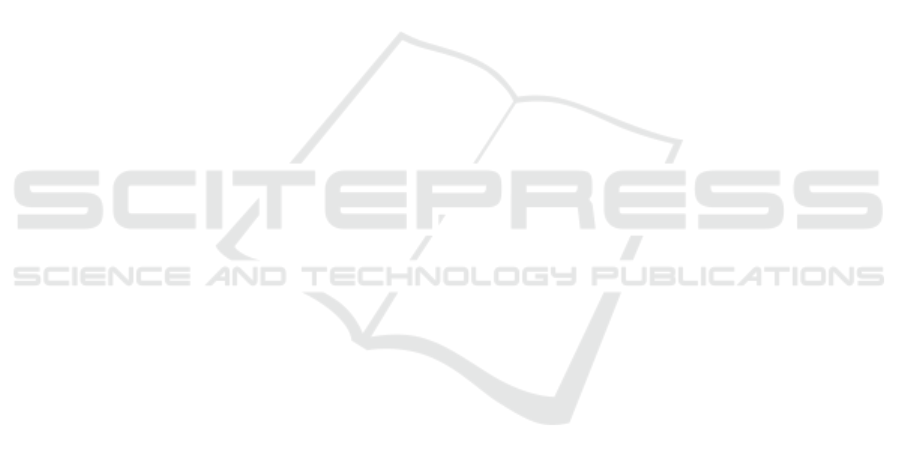
IEEE transactions on pattern analysis and machine
intelligence, 38(2):295–307.
Dong, C., Loy, C. C., and Tang, X. (2016). Accelerating
the super-resolution convolutional neural network. In
European conference on computer vision, pages 391–
407. Springer.
Farsiu, S., Elad, M., and Milanfar, P. (2004). Multiframe
demosaicing and super-resolution from undersampled
color images. In Computational Imaging II, volume
5299, pages 222–233. International Society for Optics
and Photonics.
Freeman, W. T., Jones, T. R., and Pasztor, E. C. (2002).
Example-based super-resolution. IEEE Computer
graphics and Applications, 22(2):56–65.
Girshick, R., Donahue, J., Darrell, T., and Malik, J. (2015).
Region-based convolutional networks for accurate ob-
ject detection and segmentation. IEEE transactions on
pattern analysis and machine intelligence, 38(1):142–
158.
Han, J., Yang, Y., Zhou, C., Xu, C., and Shi, B. (2021).
Evintsr-net: Event guided multiple latent frames re-
construction and super-resolution. In Proceedings of
the IEEE/CVF International Conference on Computer
Vision (ICCV), pages 4882–4891.
Hardie, R. C., Barnard, K. J., Bognar, J. G., Armstrong,
E. E., and Watson, E. A. (1998). High-resolution
image reconstruction from a sequence of rotated and
translated frames and its application to an infrared
imaging system. Optical Engineering, 37(1):247–
260.
Herrmann, C., Ruf, M., and Beyerer, J. (2018). Cnn-based
thermal infrared person detection by domain adapta-
tion. In Autonomous Systems: Sensors, Vehicles, Se-
curity, and the Internet of Everything, volume 10643,
page 1064308. International Society for Optics and
Photonics.
Huang, Y., Shao, L., and Frangi, A. F. (2019). Simultane-
ous super-resolution and cross-modality synthesis in
magnetic resonance imaging. In Deep Learning and
Convolutional Neural Networks for Medical Imaging
and Clinical Informatics, pages 437–457. Springer.
Irani, M. and Peleg, S. (1991). Improving resolution by im-
age registration. CVGIP: Graphical models and image
processing, 53(3):231–239.
Ji, H. and Ferm
¨
uller, C. (2008). Robust wavelet-based
super-resolution reconstruction: theory and algorithm.
IEEE Transactions on Pattern Analysis and Machine
Intelligence, 31(4):649–660.
