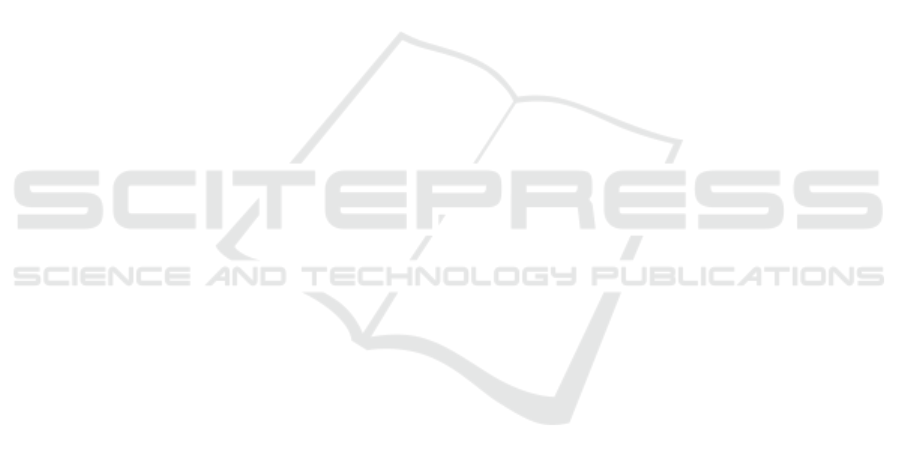
effects of different types of bin pose parametrization
on the network performance.
ACKNOWLEDGEMENTS
The authors gratefully acknowledge the support of
NVIDIA Corporation with the donation of GPUs.
REFERENCES
Besl, P. and McKay, N. D. (1992). A method for registration
of 3-d shapes. IEEE Transactions on Pattern Analysis
and Machine Intelligence, 14(2):239–256.
Bukschat, Y. and Vetter, M. (2020). Efficientpose: An effi-
cient, accurate and scalable end-to-end 6d multi object
pose estimation approach.
Drost, B., Ulrich, M., Navab, N., and Ilic, S. (2010). Model
globally, match locally: Efficient and robust 3d object
recognition. In Proceedings of the IEEE Computer
Society Conference on Computer Vision and Pattern
Recognition, pages 998–1005.
Guo, J., Xing, X., Quan, W., Yan, D.-M., Gu, Q., Liu,
Y., and Zhang, X. (2021). Efficient center voting
for object detection and 6d pose estimation in 3d
point cloud. IEEE Transactions on Image Processing,
30:5072–5084.
Guo, N., Zhang, B., Zhou, J., Zhan, K., and Lai, S.
(2020). Pose estimation and adaptable grasp configu-
ration with point cloud registration and geometry un-
derstanding for fruit grasp planning. Computers and
Electronics in Agriculture, 179:105818.
He, K., Zhang, X., Ren, S., and Sun, J. (2016). Deep resid-
ual learning for image recognition. 2016 IEEE Con-
ference on Computer Vision and Pattern Recognition
(CVPR), pages 770–778.
He, Y., Sun, W., Huang, H., Liu, J., Fan, H., and Sun, J.
(2020). Pvn3d: A deep point-wise 3d keypoints voting
network for 6dof pose estimation.
Hinterstoißer, S., Lepetit, V., Ilic, S., Holzer, S., Brad-
ski, G. R., Konolige, K., and Navab, N. (2012).
Model based training, detection and pose estimation
of texture-less 3d objects in heavily cluttered scenes.
In ACCV.
Hoda
ˇ
n, T., Sundermeyer, M., Drost, B., Labb
´
e, Y., Brach-
mann, E., Michel, F., Rother, C., and Matas, J. (2020).
BOP challenge 2020 on 6D object localization. Euro-
pean Conference on Computer Vision Workshops (EC-
CVW).
Hoda
ˇ
n, T., Barath, D., and Matas, J. (2020). Epos: Estimat-
ing 6d pose of objects with symmetries. In Proceed-
ings of the IEEE/CVF Conference on Computer Vi-
sion and Pattern Recognition (CVPR), pages 11703–
11712.
Hosseini Jafari, O., Mustikovela, S. K., Pertsch, K., Brach-
mann, E., and Rother, C. (2019). ipose: Instance-
aware 6d pose estimation of partly occluded objects.
In Jawahar, C. V., Li, H., Mori, G., and Schindler, K.,
editors, Computer Vision – ACCV 2018, pages 477–
492, Cham. Springer International Publishing.
Katsoulas, D. (2003a). Localization of piled boxes by
means of the hough transform. In Joint Pattern Recog-
nition Symposium, pages 44–51. Springer.
Katsoulas, D. (2003b). Robust extraction of vertices in
range images by constraining the hough transform. In
IbPRIA, pages 360–369.
Mitash, C., Boularias, A., and Bekris, K. E. (2018). Im-
proving 6d pose estimation of objects in clutter via
physics-aware monte carlo tree search. In 2018 IEEE
International Conference on Robotics and Automation
(ICRA), pages 3331–3338.
Park, K., Patten, T., and Vincze, M. (2019). Pix2pose:
Pixel-wise coordinate regression of objects for 6d
pose estimation. 2019 IEEE/CVF International Con-
ference on Computer Vision (ICCV), pages 7667–
7676.
Pereira, N. and Alexandre, L. A. (2020). Maskedfusion:
Mask-based 6d object pose estimation.
Photoneo (2017). Phoxi 3d scanner. https://www.photoneo.
com/products/phoxi-scan-m/.
Pitteri, G., Ramamonjisoa, M., Ilic, S., and Lepetit, V.
(2019). On object symmetries and 6d pose estima-
tion from images. In 2019 International Conference
on 3D Vision (3DV), pages 614–622. IEEE.
Stein, F. and Medioni, G. (1992). Structural index-
ing: efficient 3-d object recognition. IEEE Transac-
tions on Pattern Analysis and Machine Intelligence,
14(2):125–145.
Vidal, J., Lin, C.-Y., Llado, X., and Mart
´
ı, R. (2018). A
method for 6d pose estimation of free-form rigid ob-
jects using point pair features on range data. Sensors,
18:2678.
Vock, R., Dieckmann, A., Ochmann, S., and Klein, R.
(2019). Fast template matching and pose estimation
in 3d point clouds. Computers & Graphics, 79:36–45.
Wang, C., Xu, D., Zhu, Y., Mart
´
ın-Mart
´
ın, R., Lu, C., Fei-
Fei, L., and Savarese, S. (2020). Densefusion: 6d ob-
ject pose estimation by iterative dense fusion.
Xiang, Y., Schmidt, T., Narayanan, V., and Fox, D. (2017).
Posecnn: A convolutional neural network for 6d ob-
ject pose estimation in cluttered scenes. arXiv preprint
arXiv:1711.00199.
Zakharov, S., Shugurov, I., and Ilic, S. (2019). Dpod: 6d
pose object detector and refiner. In International Con-
ference on Computer Vision (ICCV).
Zhou, Y., Barnes, C., Lu, J., Yang, J., and Li, H. (2019). On
the continuity of rotation representations in neural net-
works. In Proceedings of the IEEE/CVF Conference
on Computer Vision and Pattern Recognition, pages
5745–5753.
VISAPP 2022 - 17th International Conference on Computer Vision Theory and Applications
552