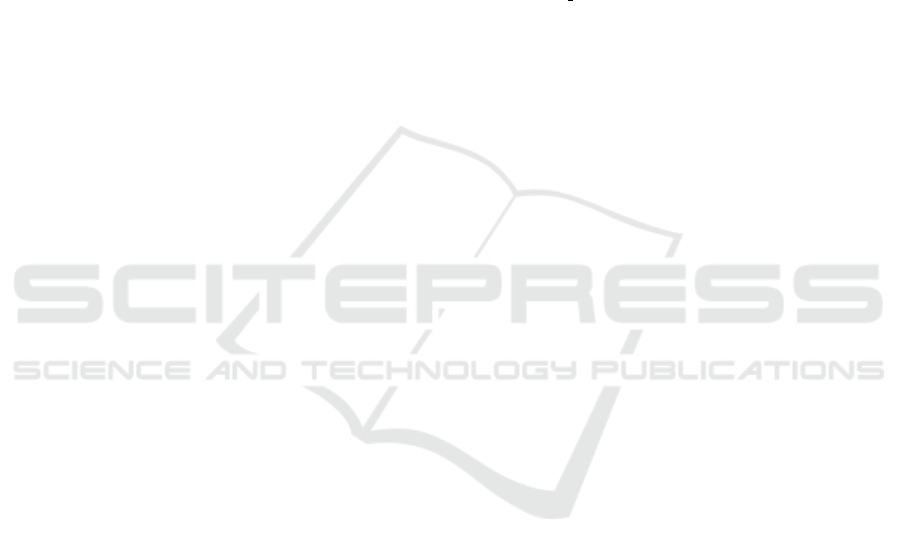
Lepetit, V., Moreno-Noguer, F., and Fua, P. (2009). Epnp:
An accurate o (n) solution to the pnp problem. Interna-
tional Journal of Computer Vision, 81(2):155–166.
Li, Z., Wang, G., and Ji, X. (2019). Cdpn: Coordinates-based
disentangled pose network for real-time rgb-based 6-
dof object pose estimation. In Proc. ICCV.
Liu, H., Su, Y., Rambach, J., Pagani, A., and Stricker, D.
(2020). Tga: Two-level group attention for assembly
state detection. In Proc. ISMAR.
Masood, T. and Egger, J. (2020). Adopting augmented reality
in the age of industrial digitalisation. Computers in
Industry, 115:103112.
Park, K., Patten, T., and Vincze, M. (2019). Pix2pose: Pixel-
wise coordinate regression of objects for 6d pose esti-
mation. In Proc. ICCV.
Peng, S., Liu, Y., Huang, Q., Zhou, X., and Bao, H. (2019).
Pvnet: Pixel-wise voting network for 6dof pose estima-
tion. In Proc. CVPR.
Prisacariu, V. A. and Reid, I. D. (2012). Pwp3d: Real-time
segmentation and tracking of 3d objects. International
Journal of Computer Vision, 98(3):335–354.
Rad, M. and Lepetit, V. (2017). Bb8: A scalable, accurate,
robust to partial occlusion method for predicting the
3d poses of challenging objects without using depth.
In Proc. ICCV.
Seibold, C., Hilsmann, A., and Eisert, P. (2017). Model-
based motion blur estimation for the improvement of
motion tracking. Computer Vision and Image Under-
standing, 160:45–56.
Seo, B.-K., Park, H., Park, J.-I., Hinterstoisser, S., and Ilic,
S. (2013). Optimal local searching for fast and ro-
bust textureless 3d object tracking in highly cluttered
backgrounds. IEEE Transactions on Visualization and
Computer Galraphics, 20(1):99–110.
Sock, J., Castro, P., Armagan, A., Garcia-Hernando, G.,
and Kim, T.-K. (2020). Tackling two challenges of
6d object pose estimation: Lack of real annotated rgb
images and scalability to number of objects. arXiv
preprint arXiv:2003.12344v1.
Song, C., Song, J., and Huang, Q. (2020). Hybridpose: 6d
object pose estimation under hybrid representations. In
Proc. CVPR.
Steinbach, E., Eisert, P., and Girod, B. (2001). Model-based
3-d shape and motion estimation using sliding textures.
