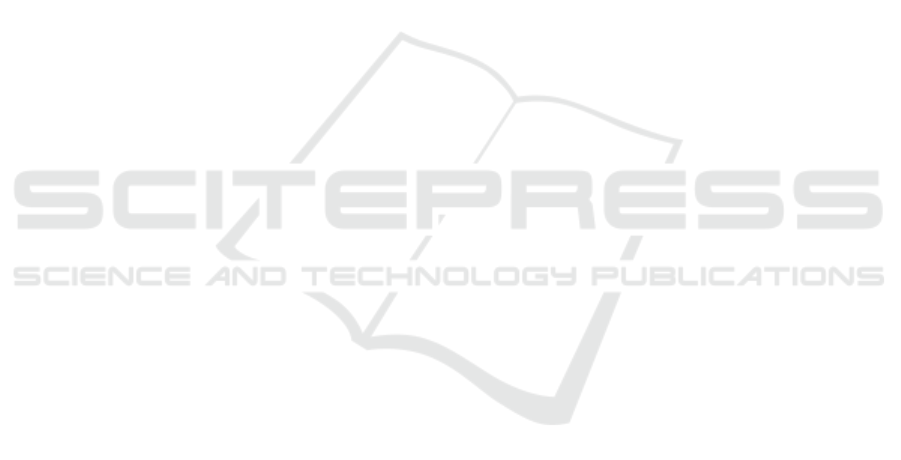
ACKNOWLEDGEMENTS
This work is funded by the Science and Technology
Development Fund STDF (Egypt); Project id: 42519
- “Automatic Video Surveillance System for Crowd
Scenes”.
REFERENCES
Berthelot, D., Carlini, N., Cubuk, E. D., Kurakin, A., Sohn,
K., Zhang, H., and Raffel, C. (2019a). Remixmatch:
Semi-supervised learning with distribution alignment
and augmentation anchoring.
Berthelot, D., Carlini, N., Goodfellow, I., Papernot, N.,
Oliver, A., and Raffel, C. (2019b). Mixmatch: A
holistic approach to semi-supervised learning.
Cai, R., Zhang, H., Liu, W., Gao, S., and Hao, Z.
(2021). Appearance-motion memory consistency net-
work for video anomaly detection. In Proceedings of
the AAAI Conference on Artificial Intelligence, vol-
ume 35, pages 938–946.
Carreira, J. and Zisserman, A. (2017). Quo vadis, action
recognition? a new model and the kinetics dataset.
In proceedings of the IEEE Conference on Computer
Vision and Pattern Recognition, pages 6299–6308.
Feichtenhofer, C. (2020). X3d: Expanding architectures
for efficient video recognition. In Proceedings of the
IEEE/CVF Conference on Computer Vision and Pat-
tern Recognition, pages 203–213.
Feichtenhofer, C., Fan, H., Malik, J., and He, K. (2019).
Slowfast networks for video recognition. In Proceed-
ings of the IEEE/CVF international conference on
computer vision, pages 6202–6211.
Feng, J.-C., Hong, F.-T., and Zheng, W.-S. (2021). Mist:
Multiple instance self-training framework for video
anomaly detection. In Proceedings of the IEEE/CVF
Conference on Computer Vision and Pattern Recogni-
tion, pages 14009–14018.
Georgescu, M.-I., Barbalau, A., Ionescu, R. T., Khan, F. S.,
Popescu, M., and Shah, M. (2021). Anomaly detec-
tion in video via self-supervised and multi-task learn-
ing. In Proceedings of the IEEE/CVF Conference
on Computer Vision and Pattern Recognition, pages
12742–12752.
Gong, D., Liu, L., Le, V., Saha, B., Mansour, M. R.,
Venkatesh, S., and Hengel, A. v. d. (2019). Memoriz-
ing normality to detect anomaly: Memory-augmented
deep autoencoder for unsupervised anomaly detec-
tion. In Proceedings of the IEEE/CVF International
Conference on Computer Vision, pages 1705–1714.
Hasan, M., Choi, J., Neumann, J., Roy-Chowdhury, A. K.,
and Davis, L. S. (2016). Learning temporal regularity
in video sequences. In Proceedings of the IEEE con-
ference on computer vision and pattern recognition,
pages 733–742.
Landi, F., Snoek, C. G., and Cucchiara, R. (2019). Anomaly
locality in video surveillance.
LaValle, S. M., Branicky, M. S., and Lindemann, S. R.
(2004). On the relationship between classical grid
search and probabilistic roadmaps. The International
Journal of Robotics Research, 23(7-8):673–692.
Lee, D.-H. et al. (2013). Pseudo-label: The simple and effi-
cient semi-supervised learning method for deep neural
networks. In Workshop on challenges in representa-
tion learning, ICML, volume 3, page 896.
Liu, K. and Ma, H. (2019). Exploring background-bias
for anomaly detection in surveillance videos. In Pro-
ceedings of the 27th ACM International Conference
on Multimedia, pages 1490–1499.
Luo, W., Liu, W., and Gao, S. (2017). A revisit of sparse
coding based anomaly detection in stacked rnn frame-
work. In Proceedings of the IEEE International Con-
ference on Computer Vision, pages 341–349.
Morais, R., Le, V., Tran, T., Saha, B., Mansour, M., and
Venkatesh, S. (2019). Learning regularity in skeleton
trajectories for anomaly detection in videos. In Pro-
ceedings of the IEEE/CVF Conference on Computer
Vision and Pattern Recognition, pages 11996–12004.
Park, H., Noh, J., and Ham, B. (2020). Learning memory-
guided normality for anomaly detection. In Proceed-
ings of the IEEE/CVF Conference on Computer Vision
and Pattern Recognition, pages 14372–14381.
Sohn, K., Berthelot, D., Li, C.-L., Zhang, Z., Carlini, N.,
Cubuk, E. D., Kurakin, A., Zhang, H., and Raffel, C.
(2020). Fixmatch: Simplifying semi-supervised learn-
ing with consistency and confidence.
Sultani, W., Chen, C., and Shah, M. (2018). Real-world
anomaly detection in surveillance videos. In Proceed-
ings of the IEEE conference on computer vision and
pattern recognition, pages 6479–6488.
Tran, D., Bourdev, L., Fergus, R., Torresani, L., and Paluri,
M. (2015). Learning spatiotemporal features with 3d
convolutional networks. In Proceedings of the IEEE
international conference on computer vision, pages
4489–4497.
Wang, S., Zeng, Y., Liu, Q., Zhu, C., Zhu, E., and Yin, J.
(2018a). Detecting abnormality without knowing nor-
mality: A two-stage approach for unsupervised video
abnormal event detection. In Proceedings of the 26th
ACM international conference on Multimedia, pages
636–644.
Wang, X., Girshick, R., Gupta, A., and He, K. (2018b).
Non-local neural networks. In Proceedings of the
IEEE conference on computer vision and pattern
recognition, pages 7794–7803.
Wang, Z., Zou, Y., and Zhang, Z. (2020). Cluster attention
contrast for video anomaly detection. In Proceedings
of the 28th ACM International Conference on Multi-
media, pages 2463–2471.
Zhang, J., Qing, L., and Miao, J. (2019). Temporal convo-
lutional network with complementary inner bag loss
for weakly supervised anomaly detection. In 2019
IEEE International Conference on Image Processing
(ICIP), pages 4030–4034. IEEE.
Zhu, Y. and Newsam, S. (2019). Motion-aware feature for
improved video anomaly detection.
VISAPP 2022 - 17th International Conference on Computer Vision Theory and Applications
878