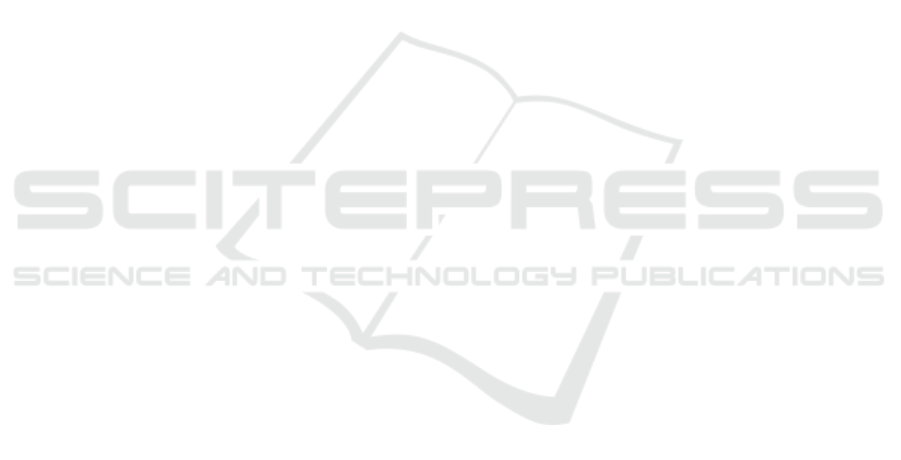
Helm, D. and Kampel, M. (2019). Shot boundary detec-
tion for automatic video analysis of historical films.
In Cristani, M., Prati, A., Lanz, O., Messelodi, S.,
and Sebe, N., editors, New Trends in Image Analysis
and Processing – ICIAP 2019, pages 137–147, Cham.
Springer International Publishing.
Helm, D., Kleber, F., and Kampel, M. (2022). HistShot: A
Shot Type Dataset based on Historical Documentation
during WWII. [will be published soon].
Helm, D., Pointner, B., and Kampel, M. (2020). Frame bor-
der detection for digitized historical footage. In Roth,
P. M., Steinbauer, G., Fraundorfer, F., Brandst
¨
otter,
M., and Perko, R., editors, Proceedings of the Joint
Austrian Computer Vision and Robotics Workshop
2020, pages 114–115, Graz. Verlag der Technischen
Universit
¨
at Graz.
Huang, Q., Xiong, Y., Rao, A., Wang, J., and Lin, D. (2020).
Movienet: A holistic dataset for movie understand-
ing. Lecture Notes in Computer Science (including
subseries Lecture Notes in Artificial Intelligence and
Lecture Notes in Bioinformatics), 12349 LNCS:709–
727.
Kahle, B. (1996). Internet archive. https://archive.org/. [On-
line; last accessed 2020/11/09].
Kipf, T. N. and Welling, M. (2016). Semi-supervised clas-
sification with graph convolutional networks. CoRR,
abs/1609.02907.
Krizhevsky, A. (2009). Learning multiple layers of features
from tiny images. pages 32–33.
Liang, Z., Guan, Y., and Rojas, J. (2020). Visual-Semantic
Graph Attention Network for Human-Object Interac-
tion Detection. 1.
Lin, T. Y., Maire, M., Belongie, S., Hays, J., Perona, P., Ra-
manan, D., Doll
´
ar, P., and Zitnick, C. L. (2014). Mi-
crosoft COCO: Common objects in context. Lecture
Notes in Computer Science (including subseries Lec-
ture Notes in Artificial Intelligence and Lecture Notes
in Bioinformatics), 8693 LNCS(PART 5):740–755.
Long, J., Yan, Z., and Chen, H. (2021). A Graph Neural
Network for superpixel image classification. Journal
of Physics: Conference Series, 1871(1).
Marino, K., Salakhutdinov, R., and Gupta, A. (2016). The
more you know: Using knowledge graphs for image
classification. CoRR, abs/1612.04844.
