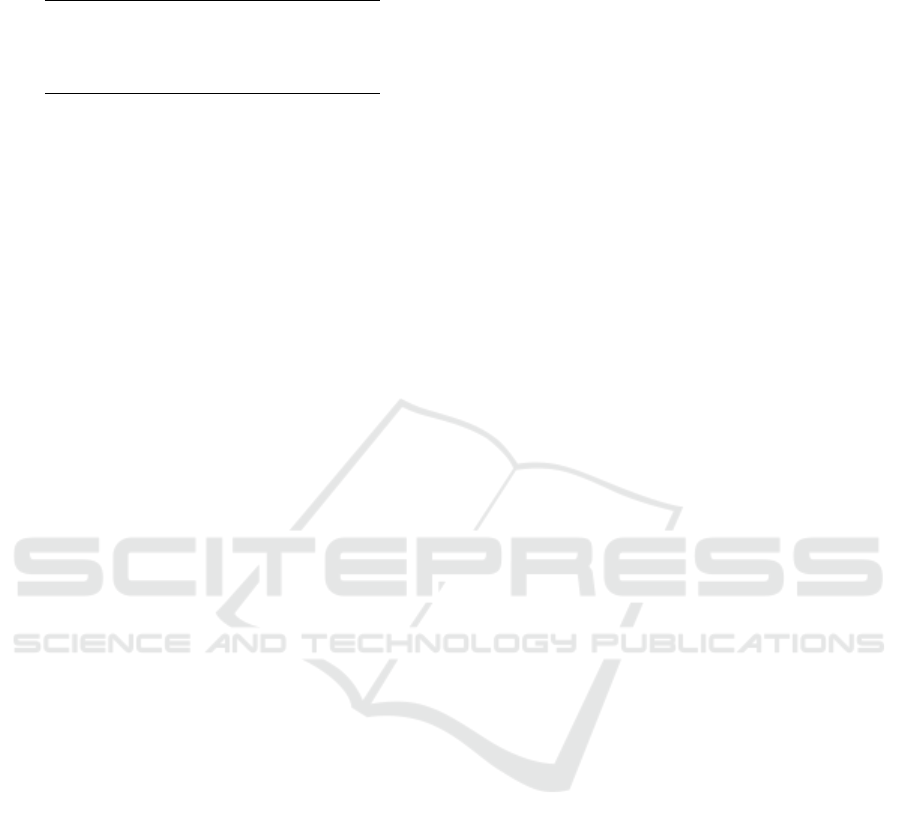
Table 1: Quantitative evaluation.
pix2pix MSGAN Ours
FID 98.23 88.84 92.27
NDB 11 11 9
JSD 0.0812 0.0559 0.0300
LPIPS 0.0621 0.3752 0.4444
be said that the lower these two indicators, the closer
the diversity is to the real data.
LPIPS
LPIPS measures the average distance between images
in the feature space. In this research, we evaluated the
diversity by measuring the average of LPIPS between
the generated images. It can be said that the higher
the LPIPS value, the more successful the generation
of diverse images.
Results
The table 1 shows the results of quantitative evalua-
tion of the existing methods and the proposed method.
From this table, we fined that the proposed method
shows the best score in metrics except FID, and we
find that the proposed method can generate diverse
images close to the ground truth distribution. Regard-
ing FID, although it has decreased in the proposed
method, the degradation is small, and we find that
the diversification was successful while maintaining
the quality of the generated images in the proposed
method.
5 CONCLUSION
In this research, we proposed a method for generat-
ing more diverse images in GAN. In particular, we
proposed a method that estimates the distribution of
training images in advance and uses it for learning to
generate diverse images. We demonstrated its effec-
tiveness by conducting comparative experiments with
the existing methods. The results show that the pro-
posed method can generate more diverse images effi-
ciently.
REFERENCES
Deng, J., Dong, W., Socher, R., Li, L.-J., Li, K., and Li, F.-
F. (2009). Imagenet: A large-scale hierarchical image
database. In CVPR, pages 248–255. IEEE Computer
Society.
Goodfellow, I., Pouget-Abadie, J., Mirza, M., Xu, B.,
Warde-Farley, D., Ozair, S., Courville, A., and Ben-
gio, Y. (2014). Generative adversarial nets. In
Advances in neural information processing systems,
pages 2672–2680.
Heusel, M., Ramsauer, H., Unterthiner, T., Nessler, B., and
Hochreiter, S. (2017). Gans trained by a two time-
scale update rule converge to a local nash equilibrium.
NIPS.
Isola, P., Zhu, J.-Y., Zhou, T., and Efros, A. A. (2017).
Image-to-image translation with conditional adversar-
ial networks. CVPR.
Mao, Q., Lee, H.-Y., Tseng, H.-Y., Ma, S., and Yang, M.-H.
(2019). Mode seeking generative adversarial networks
for diverse image synthesis. In IEEE Conference on
Computer Vision and Pattern Recognition.
Mirza, M. and Osindero, S. (2014). Conditional generative
adversarial nets. arXiv preprint arXiv:1411.1784.
Richardson, E. and Weiss, Y. (2018). On gans and gmms. In
Advances in Neural Information Processing Systems.
Szegedy, C., Wei Liu, Yangqing Jia, Sermanet, P., Reed, S.,
Anguelov, D., Erhan, D., Vanhoucke, V., and Rabi-
novich, A. (2015). Going deeper with convolutions. In
2015 IEEE Conference on Computer Vision and Pat-
tern Recognition (CVPR), pages 1–9.
Tan, M. and Le, Q. (2019). EfficientNet: Rethinking model
scaling for convolutional neural networks. In Chaud-
huri, K. and Salakhutdinov, R., editors, Proceedings of
the 36th International Conference on Machine Learn-
ing, volume 97 of Proceedings of Machine Learning
Research, pages 6105–6114. PMLR.
Yu, A. and Grauman, K. (2014). Fine-grained visual com-
parisons with local learning. In Computer Vision and
Pattern Recognition (CVPR).
Zhang, R., Isola, P., Efros, A. A., Shechtman, E., and Wang,
O. (2018). The unreasonable effectiveness of deep
features as a perceptual metric. In CVPR.
Zhu, J.-Y., Kr
¨
ahenb
¨
uhl, P., Shechtman, E., and Efros, A. A.
(2016). Generative visual manipulation on the natural
image manifold. In Proceedings of European Confer-
ence on Computer Vision (ECCV).
Zhu, J.-Y., Zhang, R., Pathak, D., Darrell, T., Efros, A. A.,
Wang, O., and Shechtman, E. (2017). Toward multi-
modal image-to-image translation. NIPS.
VISAPP 2022 - 17th International Conference on Computer Vision Theory and Applications
622