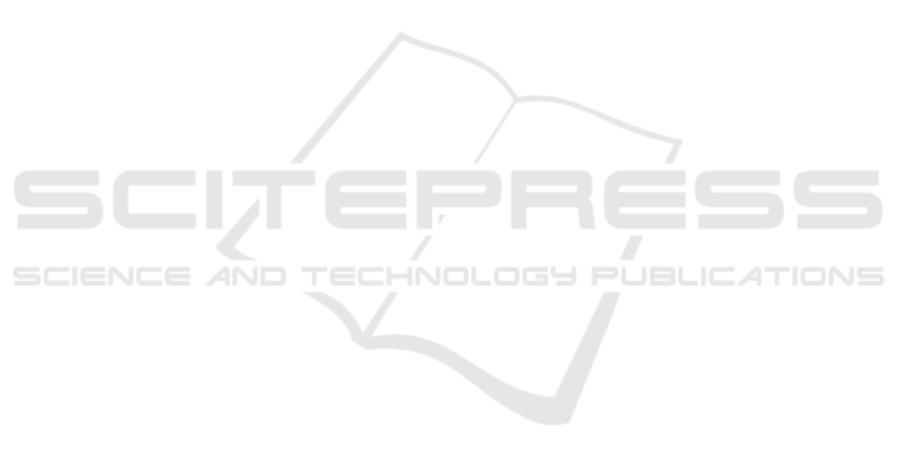
taking into account the rendering capabilities of Jet-
son AGX Xavier.
ACKNOWLEDGEMENTS
This work was supported by Polish National
Science Center (NCN) under a research grant
2017/27/B/ST6/01743.
REFERENCES
Brachmann, E., Michel, F., Krull, A., Yang, M., Gumhold,
S., and Rother, C. (2016). Uncertainty-driven 6D pose
estimation of objects and scenes from a single RGB
image. In CVPR, pages 3364–3372.
Brachmann, E. and Rother, C. (2019). Neural-guided
RANSAC: Learning where to sample model hypothe-
ses. In IEEE/CVF Int. Conf. on Computer Vision
(ICCV), pages 4321–4330.
Chen, W., Jia, X., Chang, H. J., Duan, J., Shen, L., and
Leonardis, A. (2021). FS-Net: Fast shape-based net-
work for category-level 6D object pose estimation
with decoupled rotation mechanism. In IEEE Conf. on
Comp. Vision and Pattern Rec., CVPR, pages 1581–
1590. Comp. Vision Foundation / IEEE.
Egger, B., Sch
¨
onborn, S., Schneider, A., Kortylewski, A.,
Morel-Forster, A., Blumer, C., and Vetter, T. (2018).
Occlusion-aware 3D morphable models and an illumi-
nation prior for face image analysis. Int. J. Comput.
Vision, 126(12):1269–1287.
Fan, Z., Zhu, Y., He, Y., Sun, Q., Liu, H., and He, J.
(2021). Deep learning on monocular object pose
detection and tracking: A comprehensive overview.
arXiv 2105.14291.
Hinterstoisser, S., Lepetit, V., Ilic, S., Holzer, S., Bradski,
G., Konolige, K., and Navab, N. (2013). Model based
training, detection and pose estimation of texture-less
3D objects in heavily cluttered scenes. In Computer
Vision – ACCV 2012, pages 548–562. Springer.
Hu, Y., Hugonot, J., Fua, P., and Salzmann, M. (2019).
Segmentation-driven 6D object pose estimation. In
IEEE Conf. on Computer Vision and Pattern Rec.,
CVPR, pages 3385–3394.
Kaskman, R., Zakharov, S., Shugurov, I., and Ilic, S.
(2019). HomebrewedDB: RGB-D dataset for 6D pose
estimation of 3D objects. In IEEE Int. Conf. on Com-
puter Vision Workshop (ICCVW), pages 2767–2776.
Kehl, W., Manhardt, F., Tombari, F., Ilic, S., and Navab, N.
(2017). SSD-6D: Making RGB-Based 3D Detection
and 6D Pose Estimation Great Again. In IEEE Int.
