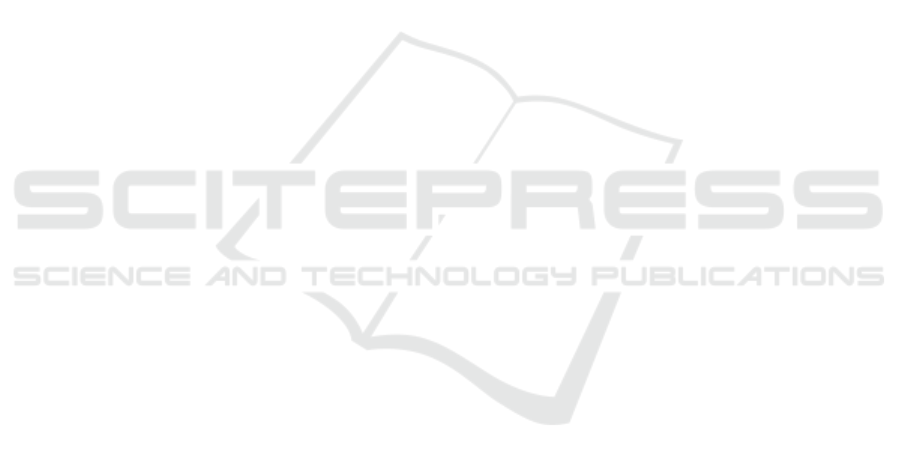
vision-based pose estimation at city scales. In 2013
IEEE international conference on robotics and au-
tomation, pages 3762–3769. IEEE.
Milioto, A., Vizzo, I., Behley, J., and Stachniss, C. (2019).
RangeNet ++: Fast and Accurate LiDAR Semantic
Segmentation. In 2019 IEEE/RSJ International Con-
ference on Intelligent Robots and Systems (IROS) ,
pages 4213–4220. IEEE.
Mohapatra, S., Yogamani, S., Gotzig, H., Milz, S., and
Mader, P. (2021). BEVDetNet: Bird’s Eye View Li-
DAR Point Cloud based Real-time 3D Object De-
tection for Autonomous Driving. arXiv preprint
arXiv:2104.10780.
Patil, P. W., Biradar, K. M., Dudhane, A., and Murala,
S. (2020). An End-to-End Edge Aggregation Net-
work for Moving Object Segmentation. In proceed-
ings of the IEEE/CVF conference on computer vision
and pattern recognition, pages 8149–8158.
Postica, G., Romanoni, A., and Matteucci, M. (2016). Ro-
bust Moving Objects Detection in Lidar Data Exploit-
ing Visual Cues. In 2016 IEEE/RSJ International
Conference on Intelligent Robots and Systems (IROS),
pages 1093–1098. IEEE.
Rashed, H., Mohamed, E., Sistu, G., Ravi Kumar, V., Eis-
ing, C., El-Sallab, A., and Yogamani, S. (2021). Gen-
eralized Object Detection on Fisheye Cameras for
Autonomous Driving: Dataset, Representations and
Baseline. In Proceedings of the Winter Conference on
Applications of Computer Vision, pages 2272–2280.
Rashed, H., Ramzy, M., Vaquero, V., El Sallab, A., Sistu,
G., and Yogamani, S. (2019). FuseMODNet: Real-
Time Camera and LiDAR based Moving Object De-
tection for robust low-light Autonomous Driving. In
Proceedings of the IEEE/CVF International Confer-
ence on Computer Vision Workshops, pages 0–0.
Ravi Kumar, V., Klingner, M., Yogamani, S., Milz, S., Fin-
gscheidt, T., and Mader, P. (2021a). Syndistnet: Self-
supervised monocular fisheye camera distance estima-
tion synergized with semantic segmentation for au-
tonomous driving. In Proceedings of the IEEE/CVF
Winter Conference on Applications of Computer Vi-
sion, pages 61–71.
Ravi Kumar, V., Milz, S., Witt, C., and Yogamani, S.
(2018). Near-field depth estimation using monocu-
lar fisheye camera: A semi-supervised learning ap-
proach using sparse LiDAR data. In Proceedings of
the IEEE/CVF Conference on Computer Vision and
Pattern Recognition (CVPR) Workshop, volume 7.
Ravi Kumar, V., Yogamani, S., Rashed, H., Sitsu, G., Witt,
C., Leang, I., Milz, S., and M
¨
ader, P. (2021b). Om-
nidet: Surround view cameras based multi-task visual
perception network for autonomous driving. IEEE
Robotics and Automation Letters, 6(2):2830–2837.
