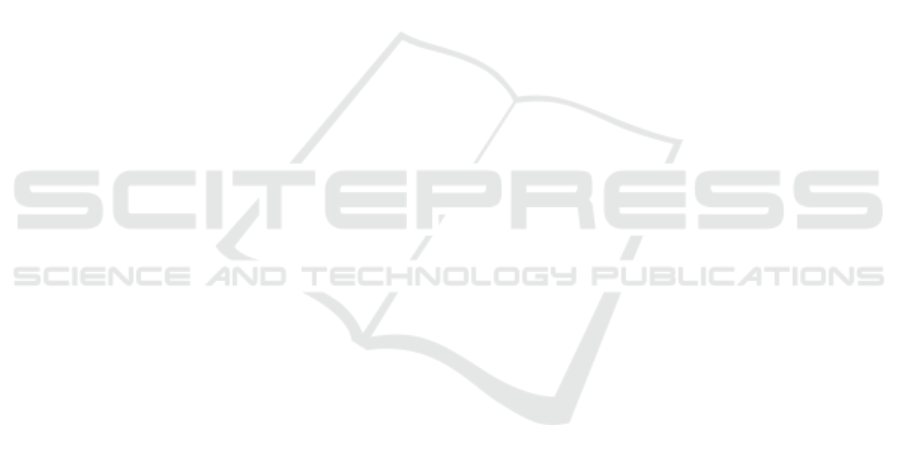
constructs. International Journal of Industrial Er-
gonomics, 24(6):631–645.
He, K., Gkioxari, G., Doll
´
ar, P., and Girshick, R. (2017).
Mask r-cnn. In 2017 IEEE International Conference
on Computer Vision (ICCV), pages 2980–2988.
Holmqvist, K., Nystr
¨
om, M., Andersson, R., Dewhurst, R.,
Jarodzka, H., and van de Weijer, J. (2015). Eye track-
ing: a comprehensive guide to methods and measures.
Oxford University Press.
Hunter, J. D. (2007). Matplotlib: A 2d graphics environ-
ment. Computing in Science & Engineering, 9(3):90–
95.
Irimia, A., Chambers, M. C., Torgerson, C. M., and Van
Horn, J. D. (2012). Circular representation of hu-
man cortical networks for subject and population-level
connectomic visualization. NeuroImage, 60(2):1340–
1351.
Judd, T., Ehinger, K., Durand, F., and Torralba, A. (2009).
Learning to predict where humans look. In 2009 IEEE
12th International Conference on Computer Vision,
pages 2106–2113.
Kim, D., Woo, S., Lee, J.-Y., and Kweon, I. S. (2020).
Video panoptic segmentation. In 2020 IEEE/CVF
Conference on Computer Vision and Pattern Recog-
nition (CVPR), pages 9856–9865.
Kirillov, A., Girshick, R., He, K., and Doll
´
ar, P.
(2019a). Panoptic feature pyramid networks. In 2019
IEEE/CVF Conference on Computer Vision and Pat-
tern Recognition (CVPR), pages 6392–6401.
Kirillov, A., He, K., Girshick, R., Rother, C., and Doll
´
ar, P.
(2019b). Panoptic segmentation. In 2019 IEEE/CVF
Conference on Computer Vision and Pattern Recogni-
tion (CVPR), pages 9396–9405.
Kurzhals, K., Bopp, C. F., B
¨
assler, J., Ebinger, F., and
Weiskopf, D. (2014). Benchmark data for evaluating
visualization and analysis techniques for eye tracking
for video stimuli. In Proceedings of the Fifth Work-
shop on Beyond Time and Errors: Novel Evaluation
Methods for Visualization, BELIV ’14, page 54–60,
New York, NY, USA. Association for Computing Ma-
chinery.
Kurzhals, K. and Weiskopf, D. (2013). Space-time vi-
sual analytics of eye-tracking data for dynamic stim-
uli. IEEE Transactions on Visualization and Com-
puter Graphics, 19(12):2129–2138.
Kurzhals, K. and Weiskopf, D. (2015). Aoi transition trees.
