshops, pages 63–79, Cham. Springer
International Publishing.
Wang, Z., Bovik, A., Sheikh, H., and Simoncelli, E. (2004).
Image quality assessment: from error visibility to
structural similarity. IEEE Transactions on Image
Processing, 13(4):600–612.
Wang, Z., Bovik, A. C., and Lu, L. (2002). Why is image
quality assessment so difficult? In 2002 IEEE Inter-
national Conference on Acoustics, Speech, and Signal
Processing, volume 4, pages IV–3313–IV–3316.
Zhang, K., Gool, L., and Timofte, R. (2020). Deep un-
folding network for image super-resolution. 2020
IEEE/CVF Conference on Computer Vision and Pat-
tern Recognition (CVPR), pages 3214–3223.
Zhang, L., Zhang, H., Shen, H., and Li, P. (2010). A
super-resolution reconstruction algorithm for surveil-
lance images. Signal Processing, 90(3):848–859.
Zhang, L., Zhang, L., Mou, X., and Zhang, D. (2011).
Fsim: A feature similarity index for image quality as-
sessment. Image Processing, IEEE Transactions on,
20:2378 – 2386.
Can Super Resolution Improve Human Pose Estimation in Low Resolution Scenarios?
501