Shi, H., Lin, G., Wang, H., Hung, T.-Y., and Wang, Z.
(2020). SpSequenceNet: Semantic Segmentation Net-
work on 4D Point Clouds. In Proceedings of the
IEEE/CVF Conference on Computer Vision and Pat-
tern Recognition, pages 4574–4583.
Sobh, I., Hamed, A., Kumar, V. R., and Yogamani, S.
(2021). Adversarial attacks on multi-task visual
perception for autonomous driving. arXiv preprint
arXiv:2107.07449.
Steinhauser, D., Ruepp, O., and Burschka, D. (2008). Mo-
tion segmentation and scene classification from 3D
LIDAR data. In 2008 IEEE intelligent vehicles sym-
posium, pages 398–403. IEEE.
Uricar, M., Sistu, G., Rashed, H., Vobecky, A., Ravi Kumar,
V., Krizek, P., Burger, F., and Yogamani, S. (2021).
Let’s get dirty: Gan based data augmentation for cam-
era lens soiling detection in autonomous driving. In
Proceedings of the IEEE/CVF Winter Conference on
Applications of Computer Vision, pages 766–775.
Vaquero, V., Del Pino, I., Moreno-Noguer, F., Sola, J., San-
feliu, A., and Andrade-Cetto, J. (2017). Deconvolu-
tional networks for point-cloud vehicle detection and
tracking in driving scenarios. In 2017 European Con-
ference on Mobile Robots (ECMR), pages 1–7. IEEE.
Varun, R. K., Klingner, M., Yogamani, S., Bach, M., Milz,
S., Fingscheidt, T., and M
¨
ader, P. (2021a). SVDist-
Net: Self-supervised near-field distance estimation on
surround view fisheye cameras. IEEE Transactions on
Intelligent Transportation Systems.
Varun, R. K., Yogamani, S., Bach, M., Witt, C., Milz,
S., and M
¨
ader, P. (2020). UnRectDepthNet: Self-
Supervised Monocular Depth Estimation using a
Generic Framework for Handling Common Camera
Distortion Models. In IEEE/RSJ International Con-
ference on Intelligent Robots and Systems, IROS.
Varun, R. K., Yogamani, S., Milz, S., and M
¨
ader, P. (2021b).
FisheyeDistanceNet++: Self-Supervised Fisheye Dis-
tance Estimation with Self-Attention, Robust Loss
Function and Camera View Generalization. In Elec-
tronic Imaging.
Wang, D. Z., Posner, I., and Newman, P. (2012). What could
move? Finding cars, pedestrians and bicyclists in 3D
laser data. In 2012 IEEE International Conference on
Robotics and Automation, pages 4038–4044. IEEE.
Wu, B., Wan, A., Yue, X., and Keutzer, K. (2018). Squeeze-
Seg: Convolutional Neural Nets with Recurrent CRF
for Real-Time Road-Object Segmentation from 3D
LiDAR Point Cloud. In 2018 IEEE International Con-
ference on Robotics and Automation (ICRA). IEEE.
Wu, B., Zhou, X., Zhao, S., Yue, X., and Keutzer, K. (2019).
SqueezeSegV2: Improved Model Structure and Un-
supervised Domain Adaptation for Road-Object Seg-
mentation from a LiDAR Point Cloud. In 2019 In-
ternational Conference on Robotics and Automation
(ICRA), pages 4376–4382. IEEE.
Yahiaoui, M., Rashed, H., Mariotti, L., Sistu, G., Clancy,
I., Yahiaoui, L., Ravi Kumar, V., and Yogamani, S.
(2019). FisheyeModNet: Moving object detection
on Surround-View Cameras for Autonomous Driving.
arXiv preprint arXiv:1908.11789.
Yan, J., Chen, D., Myeong, H., Shiratori, T., and Ma, Y.
(2014). Automatic Extraction of Moving Objects from
Image and LIDAR Sequences. In 2014 2nd Interna-
tional Conference on 3D Vision, volume 1. IEEE.
Yan, Y., Mao, Y., and Li, B. (2018). Second:
Sparsely embedded convolutional detection. Sensors,
18(10):3337.
Yoon, D., Tang, T., and Barfoot, T. (2019). Mapless On-
line Detection of Dynamic Objects in 3D Lidar. In
2019 16th Conference on Computer and Robot Vision
(CRV), pages 113–120. IEEE.
LiMoSeg: Real-time Bird’s Eye View based LiDAR Motion Segmentation
835