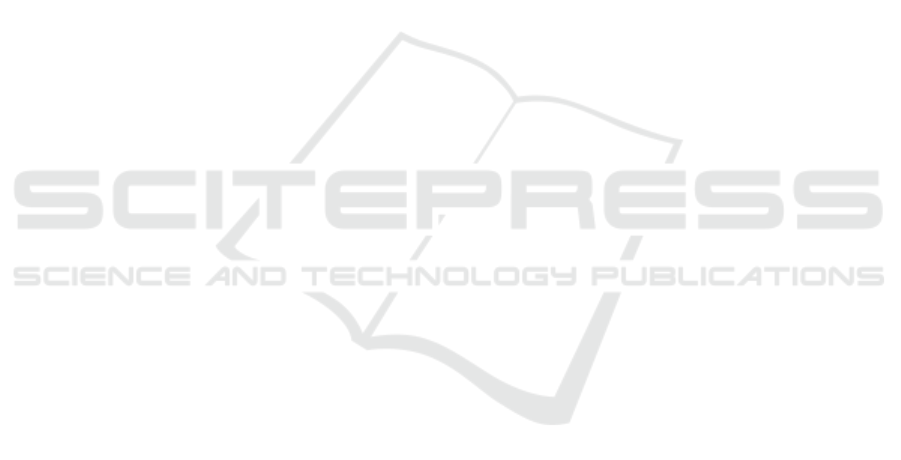
INCA (2021). Types of cancer — national can-
cer institute - jos
´
e alencar gomes da silva -
inca. https://www.inca.gov.br/tipos-de-cancer/cancer-
de-penis. (Accessed on 10/06/2021).
Kiani, A., Uyumazturk, B., Rajpurkar, P., Wang, A., Gao,
R., Jones, E., Yu, Y., Langlotz, C., Ball, R., Mon-
tine, T., Martin, B., Berry, G., Ozawa, M., Hazard, F.,
Brown, R., Chen, S., Wood, M., Allard, L., Ylagan,
L., and Shen, J. (2020). Impact of a deep learning
assistant on the histopathologic classification of liver
cancer. npj Digital Medicine, 3.
Krizhevsky, A., Sutskever, I., and Hinton, G. E. (2012).
Imagenet classification with deep convolutional neu-
ral networks. In Proceedings of the 25th Interna-
tional Conference on Neural Information Processing
Systems - Volume 1, NIPS’12, page 1097–1105, Red
Hook, NY, USA. Curran Associates Inc.
Kumar, A. and Shaik, F. (2015). Image Processing in Dia-
betic Related Causes. SpringerBriefs in Applied Sci-
ences and Technology. Springer Singapore.
Lecun, Y., Bottou, L., Bengio, Y., and Haffner, P. (1998).
Gradient-based learning applied to document recogni-
tion. Proceedings of the IEEE, 86(11):2278–2324.
Linkon, A. H. M., Labib, M. M., Hasan, T., Hossain,
M., and Marium-E-Jannat (2021). Deep learning
in prostate cancer diagnosis and gleason grading in
histopathology images: An extensive study. Informat-
ics in Medicine Unlocked, 24:100582.
Melo, R. C. N., Raas, M. W. D., Palazzi, C., Neves, V. H.,
Malta, K. K., and Silva, T. P. (2020). Whole slide
imaging and its applications to histopathological stud-
ies of liver disorders. Frontiers in Medicine, 6:310.
Morid, M. A., Borjali, A., and Del Fiol, G. (2021). A scop-
ing review of transfer learning research on medical
image analysis using imagenet. Computers in Biology
and Medicine, 128:104115.
Neto, A. D. (2012). Cytopathology technician: reference
book 3: Histopathology techniques — bras
´
ılia;
ministry of health of brazil ; 2012. 93 p.— ms.
https://pesquisa.bvsalud.org/bvsms/resource/pt/mis-
37140. (Accessed on 10/11/2021).
Rakhlin, A., Shvets, A., Iglovikov, V., and Kalinin, A. A.
(2018). Deep convolutional neural networks for breast
cancer histology image analysis. In Campilho, A.,
Karray, F., and ter Haar Romeny, B., editors, Im-
age Analysis and Recognition, pages 737–744, Cham.
Springer International Publishing.
