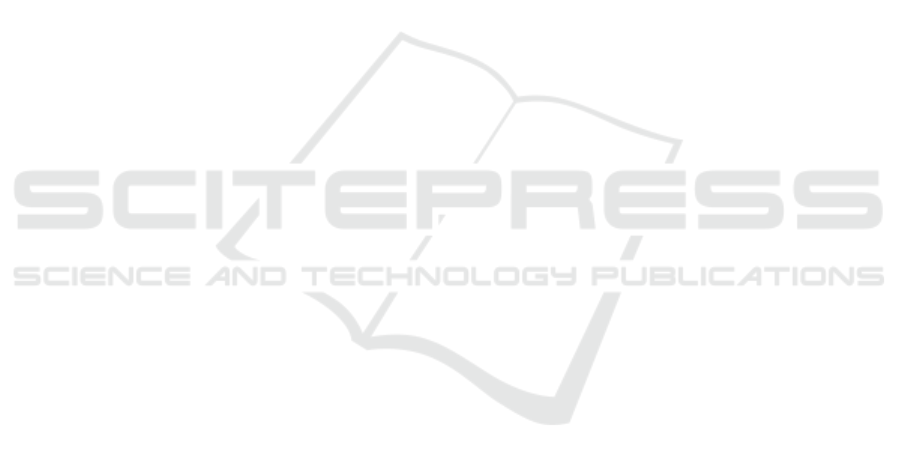
Kuo, C. and Nevatia, R. (2011). How does person iden-
tity recognition help multi-person tracking? In CVPR
2011, pages 1217–1224.
Leal-Taix
´
e, L., Milan, A., Reid, I., Roth, S., and Schindler,
K. (2015). MOTChallenge 2015: Towards a bench-
mark for multi-target tracking. arXiv:1504.01942
[cs]. arXiv: 1504.01942.
Lenz, P., Geiger, A., and Urtasun, R. (2014). Followme:
Efficient online min-cost flow tracking with bounded
memory and computation.
Li, J., Wang, J., Tian, Q., Gao, W., and Zhang, S. (2019).
Global-local temporal representations for video per-
son re-identification. In Proceedings of the IEEE
International Conference on Computer Vision, pages
3958–3967.
Li Zhang, Yuan Li, and Nevatia, R. (2008). Global data
association for multi-object tracking using network
flows. In 2008 IEEE Conference on Computer Vision
and Pattern Recognition, pages 1–8.
Lin, T.-Y., Doll
´
ar, P., Girshick, R., He, K., Hariharan, B.,
and Belongie, S. (2017). Feature pyramid networks
for object detection. In Proceedings of the IEEE con-
ference on computer vision and pattern recognition,
pages 2117–2125.
Liu, M., Zhu, M., White, M., Li, Y., and Kalenichenko,
D. (2019). Looking fast and slow: Memory-
guided mobile video object detection. arXiv preprint
arXiv:1903.10172.
Mahadevan, S., Athar, A., O
ˇ
sep, A., Hennen, S., Leal-
Taix
´
e, L., and Leibe, B. (2020). Making a case for
3d convolutions for object segmentation in videos. In
BMVC.
Milan, A., Leal-Taix
´
e, L., Reid, I., Roth, S., and Schindler,
K. (2016). MOT16: A benchmark for multi-object
tracking. arXiv:1603.00831 [cs]. arXiv: 1603.00831.
Milan, A., Leal-Taix
´
e, L., Schindler, K., and Reid, I. (2015).
Joint tracking and segmentation of multiple targets.
2015 IEEE Conference on Computer Vision and Pat-
tern Recognition (CVPR), pages 5397–5406.
Osep, A., Mehner, W., Voigtlaender, P., and Leibe, B.
(2018). Track, then decide: Category-agnostic vision-
based multi-object tracking. pages 1–8.
Pang, B., Li, Y., Zhang, Y., Li, M., and Lu, C. (2020). Tu-
betk: Adopting tubes to track multi-object in a one-
step training model. In CVPR.
Paszke, A., Gross, S., Chintala, S., Chanan, G., Yang, E.,
DeVito, Z., Lin, Z., Desmaison, A., Antiga, L., and
Lerer, A. (2017). Automatic differentiation in pytorch.
