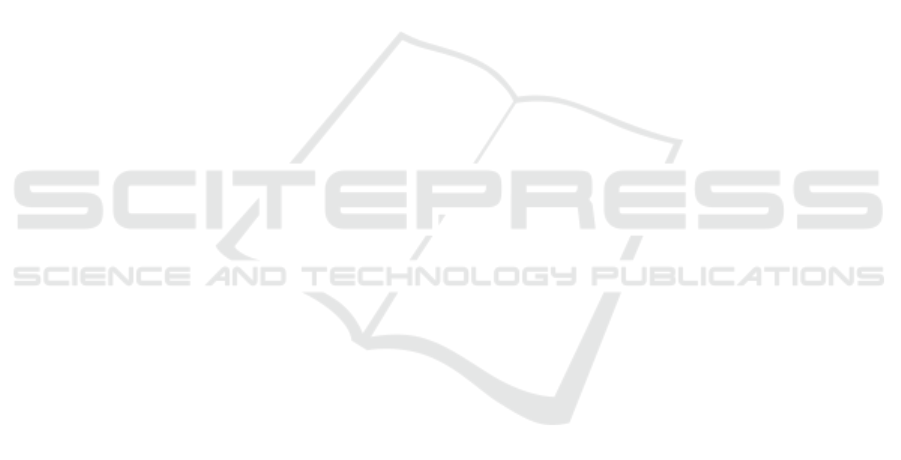
dirichlet allocation. Journal of machine Learning re-
search, 3(Jan):993–1022.
Caspi, R., Billington, R., Foerster, H., and et al.
(2016). Biocyc: Online resource for genome and
metabolic pathway analysis. The FASEB Journal, 30(1
Supplement):lb192–lb192.
Caspi, R., Billington, R., Keseler, I. M., Kothari, A., Krum-
menacker, M., Midford, P. E., Ong, W. K., Paley, S.,
Subhraveti, P., and Karp, P. D. (2019). The metacyc
database of metabolic pathways and enzymes-a 2019
update. Nucleic acids research.
Dale, J. M., Popescu, L., and Karp, P. D. (2010). Machine
learning methods for metabolic pathway prediction.
BMC bioinformatics, 11(1):1.
Fan, J., Liao, Y., and Liu, H. (2016). An overview of the
estimation of large covariance and precision matrices.
The Econometrics Journal, 19(1):C1–C32.
Hahn, A. S., Konwar, K. M., Louca, S., and et al. (2016).
The information science of microbial ecology. Cur-
rent opinion in microbiology, 31:209–216.
Hanson, N. W., Konwar, K. M., Hawley, A. K., and
et al.(2014). Metabolic pathways for the whole com-
munity. BMC genomics, 15(1):1.
Hassa, J., Maus, I., Off, S., P
¨
uhler, A., Scherer, P., Klocke,
M., and Schl
¨
uter, A. (2018). Metagenome, metatran-
scriptome, and metaproteome approaches unraveled
compositions and functional relationships of micro-
bial communities residing in biogas plants. Applied
microbiology and biotechnology, 102(12):5045–5063.
He, J., Hu, Z., Berg-Kirkpatrick, T., and et al. (2017). Effi-
cient correlated topic modeling with topic embedding.
In Proceedings of the 23rd ACM SIGKDD Interna-
tional Conference on Knowledge Discovery and Data
Mining, pages 225–233. ACM.
Hoffman, M. D., Blei, D. M., Wang, C., and Paisley,
J. W.(2013). Stochastic variational inference. Jour-
nal of Machine Learning Research, 14(1):1303–1347.
Jiao, D., Ye, Y., and Tang, H. (2013). Probabilistic infer-
ence of biochemical reactions in microbial communi-
ties from metagenomic sequences. PLoS Comput Biol,
9(3):e1002981.
Kanehisa, M., Furumichi, M., Tanabe, M., and et al.
(2017). Kegg: new perspectives on genomes, path-
ways, diseases and drugs. Nucleic Acids Research,
45(D1):D353–D361.