Russakovsky, O., Deng, J., Su, H., Krause, J., Satheesh, S.,
Ma, S., Huang, Z., Karpathy, A., Khosla, A., Bern-
stein, M., et al. (2015). Imagenet large scale visual
recognition challenge. International journal of com-
puter vision, 115(3):211–252.
Sarwinda, D., Paradisa, R. H., Bustamam, A., and Anggia,
P. (2021). Deep learning in image classification using
residual network (resnet) variants for detection of col-
orectal cancer. Procedia Computer Science, 179:423–
431. 5th International Conference on Computer Sci-
ence and Computational Intelligence 2020.
Selvaraju, R. R., Cogswell, M., Das, A., Vedantam, R.,
Parikh, D., and Batra, D. (2019). Grad-cam: Visual
explanations from deep networks via gradient-based
localization. International Journal of Computer Vi-
sion, 128(2):336–359.
Sharma, H., Zerbe, N., Klempert, I., Hellwich, O., and Huf-
nagl, P. (2017). Deep convolutional neural networks
for automatic classification of gastric carcinoma using
whole slide images in digital histopathology. Com-
puterized Medical Imaging and Graphics, 61:2–13.
Selected papers from the 13th European Congress on
Digital Pathology.
Spanhol, F. A., Oliveira, L. S., Cavalin, P. R., Petitjean, C.,
and Heutte, L. (2017). Deep features for breast cancer
histopathological image classification. In 2017 IEEE
International Conference on Systems, Man, and Cy-
bernetics (SMC), pages 1868–1873.
Spanhol, F. A., Oliveira, L. S., Petitjean, C., and Heutte, L.
(2016a). Breast cancer histopathological image classi-
fication using convolutional neural networks. In 2016
International Joint Conference on Neural Networks
(IJCNN), pages 2560–2567.
Spanhol, F. A., Oliveira, L. S., Petitjean, C., and Heutte, L.
(2016b). A dataset for breast cancer histopathological
image classification. IEEE Transactions on Biomedi-
cal Engineering, 63(7):1455–1462.
Srinidhi, C. L., Ciga, O., and Martel, A. L. (2021). Deep
neural network models for computational histopathol-
ogy: A survey. Medical Image Analysis, 67:101813.
Tajbakhsh, N., Shin, J. Y., Gurudu, S. R., Hurst, R. T.,
Kendall, C. B., Gotway, M. B., and Liang, J. (2016).
Convolutional neural networks for medical image
analysis: Full training or fine tuning? IEEE Trans-
actions on Medical Imaging, 35(5):1299–1312.
Tellez, D., Litjens, G., B
´
andi, P., Bulten, W., Bokhorst, J.-
M., Ciompi, F., and van der Laak, J. (2019). Quanti-
fying the effects of data augmentation and stain color
normalization in convolutional neural networks for
computational pathology. Medical Image Analysis,
58:101544.
Thomas, A., Necchi, A., Muneer, A., Tobias-Machado, M.,
Tran, A. T. H., Van Rompuy, A.-S., Spiess, P. E., and
Albersen, M. (2021). Penile cancer. Nature Reviews
Disease Primers, 7(1):1–24.
Vieira, C., Feitoza, L., Pinho, J., Teixeira-J
´
unior, A., Lages,
J., Calixto, J., Coelho, R., Nogueira, L., Cunha, I.,
Soares, F., and Barros-Silva, G. (2020). Profile of pa-
tients with penile cancer in the region with the highest
worldwide incidence. Scientific Reports, 10.
Vill
´
an, A. (2019). Mastering OpenCV 4 with Python: A
practical guide covering topics from image process-
ing, augmented reality to deep learning with OpenCV
4 and Python 3.7. Packt Publishing.
Wei, J., Tafe, L., Linnik, Y., Vaickus, L., Tomita, N., and
Hassanpour, S. (2019). Pathologist-level classifica-
tion of histologic patterns on resected lung adenocar-
cinoma slides with deep neural networks. Scientific
Reports, 9.
Zhang, A., Lipton, Z. C., Li, M., and Smola, A. J.
(2021). Dive into deep learning. arXiv preprint
arXiv:2106.11342.
Zuiderveld, K. J. (1994). Contrast limited adaptive his-
togram equalization. In Graphics Gems.
Classification of Histopathological Images of Penile Cancer using DenseNet and Transfer Learning
983