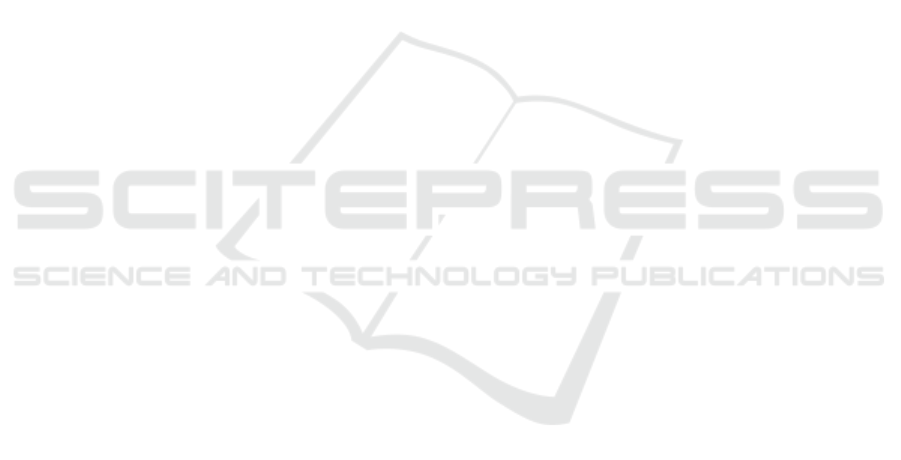
5 CONCLUSION AND FUTURE
WORK
We have demonstrated the feasibility of a novel
single- and multi-camera pose estimation technique
which relies exclusively on the computed 3D head
pose of a human in the scene. A broad range of ex-
periments were carried out on simulated and real im-
ages of vehicle cockpit scenes with varying camera
configurations. Our tests on real multi-camera data
have shown an average translational and rotational er-
ror of about 17 cm and less than 5 degrees, respec-
tively. The proposed method can be applied to use
cases where a certain decrease in accuracy compared
to traditional checkerboard calibration is outweighed
by the natural, easy and flexible handling of the head
pose based calibration. Such use cases include camera
setups within the cockpit of a vehicle, train or plane,
where one or more cameras focus on the occupants,
for example, for the purpose of attention monitoring
or early sensor fusion in a multi-camera environment.
Other potential applications include robot attention
tracking or monitoring costumer interest in automated
stores.
In future work, the 2D facial landmarks employed
in our approach and symmetries typically present in
human faces could potentially be used to extend our
approach to estimate the camera intrinsics as well.
This would allow for the extraction of a full camera
calibration from human faces as a calibration object.
Currently, our approach relies on detecting 2D facial
landmarks for head pose calculation. Further research
could try to alleviate the requirements of facial land-
marks detection in order to generalize the head pose
estimation algorithm to viewing conditions where the
human face is not visible to all cameras.
ACKNOWLEDGEMENTS
This work was partly supported by the Synthetic-
Cabin project (no. 884336), which is funded through
the Austrian Research Promotion Agency (FFG) on
behalf of the Austrian Ministry of Climate Action
(BMK) via its Mobility of the Future funding pro-
gram.
REFERENCES
Abad, F., Camahort, E., and Viv
´
o, R. (2004). Camera
calibration using two concentric circles. In Proc. of
ICIAR, pages 688–696. 1, 2
Ansar, A. and Daniilidis, K. (2002). Linear pose estimation
from points or lines. In Proc. of ECCV, pages 282–
296. 2
Balasubramanian, V., Nallure, Ye, J., and Panchanathan, S.
(2007). Biased manifold embedding: A framework for
person-independent head pose estimation. In Proc. of
CVPR, pages 1–7. 2
Bleser, G., Wuest, H., and Stricker, D. (2006). Online cam-
era pose estimation in partially known and dynamic
scenes. In Proc. of ISMAR, pages 56–65. 2
Camposeco, F., Cohen, A., Pollefeys, M., and Sattler, T.
(2018). Hybrid camera pose estimation. In Proc. of
CVPR, pages 136–144. 2
Chen, L., Zhang, L., Hu, Y., Li, M., and Zhang, H. (2003).
Head pose estimation using fisher manifold learning.
In Proc. of AMFG, pages 203–207. 2
Fanelli, G., Dantone, M., Gall, J., Fossati, A., and v. Gool,
L. (2013). Random forests for real time 3d face anal-
ysis. IJCV, 101(3):437–458. 2
Fanelli, G., Gall, J., and v. Gool, L. (2011). Real time head
pose estimation with random regression forests. In
Proc. of CVPR, pages 617–624. 2
Gross, R. (2021). How the Amazon Go Store’s AI Works.
Towards Data Science (https://bit.ly/3tVHXi2). 1
Gua, J., Deboeverie, F., Slembrouck, M., v. Haerenborgh,
D., v. Cauwelaert, D., Veelaert, P., and Philips, W.
(2015). Extrinsic calibration of camera networks us-
ing a sphere. Sensors, 15(8):18985–19005. 1
H
¨
odlmoser, M., Micusik, B., and Kampel, M. (2011).
Camera auto-calibration using pedestrians and zebra-
crossings. In Proc. of ICCVW, pages 1697–1704. 2
Huang, C., Ding, X., and Fang, C. (2010). Head pose esti-
mation based on random forests for multiclass classi-
fication. In Proc. of ICPR, pages 934–937. 2
Kosuke, T., Dan, M., Mariko, I., and Hideaki, K. (2018).
Human pose as calibration pattern: 3d human pose
estimation with multiple unsynchronized and uncal-
ibrated cameras. In Proc. of CVPRW, pages 1856–
18567. 2
Lamia, A. and Moshiul, H. M. (2019). Vision-based driver’s
attention monitoring system for smart vehicles. In In-
telligent Computing & Optimization, pages 196–209.
1
Li, Y., Wang, S., and Ding, X. (2010). Person-independent
head pose estimation based on random forest regres-
sion. In Proc. of ICIP, pages 1521–1524. 2
Liu, X., Liang, W., Wang, Y., Li, S., and Pei, M. (2016). 3d
head pose estimation with convolutional neural net-
work trained on synthetic images. In Proc. of ICIP,
pages 1289–1293. 2
Lu, C.-P., Hager, G., and Mjolsness, E. (2000). Fast and
globally convergent pose estimation from video im-
ages. TPAMI, 22(6):610–622. 3
Manolis, L. and Xenophon, Z. (2013). Model-based pose
estimation for rigid objects. In Computer Vision Sys-
tems, pages 83–92. 2
Moliner, O., Huang, S., and
˚
Astr
¨
om, K. (2020). Better
prior knowledge improves human-pose-based extrin-
sic camera calibration. In Proc. of ICPR, pages 4758–
4765. 2
Camera Pose Estimation using Human Head Pose Estimation
885