Kim, J., Kwon Lee, J., and Mu Lee, K. (2016). Accurate
image super-resolution using very deep convolutional
networks. In Proceedings of the IEEE conference on
computer vision and pattern recognition, pages 1646–
1654.
Ledig, C., Theis, L., Husz
´
ar, F., Caballero, J., Cunningham,
A., Acosta, A., Aitken, A., Tejani, A., Totz, J., Wang,
Z., et al. (2017). Photo-realistic single image super-
resolution using a generative adversarial network. In
Proceedings of the IEEE conference on computer vi-
sion and pattern recognition, pages 4681–4690.
Lim, B., Son, S., Kim, H., Nah, S., and Mu Lee, K. (2017).
Enhanced deep residual networks for single image
super-resolution. In Proceedings of the IEEE con-
ference on computer vision and pattern recognition
workshops, pages 136–144.
Long, J., Peng, Y., Li, J., Zhang, L., and Xu, Y. (2021).
Hyperspectral image super-resolution via subspace-
based fast low tensor multi-rank regularization. In-
frared Physics & Technology, 116:103631.
Milanfar, P., Takeda, H., and Farslu, S. (2011). Kernel re-
gression for image processing and reconstruction. US
Patent 7,889,950.
Molini, A. B., Valsesia, D., Fracastoro, G., and Magli,
E. (2019). Deepsum: Deep neural network for
super-resolution of unregistered multitemporal im-
ages. IEEE Transactions on Geoscience and Remote
Sensing, 58(5):3644–3656.
Mudunuri, S. P. and Biswas, S. (2015). Low resolution face
recognition across variations in pose and illumination.
IEEE transactions on pattern analysis and machine
intelligence, 38(5):1034–1040.
Nguyen, N. L., Anger, J., Davy, A., Arias, P., and Facci-
olo, G. (2021). Self-supervised multi-image super-
resolution for push-frame satellite images. In Pro-
ceedings of the IEEE/CVF Conference on Computer
Vision and Pattern Recognition, pages 1121–1131.
Peleg, S., Keren, D., and Schweitzer, L. (1987). Improv-
ing image resolution using subpixel motion. Pattern
recognition letters, 5(3):223–226.
Pesavento, M., Volino, M., and Hilton, A. (2021).
Attention-based multi-reference learning for image
super-resolution. In Proceedings of the IEEE/CVF In-
ternational Conference on Computer Vision (ICCV),
pages 14697–14706.
Qi, H. and Diakides, N. A. (2003). Thermal infrared
imaging in early breast cancer detection-a survey of
recent research. In Proceedings of the 25th An-
nual International Conference of the IEEE Engineer-
ing in Medicine and Biology Society (IEEE Cat. No.
03CH37439), volume 2, pages 1109–1112. IEEE.
Rasti, P., Uiboupin, T., Escalera, S., and Anbarjafari, G.
(2016). Convolutional neural network super resolu-
tion for face recognition in surveillance monitoring.
In International conference on articulated motion and
deformable objects, pages 175–184. Springer.
Rivadeneira, R. E., Sappa, A. D., and Vintimilla, B. X.
(2020a). Thermal image super-resolution: A novel
architecture and dataset. In VISIGRAPP (4: VISAPP),
pages 111–119.
Rivadeneira, R. E., Sappa, A. D., Vintimilla, B. X., Guo,
L., Hou, J., Mehri, A., Behjati Ardakani, P., Patel, H.,
Chudasama, V., Prajapati, K., et al. (2020b). Thermal
image super-resolution challenge-pbvs 2020. In Pro-
ceedings of the IEEE/CVF Conference on Computer
Vision and Pattern Recognition Workshops, pages 96–
97.
Rivadeneira, R. E., Sappa, A. D., Vintimilla, B. X., Nathan,
S., Kansal, P., Mehri, A., Ardakani, P. B., Dalal, A.,
Akula, A., Sharma, D., et al. (2021). Thermal image
super-resolution challenge-pbvs 2021. In Proceedings
Multi-Image Super-Resolution for Thermal Images
641