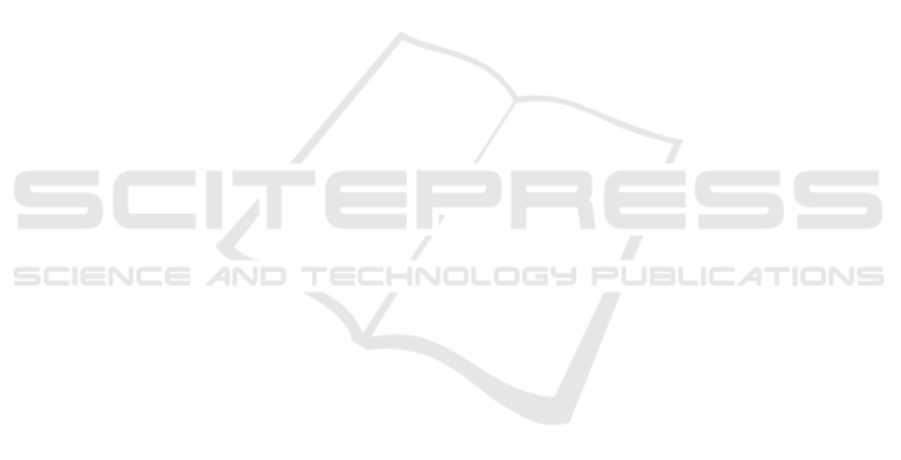
de Tournemire, P., Nitti, D., Perot, E., Migliore, D., and
Sironi, A. (2020). A large scale event-based detection
dataset for automotive. arXiv, abs/2001.08499.
Durkee, M. S., Abraham, R., Ai, J., Fuhrman, J. D., Clark,
M. R., and Giger, M. L. (2021). Comparing Mask
R-CNN and U-Net architectures for robust automatic
segmentation of immune cells in immunofluorescence
images of lupus nephritis biopsies. In Imaging, Ma-
nipulation, and Analysis of Biomolecules, Cells, and
Tissues XIX, volume 11647, page 116470X. Interna-
tional Society for Optics and Photonics.
Fan, H. and Yang, Y. (2019). PointRNN: Point recurrent
neural network for moving point cloud processing.
arXiv, 1910.08287.
Gallego, G., Delbruck, T., Orchard, G. M., Bartolozzi,
C., Taba, B., Censi, A., Leutenegger, S., Davison,
A., Conradt, J., Daniilidis, K., and Scaramuzza, D.
(2020). Event-based vision: A survey. IEEE Transac-
tions on Pattern Analysis and Machine Intelligence.
Guo, M., Huang, J., and Chen, S. (2017). Live demon-
stration: A 768 × 640 pixels 200meps dynamic vi-
sion sensor. In 2017 IEEE International Symposium
on Circuits and Systems (ISCAS), pages 1–1.
Guo, Y., Wang, H., Hu, Q., Liu, H., Liu, L., and Ben-
namoun, M. (2021). Deep learning for 3d point
clouds: A survey. IEEE Transactions on Pattern Anal-
ysis and Machine Intelligence, 43(12):4338–4364.
He, K., Gkioxari, G., Dollar, P., and Girshick, R. (2017).
Mask R-CNN. In Proceedings of the IEEE Interna-
tional Conference on Computer Vision (ICCV).
Hu, Y., Binas, J., Neil, D., Liu, S.-C., and Delbruck, T.
(2020). DDD20 end-to-end event camera driving
dataset: Fusing frames and events with deep learn-
ing for improved steering prediction. In 2020 IEEE
23rd International Conference on Intelligent Trans-
portation Systems (ITSC), pages 1–6.
Jiang, Z., Xia, P., Huang, K., Stechele, W., Chen, G., Bing,
Z., and Knoll, A. (2019). Mixed frame-/event-driven
fast pedestrian detection. In 2019 International Con-
ference on Robotics and Automation (ICRA), pages
8332–8338.
Komarichev, A., Zhong, Z., and Hua, J. (2019). A-CNN:
Annularly convolutional neural networks on point
clouds. In Proceedings of the IEEE/CVF Conference
on Computer Vision and Pattern Recognition (CVPR),
pages 7421–7430.
