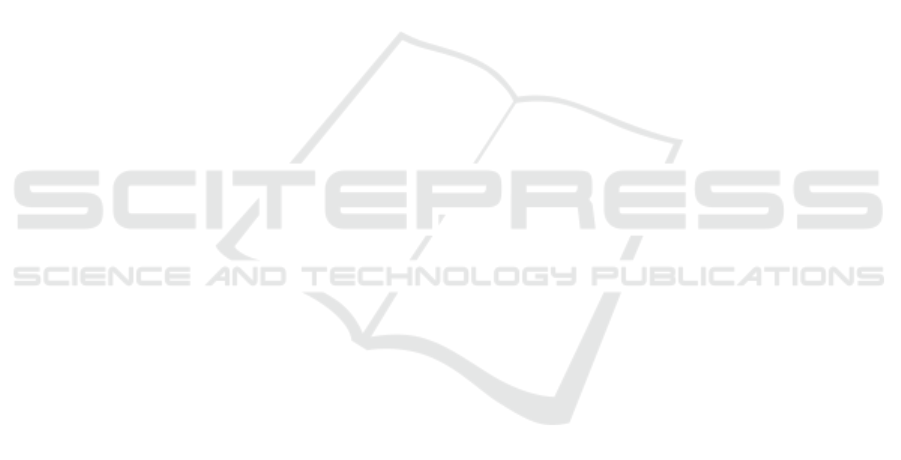
ACKNOWLEDGEMENTS
We use publicly-available datasets. Hanyuan Wang
is funded by the China Scholarship Council. Toby
Perrett is funded by the SPHERE Next Steps EPSRC
Project EP/R005273/1.
REFERENCES
Bai, Y., Wang, Y., Tong, Y., Yang, Y., Liu, Q., and Liu,
J. (2020). Boundary content graph neural network
for temporal action proposal generation. In European
Conference on Computer Vision.
Bodla, N., Singh, B., Chellappa, R., and Davis, L. S. (2017).
Soft-nms improving object detection with one line of
code. In International Conference on Computer Vi-
sion.
Caba Heilbron, F., Escorcia, V., Ghanem, B., and Car-
los Niebles, J. (2015). ActivityNet: A large-scale
video benchmark for human activity understanding. In
Computer Vision and Pattern Recognition.
Carreira, J. and Zisserman, A. (2017). Quo vadis, action
recognition? a new model and the Kinetics dataset. In
Computer Vision and Pattern Recognition.
Chao, Y.-W., Vijayanarasimhan, S., Seybold, B., Ross,
D. A., Deng, J., and Sukthankar, R. (2018). Rethink-
ing the faster r-cnn architecture for temporal action lo-
calization. In Computer Vision and Pattern Recogni-
tion.
Chen, Y., Chen, M., Wu, R., Zhu, J., Zhu, Z., Gu, Q., and
Robotics, H. (2020). Refinement of boundary regres-
sion using uncertainty in temporal action localization.
In British Machine Vision Conference.
Cioppa, A., Deli
`
ege, A., Giancola, S., Ghanem, B.,
Droogenbroeck, M. V., Gade, R., and Moeslund, T. B.
(2020). A context-aware loss function for action spot-
ting in soccer videos. In Computer Vision and Pattern
Recognition.
Feichtenhofer, C., Fan, H., Malik, J., and He, K. (2019).
SlowFast networks for video recognition. In Interna-
tional Conference on Computer Vision.
Heidarivincheh, F., Mirmehdi, M., and Damen, D. (2018).
Action completion: A temporal model for moment de-
tection. In British Machine Vision Conference.
Heidarivincheh, F., Mirmehdi, M., and Damen, D. (2019).
Weakly-supervised completion moment detection us-
ing temporal attention. In International Conference
on Computer Vision Workshop.
Jiang, Y., Liu, J., Zamir, A. R., Toderici, G., Laptev, I.,
Shah, M., and Sukthankar, R. (2014). THUMOS
challenge: Action recognition with a large number of
classes. In European Conference on Computer Vision
Workshop.
Kingma, D. and Ba, J. (2014). Adam: A method for
stochastic optimization. In International Conference
on Learning Representations.
Lin, C., Li, J., Wang, Y., Tai, Y., Luo, D., Cui, Z., Wang, C.,
Li, J., Huang, F., and Ji, R. (2020). Fast learning of
temporal action proposal via dense boundary genera-
tor. In AAAI Conference on Artificial Intelligence.
Lin, T., Liu, X., Li, X., Ding, E., and Wen, S. (2019).
BMN: Boundary-matching network for temporal ac-
tion proposal generation. In International Conference
on Computer Vision.
Lin, T., Zhao, X., Su, H., Wang, C., and Yang, M. (2018).
BSN: Boundary sensitive network for temporal ac-
tion proposal generation. In European Conference on
Computer Vision.
Liu, X., Hu, Y., Bai, S., Ding, F., Bai, X., and Torr, P. H.
(2021). Multi-shot temporal event localization: a
benchmark. In Computer Vision and Pattern Recog-
nition (CVPR).
Liu, Y., Ma, L., Zhang, Y., Liu, W., and Chang, S.-F. (2019).
Multi-granularity generator for temporal action pro-
posal. In Computer Vision and Pattern Recognition.
Long, F., Yao, T., Qiu, Z., Tian, X., Luo, J., and Mei, T.
(2019). Gaussian temporal awareness networks for
action localization. In Computer Vision and Pattern
Recognition.
Paul, S., Roy, S., and Roy-Chowdhury, A. K. (2018). W-
TALC: Weakly-supervised temporal activity localiza-
tion and classification. In European Conference on
Computer Vision.
Simonyan, K. and Zisserman, A. (2014). Two-stream con-
volutional networks for action recognition in videos.
In Neural Information Processing Systems.
Su, H., Gan, W., Wu, W., Yan, J., and Qiao, Y. (2021).
BSN++: Complementary boundary regressor with
scale-balanced relation modeling for temporal action
proposal generation. In AAAI Conference on Artificial
Intelligence.
Tran, D., Bourdev, L., Fergus, R., Torresani, L., and Paluri,
M. (2015). Learning spatiotemporal features with 3d
convolutional networks. In International Conference
on Computer Vision.
Wang, L., Xiong, Y., Lin, D., and Van Gool, L. (2017).
UntrimmedNets for weakly supervised action recog-
nition and detection. In Computer Vision and Pattern
Recognition.
Xiong, Y., Wang, L., Wang, Z., Zhang, B., Song, H., Li, W.,
Lin, D., Qiao, Y., Gool, L. V., and Tang, X. (2016).
CUHK & ETHZ & SIAT submission to activitynet
challenge 2016.
Xu, M., Zhao, C., Rojas, D. S., Thabet, A., and Ghanem,
B. (2020). G-TAD: Sub-graph localization for tempo-
ral action detection. In Computer Vision and Pattern
Recognition.
Zacks, J., Braver, T., Sheridan, M., Donaldson, D., Snyder,
A., Ollinger, J., Buckner, R., and Raichle, M. (2001).
Human brain activity time-locked to perceptual event
boundaries. Nature Neuroscience, 4:651–655.
Zeng, R., Huang, W., Tan, M., Rong, Y., Zhao, P., Huang,
J., and Gan, C. (2019). Graph convolutional networks
for temporal action localization. In International Con-
ference on Computer Vision.
Zhao, Y., Xiong, Y., Wang, L., Wu, Z., Tang, X., and Lin, D.
(2017). Temporal action detection with structured seg-
ment networks. In International Conference on Com-
puter Vision.
VISAPP 2022 - 17th International Conference on Computer Vision Theory and Applications
558