Conf. on Computer Vision, pages 1530–1538.
Kutschireiter, A., Surace, C., Sprekeler, H., and Pfister, J.-P.
(2017). Nonlinear Bayesian filtering and learning: A
neuronal dynamics for perception. Scientific Reports,
7(1).
Majcher, M. and Kwolek, B. (2021). Deep quaternion pose
proposals for 6D object pose tracking. In Proceed-
ings of the IEEE/CVF Int. Conf. on Computer Vision
(ICCV) Workshops, pages 243–251.
Manhardt, F., Wang, G., Busam, B., Nickel, M., Meier, S.,
Minciullo, L., Ji, X., and Navab, N. (2020). CPS++:
Improving class-level 6D pose and shape estimation
from monocular images with self-supervised learning.
arXiv 2003.05848.
Newell, A., Yang, K., and Deng, J. (2016). Stacked hour-
glass networks for human pose estimation. In ECCV,
pages 483–499. Springer.
Pavlakos, G., Zhou, X., Chan, A., Derpanis, K. G., and
Daniilidis, K. (2017). 6-DoF object pose from seman-
tic keypoints. In IEEE Int. Conf. on Robotics and Au-
tomation (ICRA), pages 2011–2018.
Peng, S., Liu, Y., Huang, Q., Zhou, X., and Bao, H. (2019).
PVNet: Pixel-Wise Voting Network for 6DoF Pose
Estimation. In IEEE Conf. on Comp. Vision and Patt.
Rec., pages 4556–4565.
Prisacariu, V. A. and Reid, I. D. (2012). PWP3D: Real-
Time Segmentation and Tracking of 3D Objects. Int.
J. Comput. Vision, 98(3):335–354.
Rad, M. and Lepetit, V. (2017). BB8: A scalable, accurate,
robust to partial occlusion method for predicting the
3D poses of challenging objects without using depth.
In IEEE Int. Conf. on Comp. Vision, pages 3848–3856.
Tekin, B., Sinha, S. N., and Fua, P. (2018). Real-time
seamless single shot 6D object pose prediction. In
IEEE/CVF Conf. on Comp. Vision and Pattern Rec.
(CVPR), pages 292–301. IEEE Comp. Society.
Tjaden, H., Schwanecke, U., Sch
¨
omer, E., and Cremers, D.
(2019). A region-based Gauss-Newton approach to
real-time monocular multiple object tracking. IEEE
Trans. on PAMI, 41(8):1797–1812.
Tremblay, J., To, T., Sundaralingam, B., Xiang, Y., Fox, D.,
and Birchfield, S. (2018). Deep object pose estimation
for semantic robotic grasping of household objects. In
Proc. 2nd Conf. on Robot Learn., volume 87 of Proc.
of Machine Learning Research, pages 306–316.
Wang, Z., Li, W., Kao, Y., Zou, D., Wang, Q., Ahn, M., and
Hong, S. (2018). HCR-Net: A hybrid of classification
and regression network for object pose estimation. IJ-
CAI’18, pages 1014–1020. AAAI Press.
Whelan, T., Salas-Moreno, R. F., Glocker, B., Davison,
A. J., and Leutenegger, S. (2016). ElasticFusion. Int.
J. Rob. Res., 35(14):1697–1716.
Wu, P., Lee, Y., Tseng, H., Ho, H., Yang, M., and Chien,
S. (2017). A benchmark dataset for 6DoF object pose
tracking. In IEEE Int. Symp. on Mixed and Aug. Real-
ity, pages 186–191.
Xiang, Y., Schmidt, T., Narayanan, V., and Fox, D. (2018).
PoseCNN: A Convolutional Neural Network for 6D
Object Pose Estimation in Cluttered Scenes. In
IEEE/RSJ Int. Conf. on Intel. Robots and Systems.
Zakharov, S., Shugurov, I., and Ilic, S. (2019). DPOD: 6D
pose object detector and refiner. In IEEE/CVF Int.
Conf. on Computer Vision (ICCV), pages 1941–1950.
IEEE Computer Society.
Pose Guided Feature Learning for 3D Object Tracking on RGB Videos
581