Miao, S., Chen, G., Ning, X., Zi, Y., Ren, K., Bing, Z.,
and Knoll, A. (2019). Neuromorphic vision datasets
for pedestrian detection, action recognition, and fall
detection. Frontiers in Neurorobotics, 13:38.
Min, Y., Zhang, Y., Chai, X., and Chen, X. (2020). An
efficient PointLSTM for point clouds based gesture
recognition. In Proceedings of the IEEE/CVF Con-
ference on Computer Vision and Pattern Recognition
(CVPR).
Qi, C. R., Su, H., Mo, K., and Guibas, L. J. (2017a). Point-
Net: Deep learning on point sets for 3d classification
and segmentation. In 2017 IEEE Conference on Com-
puter Vision and Pattern Recognition (CVPR), pages
77–85.
Qi, C. R., Yi, L., Su, H., and Guibas, L. J. (2017b). Point-
Net++: Deep hierarchical feature learning on point
sets in a metric space. In Proceedings of the 31st Inter-
national Conference on Neural Information Process-
ing Systems, NIPS’17, pages 5105–5114, Red Hook,
NY, USA. Curran Associates Inc.
Quoc, T. T. P., Linh, T. T., and Minh, T. N. T. (2020).
Comparing U-Net convolutional network with Mask
R-CNN in agricultural area segmentation on satellite
images. In 2020 7th NAFOSTED Conference on In-
formation and Computer Science (NICS), pages 124–
129.
Redmon, J., Divvala, S., Girshick, R., and Farhadi, A.
(2016). You only look once: Unified, real-time ob-
ject detection. In 2016 IEEE Conference on Computer
Vision and Pattern Recognition (CVPR), pages 779–
788.
Ren, S., He, K., Girshick, R., and Sun, J. (2017). Faster
R-CNN: Towards real-time object detection with re-
gion proposal networks. IEEE Transactions on Pat-
tern Analysis and Machine Intelligence, 39(6):1137–
1149.
Ronneberger, O., Fischer, P., and Brox, T. (2015). U-
Net: Convolutional networks for biomedical image
segmentation. In Navab, N., Hornegger, J., Wells,
W. M., and Frangi, A. F., editors, Medical Image Com-
puting and Computer-Assisted Intervention – MICCAI
2015, pages 234–241, Cham. Springer International
Publishing.
Vuola, A. O., Akram, S. U., and Kannala, J. (2019). Mask-
RCNN and U-Net ensembled for nuclei segmenta-
tion. In 2019 IEEE 16th International Symposium on
Biomedical Imaging (ISBI 2019), pages 208–212.
Wan, J., Xia, M., Huang, Z., Tian, L., Zheng, X., Chang, V.,
Zhu, Y., and Wang, H. (2021). Event-based pedestrian
detection using dynamic vision sensors. Electronics,
10(8).
Wang, Q., Zhang, Y., Yuan, J., and Lu, Y. (2019). Space-
time event clouds for gesture recognition: From RGB
cameras to event cameras. In 2019 IEEE Winter Con-
ference on Applications of Computer Vision (WACV),
pages 1826–1835.
Wang, Y., Zhang, X., Shen, Y., Du, B., Zhao, G.,
Cui Lizhen, L. C., and Wen, H. (2021). Event-
stream representation for human gaits identification
using deep neural networks. IEEE Transactions on
Pattern Analysis and Machine Intelligence.
Xu, Y., Fan, T., Xu, M., Zeng, L., and Qiao, Y. (2018). Spi-
derCNN: Deep learning on point sets with parameter-
ized convolutional filters. In Ferrari, V., Hebert, M.,
Sminchisescu, C., and Weiss, Y., editors, Computer
Vision – ECCV 2018, pages 90–105, Cham. Springer
International Publishing.
Zhao, T., Yang, Y., Niu, H., Wang, D., and Chen, Y.
(2018). Comparing U-Net convolutional network with
Mask R-CNN in the performances of pomegranate
tree canopy segmentation. In Multispectral, Hyper-
spectral, and Ultraspectral Remote Sensing Technol-
ogy, Techniques and Applications VII, volume SPIE
10780, page 107801J. International Society for Optics
and Photonics.
VISAPP 2022 - 17th International Conference on Computer Vision Theory and Applications
178