In Proc. VMV.
Su, Y., Rambach, J., Minaskan, N., Lesur, P., Pagani, A.,
and Stricker, D. (2019). Deep multi-state object pose
estimation for augmented reality assembly. In Proc.
ISMAR.
Sun, D., Roth, S., and Black, M. J. (2010). Secrets of optical
flow estimation and their principles. In Proc. CVPR.
Sun, X., Zhou, J., Zhang, W., Wang, Z., and Yu, Q. (2021).
Robust monocular pose tracking of less-distinct ob-
jects based on contour-part model. IEEE Transac-
tions on Circuits and Systems for Video Technology,
31(11):4409–4421.
Tan, Z., Chen, D., Chu, Q., Chai, M., Liao, J., He, M.,
Yuan, L., Hua, G., and Yu, N. (2021). Efficient seman-
tic image synthesis via class-adaptive normalization.
IEEE Transactions on Pattern Analysis and Machine
Intelligence.
Thalhammer, S., Leitner, M., Patten, T., and Vincze, M.
(2021). Pyrapose: Feature pyramids for fast and ac-
curate object pose estimation under domain shift. In
Proc. ICRA.
Tjaden, H., Schwanecke, U., Sch
¨
omer, E., and Cremers,
D. (2018). A region-based gauss-newton approach to
real-time monocular multiple object tracking. IEEE
Transactions on Pattern Analysis and Machine Intelli-
gence, 41(8):1797–1812.
To, T., Tremblay, J., McKay, D., Yamaguchi, Y., Le-
ung, K., Balanon, A., Cheng, J., Hodge, W., and
Birchfield, S. (2018). NDDS: NVIDIA deep learn-
ing dataset synthesizer. https://github.com/NVIDIA/
Dataset Synthesizer.
Tobin, J., Fong, R., Ray, A., Schneider, J., Zaremba, W.,
and Abbeel, P. (2017). Domain randomization for
transferring deep neural networks from simulation to
the real world. In Proc. IROS.
Tremblay, J., To, T., Sundaralingam, B., Xiang, Y., Fox, D.,
and Birchfield, S. (2018). Deep object pose estimation
for semantic robotic grasping of household objects. In
Proc. CoRL.
Vassallo, R., Rankin, A., Chen, E. C., and Peters, T. M.
(2017). Hologram stability evaluation for microsoft
hololens. In Proc. SPIE Volume 10136.
Wang, B., Zhong, F., and Qin, X. (2019). Robust edge-based
3d object tracking with direction-based pose valida-
tion. Multimedia Tools and Applications, 78(9):12307–
12331.
Wang, G., Manhardt, F., Shao, J., Ji, X., Navab, N., and
Tombari, F. (2020). Self6d: Self-supervised monocular
6d object pose estimation. In Proc. ECCV.
Wang, G., Wang, B., Zhong, F., Qin, X., and Chen, B.
(2015). Global optimal searching for textureless 3d
object tracking. The Visual Computer, 31(6):979–988.
Xiang, Y., Schmidt, T., Narayanan, V., and Fox, D. (2018).
Posecnn: A convolutional neural network for 6d object
pose estimation in cluttered scenes. In Proc. RSS.
Yu, X., Zhuang, Z., Koniusz, P., and Li, H. (2020). 6dof
object pose estimation via differentiable proxy voting
regularizer. In Proc. BMVC.
Zakharov, S., Shugurov, I., and Ilic, S. (2019). DPOD: 6d
pose object detector and refiner. In Proc. ICCV.
Zhang, Z. (1997). Parameter estimation techniques: A tuto-
rial with application to conic fitting. Image and Vision
Computing, 15(1):59–76.
Zhong, L. and Zhang, L. (2019). A robust monocular 3d
object tracking method combining statistical and photo-
metric constraints. International Journal of Computer
Vision, 127(8):973–992.
Zubizarreta, J., Aguinaga, I., and Amundarain, A. (2019). A
framework for augmented reality guidance in industry.
The International Journal of Advanced Manufacturing
Technology, 102(9):4095–4108.
VISAPP 2022 - 17th International Conference on Computer Vision Theory and Applications
756