Pirsiavash, H., Ramanan, D., and Fowlkes, C. (2011).
Globally-optimal greedy algorithms for tracking a
variable number of objects. pages 1201 – 1208.
Ren, S., He, K., Girshick, R., and Sun, J. (2015). Faster
r-cnn: Towards real-time object detection with region
proposal networks. In Advances in neural information
processing systems, pages 91–99.
Ristani, E., Solera, F., Zou, R., Cucchiara, R., and Tomasi,
C. (2016). Performance measures and a data set for
multi-target, multi-camera tracking.
Ristani, E. and Tomasi, C. (2018). Features for multi-target
multi-camera tracking and re-identification. In 2018
IEEE/CVF Conference on Computer Vision and Pat-
tern Recognition, pages 6036–6046.
Sadeghian, A., Alahi, A., and Savarese, S. (2017). Tracking
the untrackable: Learning to track multiple cues with
long-term dependencies. pages 300–311.
Sun, S., Akhtar, N., Song, X., Song, H., Mian, A., and Shah,
M. (2020). Simultaneous detection and tracking with
motion modelling for multiple object tracking. ECCV.
Tang, S., Andriluka, M., Andres, B., and Schiele, B. (2017).
Multiple people tracking by lifted multicut and person
re-identification. In 2017 IEEE Conference on Com-
puter Vision and Pattern Recognition (CVPR), pages
3701–3710.
Tian, Z., Shen, C., Chen, H., and He, T. (2019). Fcos: Fully
convolutional one-stage object detection. In Proceed-
ings of the IEEE international conference on com-
puter vision, pages 9627–9636.
Tran, D., Wang, H., Torresani, L., Ray, J., LeCun, Y.,
and Paluri, M. (2018). A closer look at spatiotem-
poral convolutions for action recognition. In 2018
IEEE/CVF Conference on Computer Vision and Pat-
tern Recognition, pages 6450–6459.
Veli
ˇ
ckovi
´
c, P., Cucurull, G., Casanova, A., Romero, A., Lio,
P., and Bengio, Y. (2017). Graph attention networks.
arXiv preprint arXiv:1710.10903.
Voigtlaender, P., Krause, M., Osep, A., Luiten, J., Sekar, B.
B. G., Geiger, A., and Leibe, B. (2019). Mots: Multi-
object tracking and segmentation. In 2019 IEEE/CVF
Conference on Computer Vision and Pattern Recogni-
tion (CVPR), pages 7934–7943.
Wang, X., Xiao, T., Jiang, Y., Shao, S., Sun, J., and Shen,
C. (2018). Repulsion loss: Detecting pedestrians in
a crowd. In Proceedings of the IEEE Conference
on Computer Vision and Pattern Recognition, pages
7774–7783.
Wang, Y., Weng, X., and Kitani, K. (2020). Joint detection
and multi-object tracking with graph neural networks.
arXiv preprint arXiv:2006.13164.
Wang, Z., Zheng, L., Liu, Y., and Wang, S. (2019). To-
wards real-time multi-object tracking. arXiv preprint
arXiv:1909.12605.
Xiu, Y., Li, J., Wang, H., Fang, Y., and Lu, C. (2018). Pose
Flow: Efficient online pose tracking. In BMVC.
Xu, Y. and Wang, J. (2019). A unified neural network for
object detection, multiple object tracking and vehicle
re-identification. ArXiv, abs/1907.03465.
Yu, F., Koltun, V., and Funkhouser, T. (2017). Dilated
residual networks. In Proceedings of the IEEE con-
ference on computer vision and pattern recognition,
pages 472–480.
Zhu, J., Yang, H., Liu, N., Kim, M., Zhang, W., and Yang,
M.-H. (2018). Online multi-object tracking with dual
matching attention networks. In Proceedings of the
European Conference on Computer Vision (ECCV),
pages 366–382.
Zhu, X., Wang, Y., Dai, J., Yuan, L., and Wei, Y. (2017).
Flow-guided feature aggregation for video object de-
tection. In Proceedings of the IEEE International
Conference on Computer Vision, pages 408–417.
VISAPP 2022 - 17th International Conference on Computer Vision Theory and Applications
536