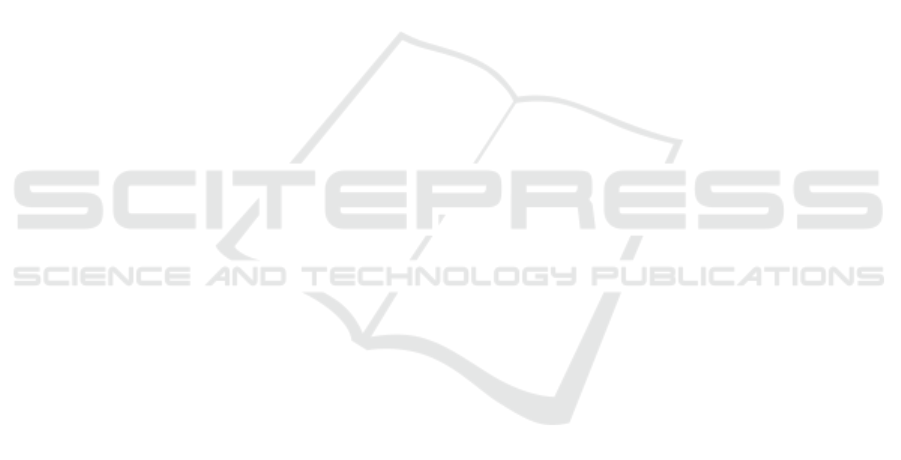
Berrar, D. (2019). Cross-validation. In Ranganathan, S.,
Gribskov, M., Nakai, K., and Sch
¨
onbach, C., editors,
Encyclopedia of Bioinformatics and Computational
Biology - Volume 1, pages 542–545. Elsevier.
Chen, E., Lerman, K., and Ferrara, E. (2020). Tracking
social media discourse about the covid-19 pandemic:
Development of a public coronavirus twitter data set.
JMIR Public Health and Surveillance, 6(2):e19273.
Decoupes, R., Kafando, R., Roche, M., and Teisseire, M.
(2021). H-tfidf: What makes areas specific over time
in the massive flow of tweets related to the covid pan-
demic? AGILE: GIScience Series, 2:1–8.
Devlin, J., Chang, M.-W., Lee, K., and Toutanova, K.
(2018). Bert: Pre-training of deep bidirectional trans-
formers for language understanding. arXiv preprint
arXiv:1810.04805.
Dubey, A. D. (2020). Twitter sentiment analysis during
covid-19 outbreak. Available at SSRN 3572023.
Fernandes, B., Biswas, U. N., Mansukhani, R. T., Casar
´
ın,
A. V., and Essau, C. A. (2020). The impact of covid-
19 lockdown on internet use and escapism in adoles-
cents. Revista de psicolog
´
ıa cl
´
ınica con ni
˜
nos y ado-
lescentes, 7(3):59–65.
Ferrara, E. (2020). # covid-19 on twitter: Bots, conspira-
cies, and social media activism. arXiv preprint arXiv:
2004.09531.
Gulati, K. (2021). Comparative analysis of machine
learning-based classification models using sentiment
classification of tweets related to covid-19 pandemic.
Materials Today: Proceedings.
Ho, S. Y. and Tan (2021). What can venn diagrams teach us
about doing data science better? International Journal
of Data Science and Analytics, 11(1):1–10.
Hoang, M., Bihorac, O. A., and Rouces, J. (2019). Aspect-
based sentiment analysis using BERT. In Proceedings
of the 22nd Nordic Conference on Computational Lin-
guistics, pages 187–196, Turku, Finland. Link
¨
oping
University Electronic Press.
KazAnova (2016). Sentiment140 dataset.
https://www.kaggle.com/kazanova/sentiment140.
Kou, G., Yang, P., Peng, Y., Xiao, F., Chen, Y., and Alsaadi,
F. E. (2020). Evaluation of feature selection methods
for text classification with small datasets using mul-
tiple criteria decision-making methods. Applied Soft
Computing, 86:105836.
Kumar, S. V. K. R. (2014). Analysis of feature selection
algorithms on classification: a survey.
Madasu, A. and Elango, S. (2020). Efficient feature se-
lection techniques for sentiment analysis. Multimedia
Tools and Applications, 79(9):6313–6335.
Mansoor, M., Gurumurthy, K., Prasad, V., et al. (2020).
Global sentiment analysis of covid-19 tweets over
time. arXiv preprint arXiv:2010.14234.
Organization, W. H. et al. (2020). Aparttogether survey:
preliminary overview of refugees and migrants self-
reported impact of covid-19.
Prusa, J. D., Khoshgoftaar, T. M., and Dittman, D. J. (2015).
Impact of feature selection techniques for tweet sen-
timent classification. In The Twenty-eighth interna-
tional flairs conference.
Qaiser, S. and Ali, R. (2018). Text mining: use of
tf-idf to examine the relevance of words to docu-
ments. International Journal of Computer Applica-
tions, 181(1):25–29.
Raschka, S. (2018). Model evaluation, model selection,
and algorithm selection in machine learning. arXiv
preprint arXiv:1811.12808.
Rustam, F., Khalid, M., Aslam, W., Rupapara, V.,
Mehmood, A., and Choi, G. S. (2021). A performance
comparison of supervised machine learning models
for covid-19 tweets sentiment analysis. Plos one,
16(2):e0245909.
Schouten, K., Van Der Weijde, O., Frasincar, F., and
Dekker, R. (2017). Supervised and unsupervised as-
pect category detection for sentiment analysis with co-
occurrence data. IEEE transactions on cybernetics,
48(4):1263–1275.
Sharma, A. and Ghose, U. (2021). Lexicon a linguistic
approach for sentiment classification. In 2021 11th
International Conference on Cloud Computing, Data
Science & Engineering (Confluence), pages 887–893.
IEEE.
Shen, C.-w., Chen, M., and Wang, C.-c. (2019). Analyz-
ing the trend of o2o commerce by bilingual text min-
ing on social media. Computers in Human Behavior,
101:474–483.
Wang, Z. and Lin, Z. (2020). Optimal feature selection for
learning-based algorithms for sentiment classification.
Cognitive Computation, 12(1):238–248.
WHO (2020). Who announces covid-19 outbreak a pan-
demic.
Wisesty, U. N., Rismala, R., Munggana, W., and Purwari-
anti, A. (2021). Comparative study of covid-19 tweets
sentiment classification methods. In 2021 9th Inter-
national Conference on Information and Communica-
tion Technology (ICoICT), pages 588–593. IEEE.
Yahav, I., Shehory, O., and Schwartz, D. (2018). Comments
mining with tf-idf: the inherent bias and its removal.
IEEE Transactions on Knowledge and Data Engineer-
ing, 31(3):437–450.
¨
Ozcan, S. (2016). tweet-preprocessor: Elegant tweet pre-
processing. https://github.com/s/preprocessor.
HEALTHINF 2022 - 15th International Conference on Health Informatics
656