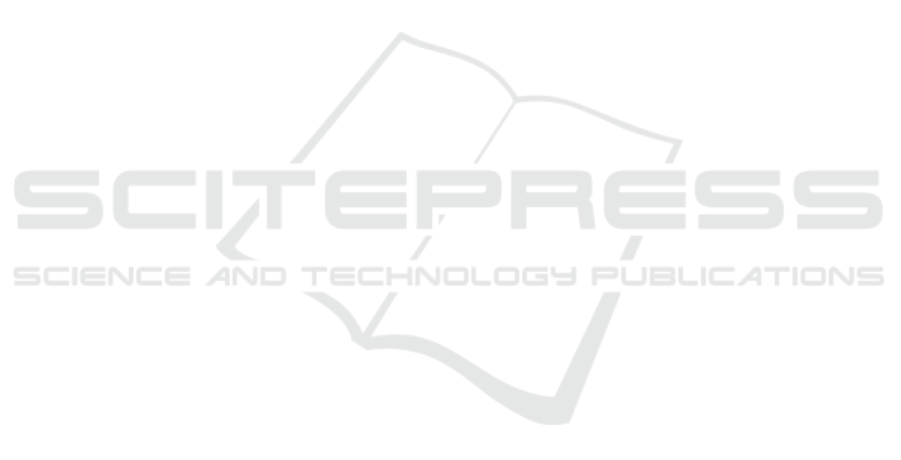
teractive and passive classes (reaching 95.9%) in or-
der to determine whether someone is trying to interact
with the robot or not.
The results show that, with our approach, gesture
recognition with high classification rates is possible
for important subtasks in HRI. On the full classifica-
tion issue of 20 classes, our method achieves 70%.
A different joint constellation could improve results
on classes that rely on finger joints, which are not in-
cluded in the dataset.
Further enhancement of gesture recognition is
possible. Extending the dataset or creating algo-
rithms which achieve higher accuracy on the full HRI-
Gestures dataset could be considered.
ACKNOWLEDGEMENTS
This research was supported by the HanDiRob
project, funded by the European Fund for regional
development, and by the DIREC project, funded by
Innovation Fund Denmark.
REFERENCES
Bodenhagen, L., Suvei, S.-D., Juel, W. K., Brander, E.,
and Kr
¨
uger, N. (2019). Robot technology for future
welfare: meeting upcoming societal challenges – an
outlook with offset in the development in scandinavia.
Health and Technology, 9:197–218.
Cao, Z., Hidalgo Martinez, G., Simon, T., Wei, S., and
Sheikh, Y. A. (2019). Openpose: Realtime multi-
person 2d pose estimation using part affinity fields.
IEEE Transactions on Pattern Analysis and Machine
Intelligence.
Chatterjee, S., Zunjani, F. H., and Nandi, G. C. (2020).
Real-time object detection and recognition on low-
compute humanoid robots using deep learning. In
2020 6th International Conference on Control, Au-
tomation and Robotics (ICCAR), pages 202–208.
Hull, R. (2016). The art of nonverbal communication in
practice. The Hearing Journal.
Juel., W., Haarslev., F., Kr
¨
uger., N., and Bodenhagen., L.
(2020). An integrated object detection and tracking
framework for mobile robots. In Proceedings of the
17th International Conference on Informatics in Con-
trol, Automation and Robotics (ICINCO).
Kay, W., Carreira, J., Simonyan, K., Zhang, B., Hillier, C.,
Vijayanarasimhan, S., Viola, F., Green, T., Back, T.,
Natsev, A., Suleyman, M., and Zisserman, A. (2017).
The kinetics human action video dataset. ArXiv,
abs/1705.06950.
Kr
¨
uger, N., Fischer, K., Manoonpong, P., Palinko, O., Bo-
denhagen, L., Baumann, T., Kjærum, J., Rano, I.,
Naik, L., Juel, W., Haarslev, F., Ignasov, J., Marchetti,
E., Langedijk, R., Kollakidou, A., Camillus Jeppesen,
K., Heidtmann, C., and Dalgaard, L. (2021). The
smooth-robot: A modular, interactive service robot.
Frontiers in Robotics and AI, 8.
Lin, T.-Y., Maire, M., Belongie, S., Hays, J., Perona, P.,
Ramanan, D., Doll
´
ar, P., and Zitnick, C. L. (2014).
Microsoft coco: Common objects in context. In Fleet,
D., Pajdla, T., Schiele, B., and Tuytelaars, T., editors,
Computer Vision – ECCV 2014, pages 740–755.
Liu, H., Tu, J., and Liu, M. (2017). Two-stream 3d convolu-
tional neural network for skeleton-based action recog-
nition. ArXiv, abs/1904.07850.
Liu, J., Shahroudy, A., Perez, M., Wang, G., Duan, L.-Y.,
and Kot, A. C. (2020a). Ntu rgb+d 120: A large-
scale benchmark for 3d human activity understanding.
IEEE Transactions on Pattern Analysis and Machine
Intelligence, 42(10):2684–2701.
Liu, Z., Zhang, H., Chen, Z., Wang, Z., and Ouyang, W.
(2020b). Disentangling and unifying graph convo-
lutions for skeleton-based action recognition. 2020
IEEE/CVF Conference on Computer Vision and Pat-
tern Recognition (CVPR), pages 140–149.
McNally, W. J., Vats, K., Wong, A., and McPhee, J.
(2020). Evopose2d: Pushing the boundaries of 2d
human pose estimation using neuroevolution. ArXiv,
abs/2011.08446.
Palinko, O., Ram
´
ırez, E., Juel, W., Kr
¨
uger, N., and Bo-
denhagen, L. (2020). Intention indication for human
aware robot navigation. In Proceedings of the 15th
International Joint Conference on Computer Vision,
Imaging and Computer Graphics Theory and Appli-
cations - Volume 2: HUCAPP.
Song, Y.-F., Zhang, Z., Shan, C., and Wang, L. (2020).
Stronger, faster and more explainable: A graph con-
volutional baseline for skeleton-based action recogni-
tion. In Proceedings of the 28th ACM International
Conference on Multimedia (ACMMM).
Song, Y.-F., Zhang, Z., Shan, C., and Wang, L. (2021).
Richly activated graph convolutional network for ro-
bust skeleton-based action recognition. IEEE Trans-
actions on Circuits and Systems for Video Technology,
31(5):1915–1925.
Song, Y.-F., Zhang, Z., and Wang, L. (2019). Richly acti-
vated graph convolutional network for action recogni-
tion with incomplete skeletons. In International Con-
ference on Image Processing (ICIP). IEEE.
Tada, Y., Hagiwara, Y., Tanaka, H., and Taniguchi, T.
(2020). Robust understanding of robot-directed
speech commands using sequence to sequence with
noise injection. Frontiers in Robotics and AI, 6:144.
Thakkar, K. C. and Narayanan, P. (2018). Part-based graph
convolutional network for action recognition. ArXiv,
abs/1809.04983.
Yan, S., Xiong, Y., and Lin, D. (2018). Spatial temporal
graph convolutional networks for skeleton-based ac-
tion recognition. ArXiv, abs/1801.07455.
Zhou, X., Wang, D., and Kr
¨
ahenb
¨
uhl, P. (2019). Objects as
points. ArXiv, abs/1904.07850.
VISAPP 2022 - 17th International Conference on Computer Vision Theory and Applications
566