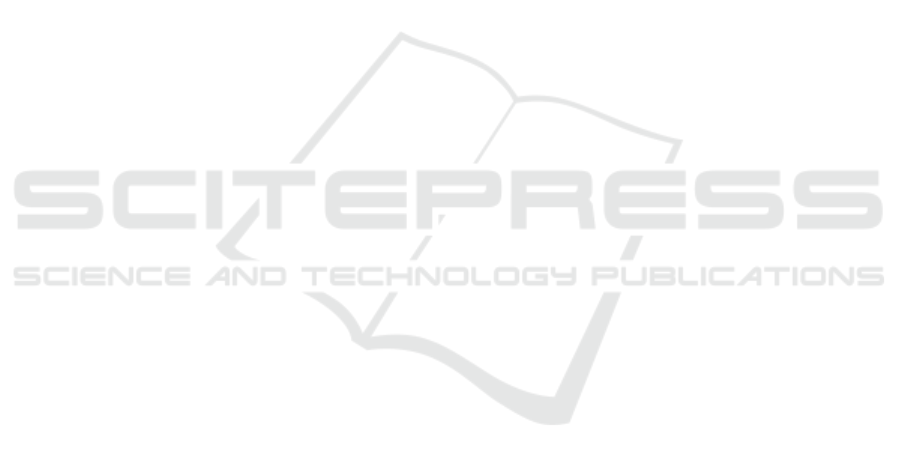
of participants still performed each standing and sit-
ting at about 1 to 2 seconds using the pushbutton, and
the overall duration statistics of these two activities
are approximately normally distributed. Such phe-
nomenons may involve a variety of topics such as
behavioral science and natural psychological rhythm,
which will not be expanded due to the different re-
search fields.
4 CONCLUSIONS
Relying on the rigorous acquisition protocol design
and execution, as well as the well-segmented data
corpus of the recently released multimodal wearable
sensor-based human activity dataset CSL-SHARE,
this paper analyzes the duration statistics and distri-
bution of 22 basic single motions of daily activities
and sports, providing research references for human
activity studies, such as segmentation, feature extrac-
tion, modeling, and recognition.
Through the big data statistical analysis of each
activity’s duration, we discovered that one single-
motion activity or one cycle in the activities of cycli-
cal motions has an average duration in the interval
from about 1 second to 2 seconds.
Furthermore, the duration distribution histograms
of each studied human daily activity or simple sports
activity evince interindividual similarities and natu-
rally obey a normal distribution. Even the two pos-
tures, standing and sitting, for which participants ar-
bitrarily decided each segment’s length, also conform
to this observation unpredictably.
As a classic case of applying activity duration
statistics in ML, (Liu and Schultz, 2019) used the pre-
vious dataset of CSL-SHARE with the same equip-
ment and investigated the transition from the offline
HAR modeling research to a real-time HAR system.
The activity duration was utilized as one of the ref-
erences to find the optimal balance between the on-
line decoding window length, the window overlap
length, and the recognition delay, endowing the real-
time demonstration with a satisfactory performance
and user experience.
We have noticed that different types of falling ac-
tivities also show interindividual similarity in terms
of duration, which is of great significance for human
activity research based on internal sensing and exter-
nal sensing, such as adopting HAR modeling for fall
detection and recognition (Xue and Liu, 2021). Dura-
tion analysis of typical falling activities will be a valu-
able topic to explore in the future, given appropriate
and adequate research materials.
REFERENCES
Amma, C., Krings, T., B
¨
oer, J., and Schultz, T. (2015).
Advancing muscle-computer interfaces with high-
density electromyography. In Proceedings of the 33rd
Annual ACM Conference on Human Factors in Com-
puting Systems, pages 929–938, New York, NY, USA.
ACM.
Ancans, A., Rozentals, A., Nesenbergs, K., and Greitans,
M. (2017). Inertial sensors and muscle electrical sig-
nals in human-computer interaction. In ICTA 2017
- 6th International Conference on Information and
Communication Technology and Accessibility, pages
1–6. IEEE.
Arous, M. A. B., Dunbar, M., Arfaoui, S., Mitiche,
A., Ouakrim, Y., Fuentes, A., Richardson, G., and
Mezghani, N. (2018). Knee kinematics feature selec-
tion for surgical and nonsurgical arthroplasty candi-
date characterization. In Proceedings of the 11th In-
ternational Joint Conference on Biomedical Engineer-
ing Systems and Technologies - Volume 3: BIOSIG-
NALS, pages 176–181.
Barandas, M., Folgado, D., Fernandes, L., Santos, S.,
Abreu, M., Bota, P., Liu, H., Schultz, T., and Gam-
boa, H. (2020). TSFEL: Time series feature extraction
library. SoftwareX, 11:100456.
Beddiar, D. R., Nini, B., Sabokrou, M., and Hadid,
A. (2020). Vision-based human activity recogni-
tion: A survey. Multimedia Tools and Applications,
79(41):30509–30555.
Ejupi, A., Gschwind, Y. J., Valenzuela, T., Lord, S. R., and
Delbaere, K. (2016). A kinect and inertial sensor-
based system for the self-assessment of fall risk: A
home-based study in older people. HCI 2016 - 18th
International Conference on Human-Computer Inter-
action, 31(3-4):261–293.
Gehrig, D. (2015). Automatic Recognition of Concurrent
and Coupled Human Motion Sequences. PhD thesis,
Karlsruher Institut f
¨
ur Technologie.
Georgi, M., Amma, C., and Schultz, T. (2015). Recognizing
hand and finger gestures with imu based motion and
emg based muscle activity sensing. In Proceedings of
the International Conference on Bio-inspired Systems
and Signal Processing - BIOSIGNALS, (BIOSTEC
2015), pages 99–108. INSTICC, SciTePress.
Hartmann, Y., Liu, H., Lahrberg, S., and Schultz, T. (2022).
Interpretable high-level features for human activity
recognition. In BIOSIGNALS 2022 — 15th Interna-
tional Conference on Bio-inspired Systems and Signal
Processing. INSTICC, SciTePress. forthcoming.
Hartmann, Y., Liu, H., and Schultz, T. (2020). Feature space
reduction for multimodal human activity recognition.
In Proceedings of the 13th International Joint Confer-
ence on Biomedical Engineering Systems and Tech-
nologies - Volume 4: BIOSIGNALS, pages 135–140.
INSTICC, SciTePress.
Hartmann, Y., Liu, H., and Schultz, T. (2021). Feature
space reduction for human activity recognition based
on multi-channel biosignals. In Proceedings of the
14th International Joint Conference on Biomedical
How Long Are Various Types of Daily Activities? Statistical Analysis of a Multimodal Wearable Sensor-based Human Activity Dataset
687