Misraa, A. K., Kale, A., Aggarwal, P., and Aminian, A.
(2020). Multi-modal retrieval using graph neural net-
works. CoRR, abs/2010.01666.
Nikolentzos, G., Thomas, M., Rivera, A. R., and Vazirgian-
nis, M. (2021). Image Classification Using Graph-
Based Representations and Graph Neural Networks,
volume 944. Springer International Publishing.
Rao, A., Wang, J., Xu, L., Jiang, X., Huang, Q., Zhou, B.,
and Lin, D. (2020). A unified framework for shot type
classification based on subject centric lens. In The
European Conference on Computer Vision (ECCV).
Russakovsky, O., Deng, J., Su, H., Krause, J., Satheesh, S.,
Ma, S., Huang, Z., Karpathy, A., Khosla, A., Bern-
stein, M., Berg, A. C., and Fei-Fei, L. (2015). Ima-
geNet Large Scale Visual Recognition Challenge. In-
ternational Journal of Computer Vision, 115(3):211–
252.
Sangeetha, V. and Prasad, K. J. (2006). Syntheses
of novel derivatives of 2-acetylfuro[2,3-a]carbazoles,
benzo[1,2-b]-1,4-thiazepino[2,3-a]carbazoles and 1-
acetyloxycarbazole-2- carbaldehydes. Indian Jour-
nal of Chemistry - Section B Organic and Medicinal
Chemistry, 45(8):1951–1954.
Savardi, M., Kov
´
acs, A. B., Signoroni, A., and Benini, S.
(2021). CineScale: A dataset of cinematic shot scale
in movies. Data in Brief, 36:107002.
Savardi, M., Signoroni, A., Migliorati, P., and Benini, S.
(2018). Shot scale analysis in movies by convolu-
tional neural networks. In 2018 25th IEEE Interna-
tional Conference on Image Processing (ICIP), pages
2620–2624.
Simonyan, K. and Zisserman, A. (2015). Very deep con-
volutional networks for large-scale image recognition.
3rd International Conference on Learning Represen-
tations, ICLR 2015 - Conference Track Proceedings,
pages 1–14.
Veli
ˇ
ckovi
´
c, P., Cucurull, G., Casanova, A., Romero, A., Li
`
o,
P., and Bengio, Y. (2018). Graph attention networks.
Vretos, N., Tsingalis, I., Nikolaidis, N., and Pitas, I. (2012).
Svm-based shot type classification of movie content.
Wu, Z., Pan, S., Chen, F., Long, G., Zhang, C., and Yu,
P. S. (2019). A comprehensive survey on graph neural
networks. CoRR, abs/1901.00596.
Yoon, S., Kang, W., Jeon, S., Lee, S., Han, C., Park,
J., and Kim, E. (2020). Image-to-image retrieval
by learning similarity between scene graphs. CoRR,
abs/2012.14700.
Zechner, I. (2015). Ludwig Boltzmann Institute for History
and Society: Ephemeral Films Project National So-
cialism in Austria. http://efilms.ushmm.org/. [Online;
last accessed 31.08.2020].
Zechner, I. and Loebenstein, M. (2016). Lud-
wig Boltzmann Institute for History and Soci-
ety and Austrian Film Museum: I-Media-Cities.
https://imediacities.hpc.cineca.it/app/catalog. [On-
line; last accessed 31.08.2020].
Zechner, I. and Loebenstein, M. (2019). Ludwig Boltz-
mann Institute for History and Society and Aus-
trian Film Museum. Project: Visual History of the
Holocaust: Rethinking Curation in the Digital Age.
https://www.vhh-project.eu/. [Online; last accessed
31.08.2020].
Zhang, S., Tong, H., Xu, J., and Maciejewski, R. (2019).
Graph convolutional networks: a comprehensive re-
view. Computational Social Networks, 6(1).
VISAPP 2022 - 17th International Conference on Computer Vision Theory and Applications
998