In Proceedings of the 41st Graphics Interface Confer-
ence, GI ’15, page 41–48, CAN. Canadian Informa-
tion Processing Society.
Lin, T.-Y., Maire, M., Belongie, S., Hays, J., Perona, P., Ra-
manan, D., Doll
´
ar, P., and Zitnick, C. L. (2014). Mi-
crosoft coco: Common objects in context. In Fleet,
D., Pajdla, T., Schiele, B., and Tuytelaars, T., edi-
tors, Computer Vision – ECCV 2014, pages 740–755,
Cham. Springer International Publishing.
Panetta, K., Wan, Q., Rajeev, S., Kaszowska, A., Gardony,
A. L., Naranjo, K., Taylor, H. A., and Agaian, S.
(2020). Iseecolor: Method for advanced visual ana-
lytics of eye tracking data. IEEE Access, 8:52278–
52287.
Paszke, A., Gross, S., Massa, F., Lerer, A., Bradbury, J.,
Chanan, G., Killeen, T., Lin, Z., Gimelshein, N.,
Antiga, L., Desmaison, A., Kopf, A., , E., DeVito,
Z., Raison, M., Tejani, A., Chilamkurthy, S., Steiner,
B., Fang, L., Bai, J., and Chintala, S. (2019). Pytorch:
An imperative style, high-performance deep learning
library. In Advances in Neural Information Process-
ing Systems 32, pages 8024–8035. Curran Associates,
Inc.
Privitera, C. and Stark, L. (2000). Algorithms for defining
visual regions-of-interest: comparison with eye fixa-
tions. IEEE Transactions on Pattern Analysis and Ma-
chine Intelligence, 22(9):970–982.
Raschke, M., Chen, X., and Ertl, T. (2012). Parallel scan-
path visualization. In Proceedings of the Symposium
on Eye Tracking Research and Applications, ETRA
’12, page 165–168, New York, NY, USA. Association
for Computing Machinery.
Richardson, D. C. and Dale, R. (2005). Looking to under-
stand: The coupling between speakers’ and listeners’
eye movements and its relationship to discourse com-
prehension. Cognitive Science, 29(6):1045–1060.
Santella, A. and DeCarlo, D. (2004). Robust clustering of
eye movement recordings for quantification of visual
interest. In Proceedings of the 2004 Symposium on
Eye Tracking Research & Applications, ETRA ’04,
page 27–34, New York, NY, USA. Association for
Computing Machinery.
Shelhamer, E., Long, J., and Darrell, T. (2017). Fully con-
volutional networks for semantic segmentation. IEEE
Transactions on Pattern Analysis and Machine Intel-
ligence, 39(4):640–651.
Tighe, J., Niethammer, M., and Lazebnik, S. (2014). Scene
parsing with object instances and occlusion ordering.
In 2014 IEEE Conference on Computer Vision and
Pattern Recognition, pages 3748–3755.
Tu, Z., Chen, X., Yuille, A. L., and Zhu, S.-C. (2005). Im-
age parsing: Unifying segmentation, detection, and
recognition. Int. J. Comput. Vision, 63(2):113–140.
Wolf, J., Hess, S., Bachmann, D., Lohmeyer, Q., and
Meboldt, M. (2018). Automating areas of interest
analysis in mobile eye tracking experiments based
on machine learning. Journal of Eye Movement Re-
search, 11(6).
Wu, Y., Kirillov, A., Massa, F., Lo, W.-Y., and Gir-
shick, R. (2019). Detectron2. https://github.com/
facebookresearch/detectron2.
Zanca, D., Serchi, V., Piu, P., Rosini, F., and Rufa, A.
(2018). Fixatons: A collection of human fixations
datasets and metrics for scanpath similarity.
Zhang, H., Dana, K., Shi, J., Zhang, Z., Wang, X., Tyagi,
A., and Agrawal, A. (2018). Context encoding for se-
mantic segmentation. In 2018 IEEE/CVF Conference
on Computer Vision and Pattern Recognition, pages
7151–7160.
IVAPP 2022 - 13th International Conference on Information Visualization Theory and Applications
178