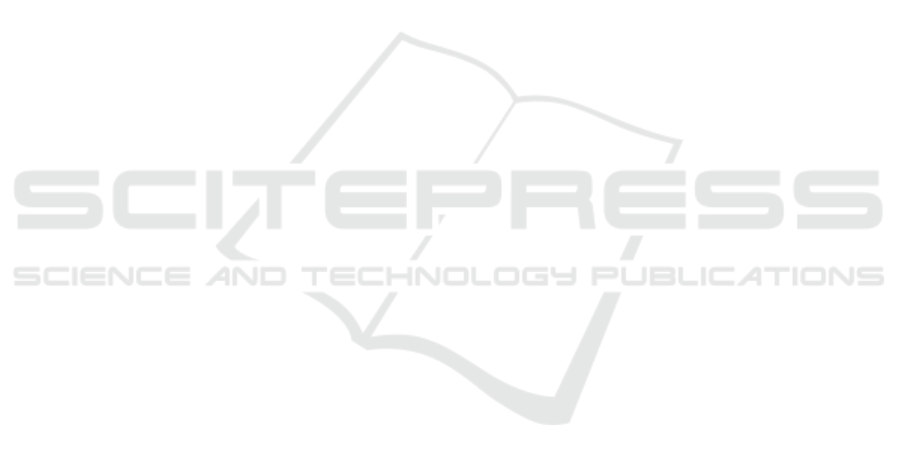
Geirhos, R., Jacobsen, J.-H., Michaelis, C., Zemel, R.,
Brendel, W., Bethge, M., and Wichmann, F. A. (2020).
Shortcut learning in deep neural networks. Nature
Machine Intelligence, 2(11):665–673.
Godard, C., Mac Aodha, O., and Brostow, G. J. (2017). Un-
supervised monocular depth estimation with left-right
consistency. In Proceedings of the IEEE Conference
on Computer Vision and Pattern Recognition, pages
270–279.
Godard, C., Mac Aodha, O., Firman, M., and Brostow, G. J.
(2019). Digging into self-supervised monocular depth
estimation. In Proceedings of the IEEE/CVF Interna-
tional Conference on Computer Vision, pages 3828–
3838.
Goldman, M., Hassner, T., and Avidan, S. (2019). Learn
stereo, infer mono: Siamese networks for self-
supervised, monocular, depth estimation. In Proceed-
ings of the IEEE Conference on Computer Vision and
Pattern Recognition Workshops, pages 0–0.
Gordon, A., Li, H., Jonschkowski, R., and Angelova, A.
(2019). Depth from videos in the wild: Unsupervised
monocular depth learning from unknown cameras.
Guizilini, V., Ambrus, R., Pillai, S., Raventos, A., and
Gaidon, A. (2020). 3d packing for self-supervised
monocular depth estimation. In Proceedings of the
IEEE/CVF Conference on Computer Vision and Pat-
tern Recognition, pages 2485–2494.
Guo, X., Li, H., Yi, S., Ren, J., and Wang, X. (2018). Learn-
ing monocular depth by distilling cross-domain stereo
networks. In Proceedings of the European Conference
on Computer Vision (ECCV), pages 484–500.
He, K., Zhang, X., Ren, S., and Sun, J. (2016). Deep resid-
ual learning for image recognition. In Proceedings of
the IEEE conference on computer vision and pattern
recognition, pages 770–778.
Hendrycks, D. and Dietterich, T. (2019). Benchmarking
neural network robustness to common corruptions and
perturbations. Proceedings of the International Con-
ference on Learning Representations.
Jiang, H. and Huang, R. (2019). Hierarchical binary classi-
fication for monocular depth estimation. In 2019 IEEE
International Conference on Robotics and Biomimet-
ics (ROBIO), pages 1975–1980. IEEE.
Johnston, A. and Carneiro, G. (2020). Self-supervised
monocular trained depth estimation using self-
attention and discrete disparity volume. In Proceed-
ings of the ieee/cvf conference on computer vision and
pattern recognition, pages 4756–4765.
Kingma, D. P. and Ba, J. (2014). Adam: A
method for stochastic optimization. arXiv preprint
arXiv:1412.6980.