Karp, P. D., Latendresse, M., Paley, S. M., and et al. (2016).
Pathway tools version 19.0 update: software for path-
way/genome informatics and systems biology. Brief-
ings in bioinformatics, 17(5):877–890.
Khatri, P., Sirota, M., and Butte, A. J. (2012). Ten years
of pathway analysis: current approaches and out-
standing challenges. PLoS computational biology,
8(2):e1002375.
Lawson, C. E., Harcombe, W. R., Hatzenpichler, R., and
et al. (2019). Common principles and best practices
for engineering microbiomes. Nature Reviews Micro-
biology, pages 1–17.
Lin, T., Tian, W., Mei, Q., and Cheng, H.(2014). The
dual-sparse topic model: mining focused topics and
focused terms in short text. In Proceedings of the 23rd
international conference on World wide web, pages
539–550. ACM.
Loh, P.-R., Baym, M., and Berger, B. (2012). Compressive
genomics. Nature biotechnology, 30(7):627.
M. A. Basher, A. R. and Hallam, S. J. (2021). Relabeling
metabolic pathway data with groups to improve pre-
diction outcomes. BioRxiv.
M. A. Basher, A. R., McLaughlin, R. J., and Hallam, S. J.
(2020). Metabolic pathway inference using multi-
label classification with rich pathway features. PLOS
Computational Biology, 16(10):1–22.
M. A. Basher, A. R., McLaughlin, R. J., and Hallam, S. J.
(2021a). Metabolic pathway prediction using non-
negative matrix factorization with improved precision.
Journal of Computational Biology.
M. A. Basher, A. R., McLaughlin, R. J., and Hallam,
S. J. (2021b). Metabolic pathway prediction using
non-negative matrix factorization with improved pre-
cision. In Computational Advances in Bio and Med-
ical Sciences, pages 33–44, Cham. Springer Interna-
tional Publishing.
Mascher, M., Schreiber, M., Scholz, U., Graner, A.,
Reif, J. C., and Stein, N. (2019). Genebank ge-
nomics bridges the gap between the conservation of
crop diversity and plant breeding. Nature genetics,
51(7):1076–1081.
Qi, Q., Li, J., and Cheng, J. (2014). Reconstruction
of metabolic pathways by combining probabilistic
graphical model-based and knowledge-based meth-
ods. In BMC proceedings, volume 8, pages 1–10.
Springer.
Sczyrba, A., Hofmann, P., Belmann, P., and et al. (2017).
Critical assessment of metagenome interpretation—a
benchmark of metagenomics software. Nature meth-
ods, 14(11):1063.
Shafiei, M., Dunn, K. A., Chipman, H., Gu, H.,
and Bielawski, J. P. (2014). Biomenet: A
bayesian model for inference of metabolic divergence
among microbial communities. PLoS Comput Biol,
10(11):e1003918.
Stewart, F. J., Sharma, A. K., Bryant, J. A., and et al. (2011).
Community transcriptomics reveals universal patterns
of protein sequence conservation in natural microbial
communities. Genome biology, 12(3):R26.
Tabei, Y., Yamanishi, Y., and Kotera, M. (2016). Simul-
taneous prediction of enzyme orthologs from chem-
ical transformation patterns for de novo metabolic
pathway reconstruction. Bioinformatics, 32(12):i278–
i287.
Hallam Lab(2021a). leADS: https://github.com/ hallam-
lab/leADS.
Hallam Lab(2021b). reMap: https://github.com/ hallam-
lab/reMap.
Wang, W.-L., Xu, S.-Y., Ren, Z.-G., Tao, L., Jiang, J.-W.,
and Zheng, S.-S.(2015). Application of metagenomics
in the human gut microbiome. World journal of gas-
troenterology: WJG, 21(3):803.
Yamanishi, Y., Tabei, Y., and Kotera, M. (2015).
Metabolome-scale de novo pathway reconstruction
BIOINFORMATICS 2022 - 13th International Conference on Bioinformatics Models, Methods and Algorithms
60