Klingner, M., Term
¨
ohlen, J.-A., Mikolajczyk, J., and
Fingscheidt, T. (2020). Self-Supervised Monocular
Depth Estimation: Solving the Dynamic Object Prob-
lem by Semantic Guidance. In ECCV.
Kong, S. and Fowlkes, C. (2019). Pixel-wise attentional
gating for scene parsing. In 2019 IEEE Winter Con-
ference on Applications of Computer Vision (WACV),
pages 1024–1033. IEEE.
Kurakin, A., Goodfellow, I., Bengio, S., et al. (2016). Ad-
versarial examples in the physical world.
Lee, J. H., Han, M.-K., Ko, D. W., and Suh, I. H. (2019).
From big to small: Multi-scale local planar guid-
ance for monocular depth estimation. arXiv preprint
arXiv:1907.10326.
Li, B., Dai, Y., and He, M. (2018a). Monocular depth es-
timation with hierarchical fusion of dilated cnns and
soft-weighted-sum inference. Pattern Recognition,
83:328–339.
Li, R., Xian, K., Shen, C., Cao, Z., Lu, H., and Hang, L.
(2018b). Deep attention-based classification network
for robust depth prediction. In Asian Conference on
Computer Vision (ACCV).
Li, Z., Liu, X., Drenkow, N., Ding, A., Creighton,
F. X., Taylor, R. H., and Unberath, M. (2020). Re-
visiting stereo depth estimation from a sequence-
to-sequence perspective with transformers. arXiv
preprint arXiv:2011.02910.
Liebel, L. and K
¨
orner, M. (2019). Multidepth: Single-
image depth estimation via multi-task regression and
classification. In 2019 IEEE Intelligent Transporta-
tion Systems Conference (ITSC), pages 1440–1447.
IEEE.
Lin, G., Milan, A., Shen, C., and Reid, I. (2017). Refinenet:
Multi-path refinement networks for high-resolution
semantic segmentation. In Proceedings of the IEEE
conference on computer vision and pattern recogni-
tion, pages 1925–1934.
Liu, Z., Lin, Y., Cao, Y., Hu, H., Wei, Y., Zhang, Z., Lin,
S., and Guo, B. (2021). Swin transformer: Hierarchi-
cal vision transformer using shifted windows. arXiv
preprint arXiv:2103.14030.
Lopez, M., Mari, R., Gargallo, P., Kuang, Y., Gonzalez-
Jimenez, J., and Haro, G. (2019). Deep single image
camera calibration with radial distortion. In Proceed-
ings of the IEEE/CVF Conference on Computer Vision
and Pattern Recognition, pages 11817–11825.
Loshchilov, I. and Hutter, F. (2017). Decoupled weight de-
cay regularization. arXiv preprint arXiv:1711.05101.
Lyu, X., Liu, L., Wang, M., Kong, X., Liu, L., Liu, Y., Chen,
X., and Yuan, Y. (2020). Hr-depth: high resolution
self-supervised monocular depth estimation. CoRR
abs/2012.07356.
Madry, A., Makelov, A., Schmidt, L., Tsipras, D., and
Vladu, A. (2018). Towards deep learning models
resistant to adversarial attacks. In 6th International
Conference on Learning Representations, ICLR 2018,
Vancouver, BC, Canada, April 30 - May 3, 2018, Con-
ference Track Proceedings. OpenReview.net.
Mahjourian, R., Wicke, M., and Angelova, A. (2018). Un-
supervised learning of depth and ego-motion from
monocular video using 3d geometric constraints. In
Proceedings of the IEEE Conference on Computer Vi-
sion and Pattern Recognition, pages 5667–5675.
Michaelis, C., Mitzkus, B., Geirhos, R., Rusak, E., Bring-
mann, O., Ecker, A. S., Bethge, M., and Brendel, W.
(2019). Benchmarking robustness in object detection:
Autonomous driving when winter is coming. arXiv
preprint arXiv:1907.07484.
VISAPP 2022 - 17th International Conference on Computer Vision Theory and Applications
768